Meta Structure Search for Link Weight Prediction in Heterogeneous Graphs.
IEEE International Conference on Acoustics, Speech, and Signal Processing(2024)
Abstract
Recently link weight prediction has attracted an increasing research interest due to its merits in quantifying the strength between nodes within a graph. Nonetheless, current link weight prediction methods focus solely on graph topology, disregarding node feature information embedded in graphs. In real-world applications, we often collect heterogeneous graph data where multiple types of nodes linked by multiple types of edges are available for analysis, and it is essential and challenging to quantify the proximity of different types of nodes. To solve this challenge, we present a new model for Heterogeneous Graph Link Weight Prediction (HLWP for short). In HLWP, message passing in heterogeneous graph neural networks is described as a meta structure, which can be effectively designed by Differentiable Neural Architecture Search (DARTS) algorithms. Thus, HLWP can enhance the message passing in heterogeneous graphs by DARTS. In addition, HLWP employs a perturbation-based algorithm to enhance stability and precision. Through empirical experiments conducted on three real-world datasets, we demonstrate that HLWP achieves accurate predictions of link weights. Our results highlight the superiority of HLWP over existing methods for link weight prediction and baseline GNN models in terms of accurately predicting link weights within heterogeneous graphs.
MoreTranslated text
Key words
link weight prediction,heterogeneous graph,meta structure,graph neural networks
AI Read Science
Must-Reading Tree
Example
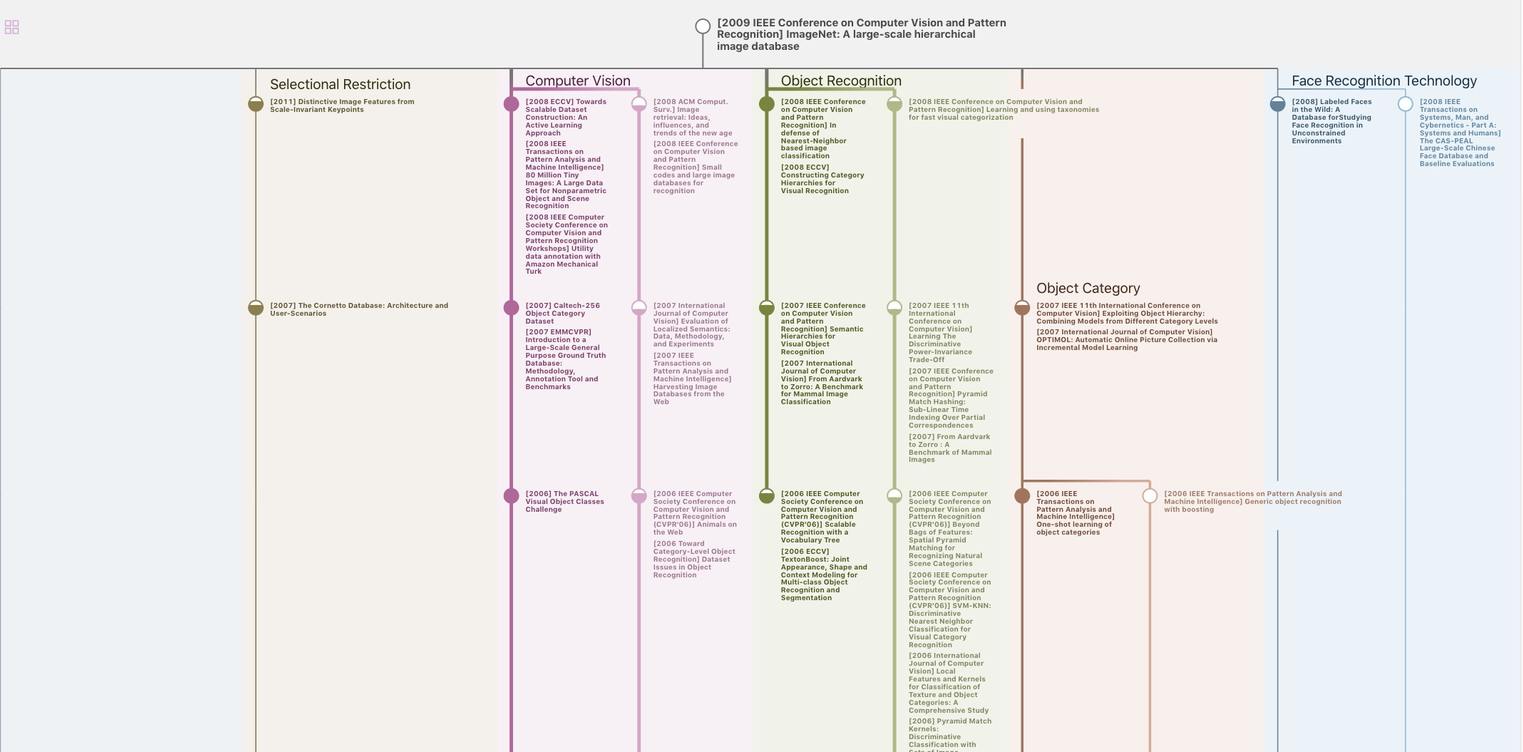
Generate MRT to find the research sequence of this paper
Chat Paper
Summary is being generated by the instructions you defined