Quantization Effects on Neural Networks Perception: How Would Quantization Change the Perceptual Field of Vision Models?
2024 IEEE Thirteenth International Conference on Image Processing Theory, Tools and Applications (IPTA)(2024)
关键词
Neural Network Quantization,Class Activation Maps (CAM),Model Interpretability,Salient objects detection
AI 理解论文
溯源树
样例
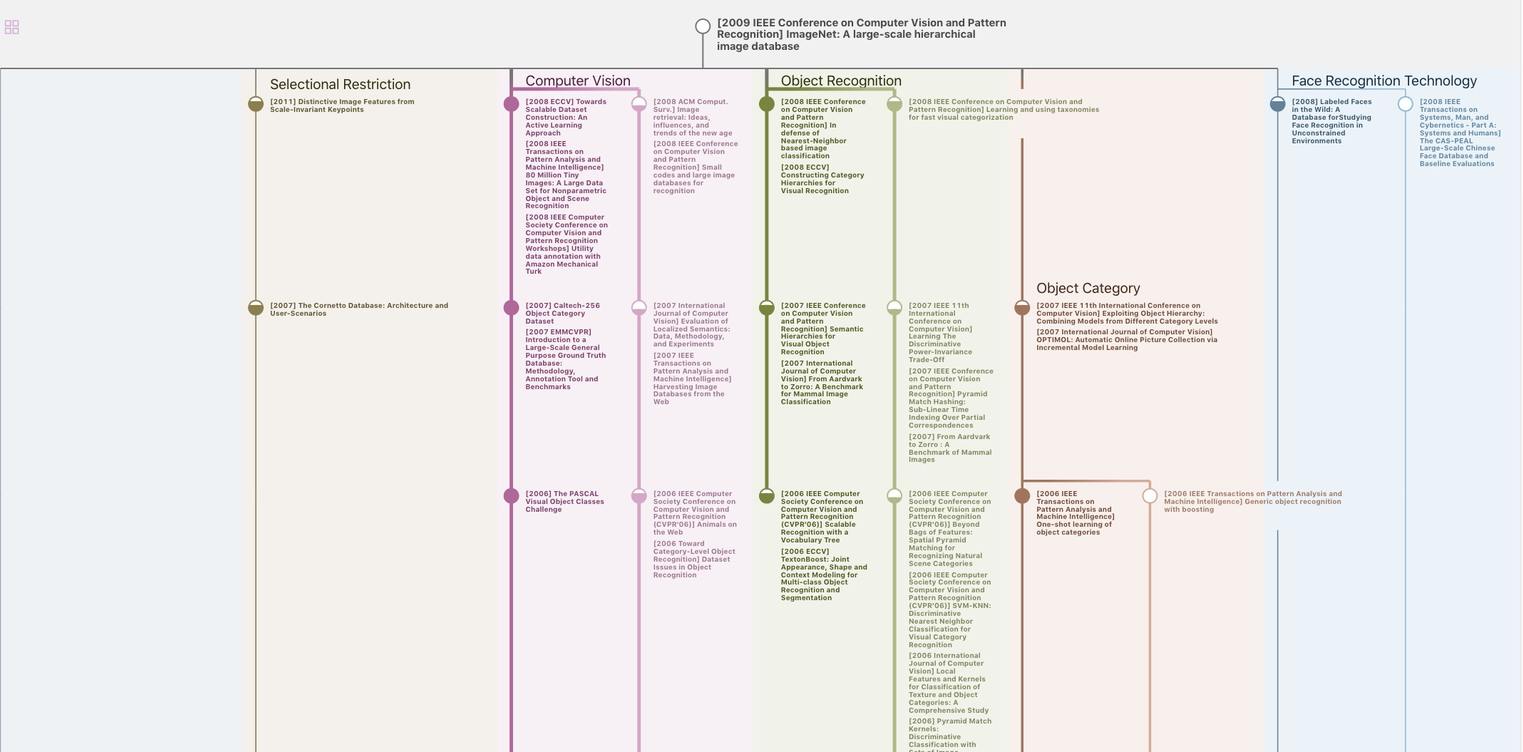
生成溯源树,研究论文发展脉络
Chat Paper
正在生成论文摘要