Abrupt Change Fault, Slow Change Fault and Intermittent Fault Data Oversampling Technique for Health Evaluation of Transmission Equipment in Electric Power Communication Network
Advanced Fiber Laser Conference (AFL2023)(2024)
摘要
With the continuous expansion of electric power communication network scale, the increasingly complex topology structure, the rapid growth of access terminals and production business, electric power communication network faces challenges in intelligent operation and maintenance. Training a deep neural network fault prediction model and then constructing an electric power communication network transmission equipment health evaluation model can quantify the network health status and improve the level of network intelligent operation and maintenance. However, after the original data collected from the electric power communication network is preprocessed, the number of alarm data is far less than the number of non-alarm data, and the distribution of data characteristics is unbalanced, which will lead to the deterioration of the prediction performance of the fault prediction model and the accuracy of the transmission equipment health analysis results. This paper presents an abrupt change fault, slow change fault and intermittent fault data oversampling technique (ASIDOTE). According to the distribution of alarm data, which is divided into abrupt change fault, slow change fault and intermittent fault data, different data augmentation methods are applied to generate new alarm data while retaining the characteristic distribution of alarm data, and the number of alarm data and non-alarm data are balanced to reduce the imbalance degree. The proposed oversampling method is used to perform data augmentation on real data from the electric power communication network to train a fault prediction model. Experiment results demonstrate that the accuracy of the fault prediction model trained by the proposed oversampling algorithm on the test data set reaches 97.92%, which is 5.21% higher than that without data augmentation. The proposed ASMOTE algorithm reduces the deviation of fault prediction model due to uneven feature distribution, and improves the accuracy of fault prediction model applied to the health evaluation of transmission equipment in electric power communication network.
更多查看译文
AI 理解论文
溯源树
样例
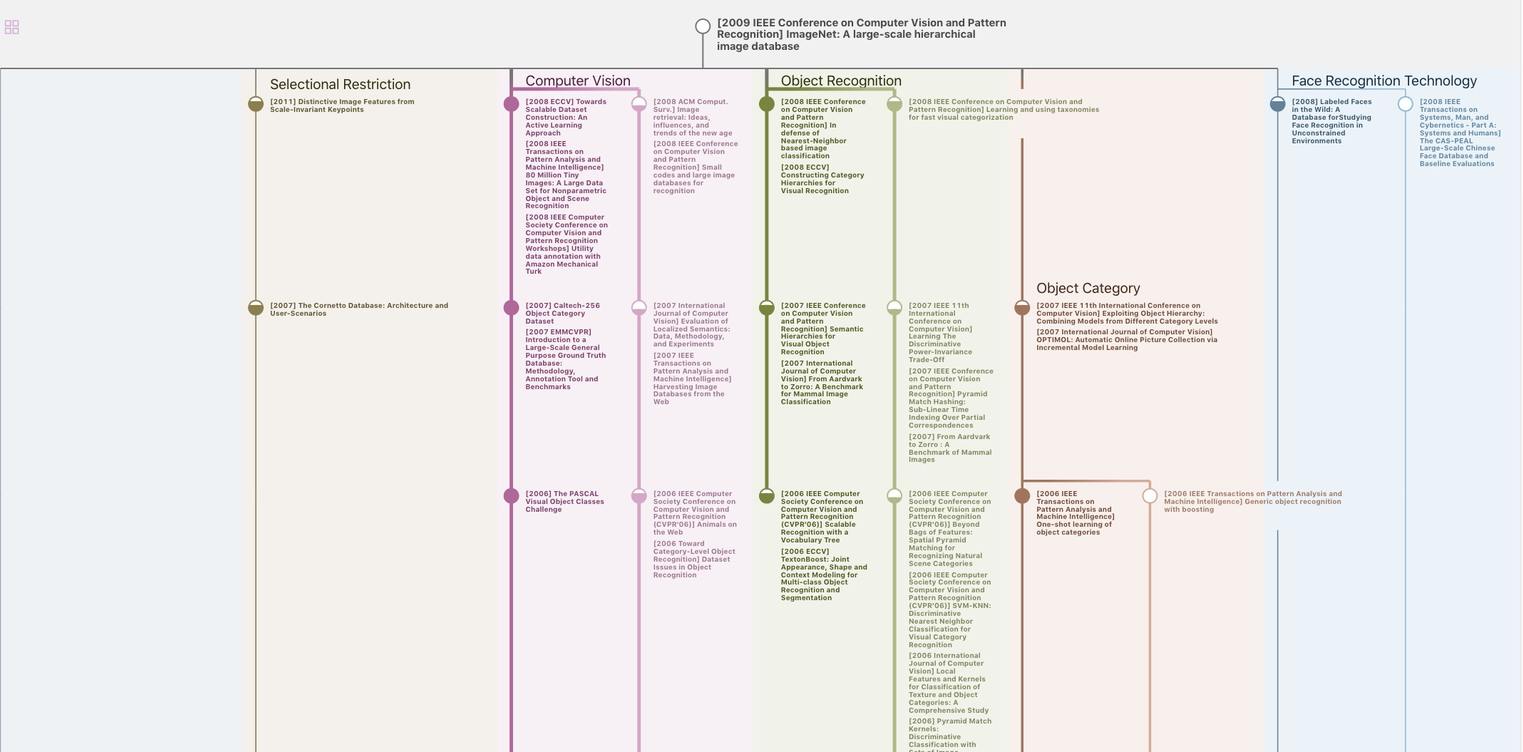
生成溯源树,研究论文发展脉络
Chat Paper
正在生成论文摘要