Accessible Machine Learning and Deep Learning Models Predict Response and Survival in Early Stage Hormone Receptor-Positive/HER2-Negative Breast Cancer Receiving Neoadjuvant Chemotherapy
crossref(2024)
摘要
Abstract Hormone receptor-positive/HER2 negative breast cancer (BC) is the most common subtype of BC and typically occurs as an early, operable disease. In patients receiving neoadjuvant chemotherapy (NACT), pathological complete response (pCR) is rare and multiple efforts have been made to predict disease recurrence and survival. We developed a framework to predict pCR, disease-free survival (DFS) and overall survival (OS) using clinicopathological characteristics widely available at diagnosis and after surgery. The machine learning (ML) model trained to predict pCR (n = 463) was evaluated in an internal validation cohort (n = 109) and validated in an external validation cohort (n = 171), achieving an area under the curve (AUC) of respectively 0.86 and 0.81. The models trained to predict DFS and OS were evaluated in the internal validation cohort, achieving a concordance index of 0.70 and 0.69. Our results emphasize the value of including accessible ML algorithms in clinical practice and provide a framework for the development of risk-adapted clinical trials based on ML models.
更多查看译文
AI 理解论文
溯源树
样例
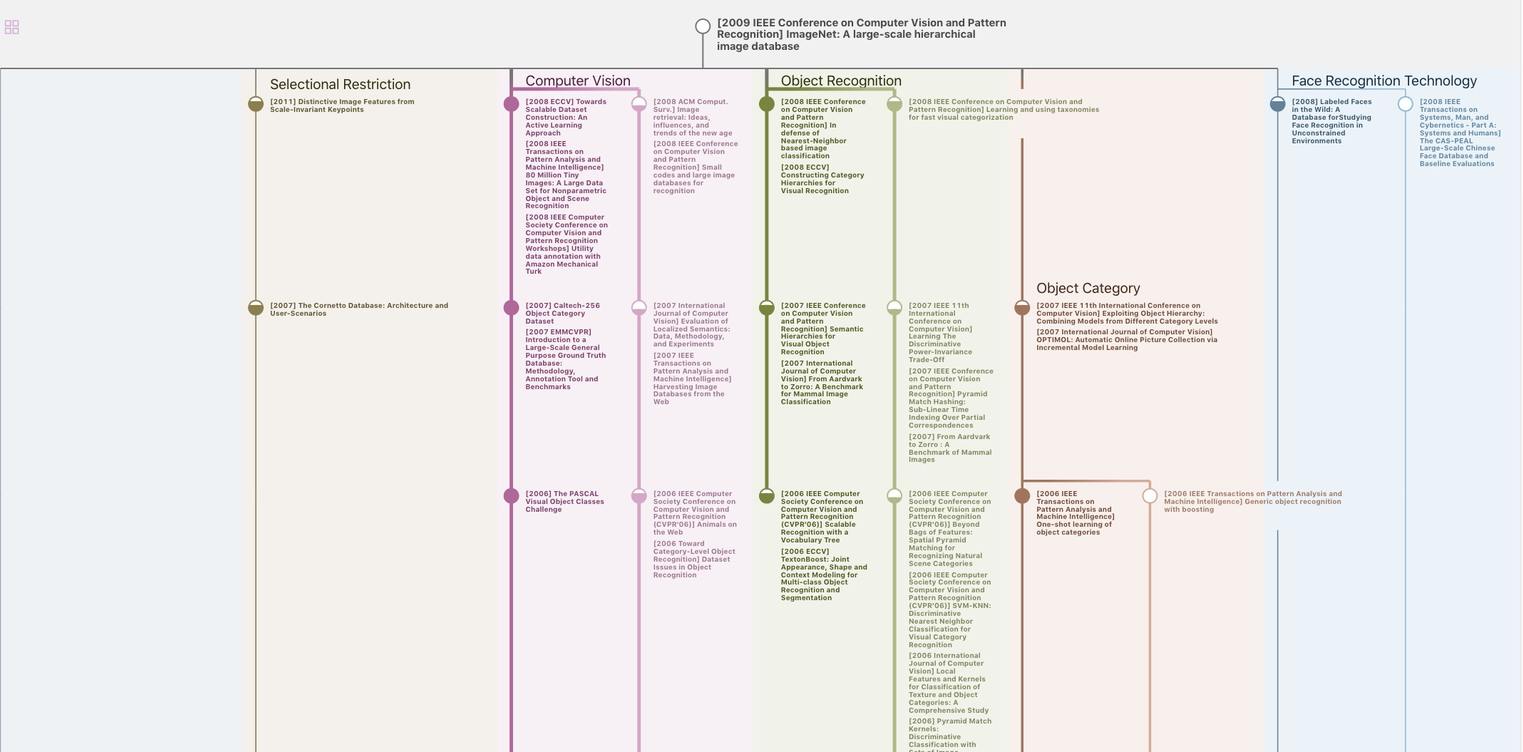
生成溯源树,研究论文发展脉络
Chat Paper
正在生成论文摘要