Continuous monitoring of grassland AGB during the growing season through integrated remote sensing: a hybrid inversion framework
INTERNATIONAL JOURNAL OF DIGITAL EARTH(2024)
摘要
Inverting grassland above-ground biomass (AGB) presents a significant challenge due to difficulties in characterizing leaf physiological states and obtaining accurate ground-truth data. This study introduces an innovative hybrid model for AGB inversion based on the AGB = leaf mass per area (LMA) * leaf area index (LAI) paradigmn in the Ewenki Banner region of Inner Mongolia. The model integrates the PROSAIL radiative transfer model, machine learning regression, LEnKF data assimilation theory, multisource remote sensing, and meteorological data, following a four-step approach. Firstly, we establish LAI and LMA inversion models by combining the PROSAIL model with machine learning techniques. Secondly, data assimilation fuses the PROSAIL-derived LAI with MODIS-LAI. In the third phase, a Random Forest predictive model is developed for LMA estimation. Lastly, the accuracy of the hybrid model is assessed using empirical data. Precision evaluation with ground-truth samples demonstrates that the assimilated LAI and RF-predicted LMA yield the lowest prediction error for grassland AGB (RMSE = 0.0033 g/cm2; MAE = 0.0028 g/cm2). This model framework addresses the challenge of limited prior knowledge in the PROSAIL-AGB prediction model, thereby enhancing the prediction accuracy while maintaining its key advantages: providing continuous observations at high spatiotemporal resolutions without relying on measured sample data.
更多查看译文
关键词
Grassland AGB,PROSAIL,machine learning,data assimilation,Inner Mongolia,hybrid inversion
AI 理解论文
溯源树
样例
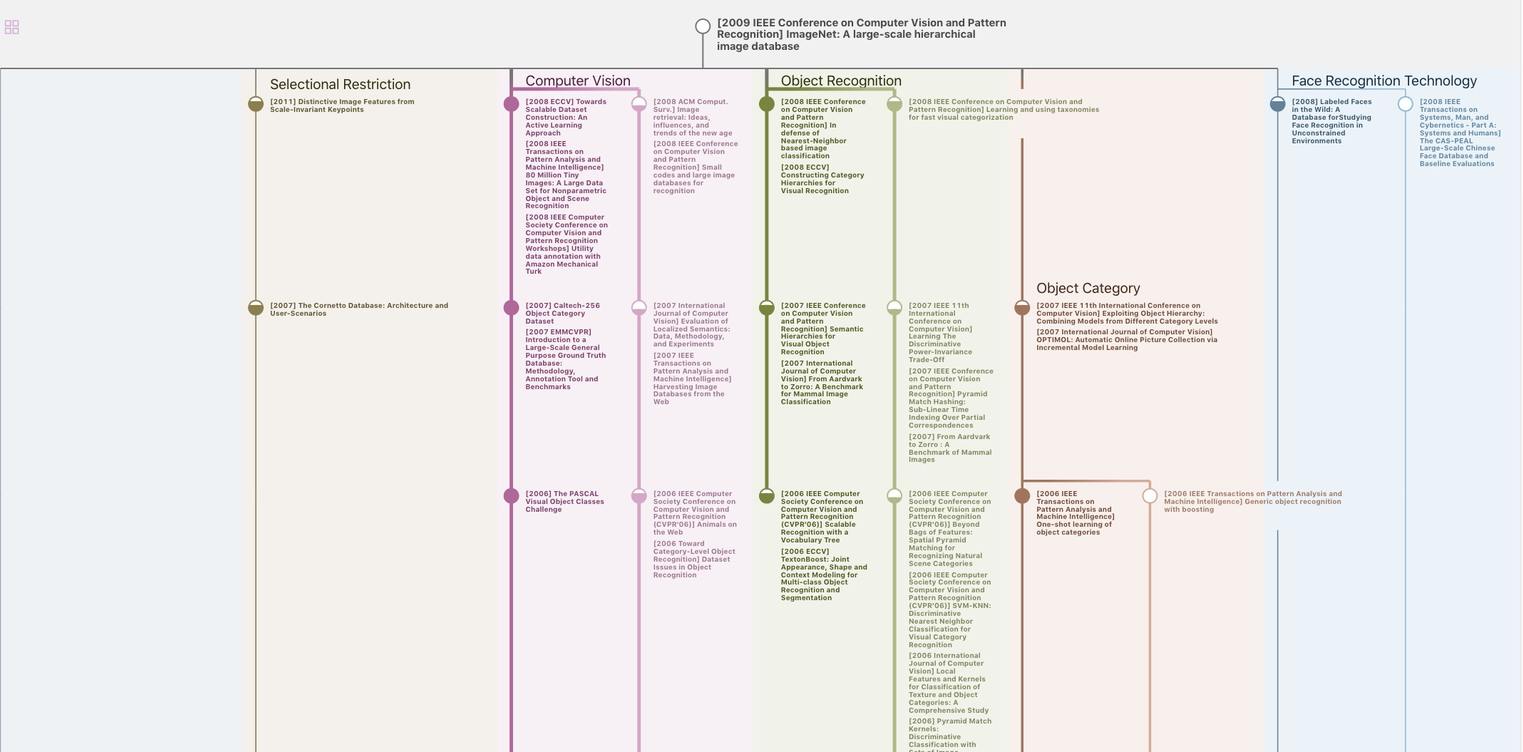
生成溯源树,研究论文发展脉络
Chat Paper
正在生成论文摘要