Self-attention CNN for Retinal Layer Segmentation in OCT.
BIOMEDICAL OPTICS EXPRESS(2024)
关键词
OCT Technology,Spectral-Domain OCT,Optical Coherence Tomography,Image Segmentation,Tissue Optical Clearing
AI 理解论文
溯源树
样例
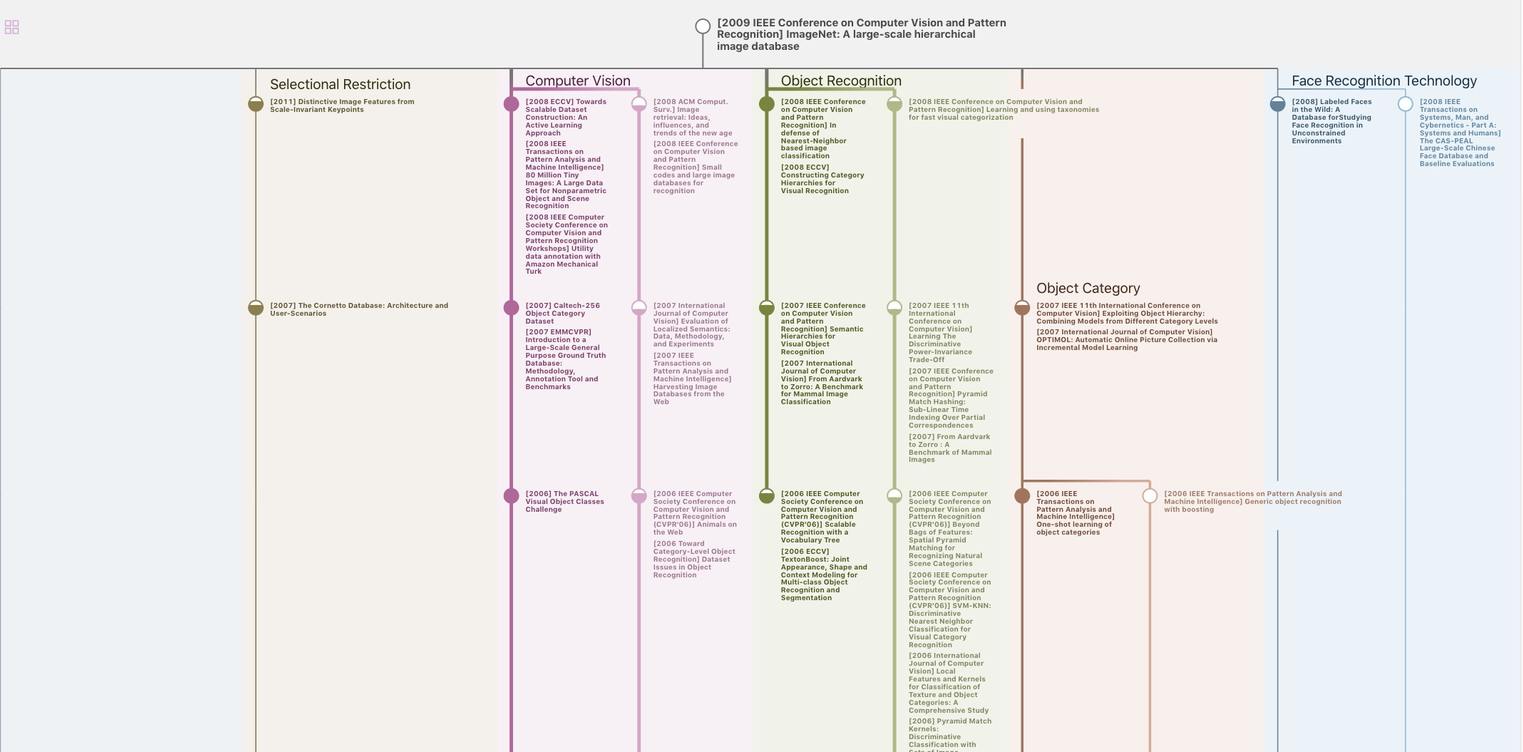
生成溯源树,研究论文发展脉络
Chat Paper
正在生成论文摘要