Leveraging Chat-Based Large Vision Language Models for Multimodal Out-Of-Context Detection
Advanced Information Networking and Applications Lecture Notes on Data Engineering and Communications Technologies(2024)
摘要
Out-of-context (OOC) detection is a challenging task involving identifying
images and texts that are irrelevant to the context in which they are
presented. Large vision-language models (LVLMs) are effective at various tasks,
including image classification and text generation. However, the extent of
their proficiency in multimodal OOC detection tasks is unclear. In this paper,
we investigate the ability of LVLMs to detect multimodal OOC and show that
these models cannot achieve high accuracy on OOC detection tasks without
fine-tuning. However, we demonstrate that fine-tuning LVLMs on multimodal OOC
datasets can further improve their OOC detection accuracy. To evaluate the
performance of LVLMs on OOC detection tasks, we fine-tune MiniGPT-4 on the
NewsCLIPpings dataset, a large dataset of multimodal OOC. Our results show that
fine-tuning MiniGPT-4 on the NewsCLIPpings dataset significantly improves the
OOC detection accuracy in this dataset. This suggests that fine-tuning can
significantly improve the performance of LVLMs on OOC detection tasks.
更多查看译文
AI 理解论文
溯源树
样例
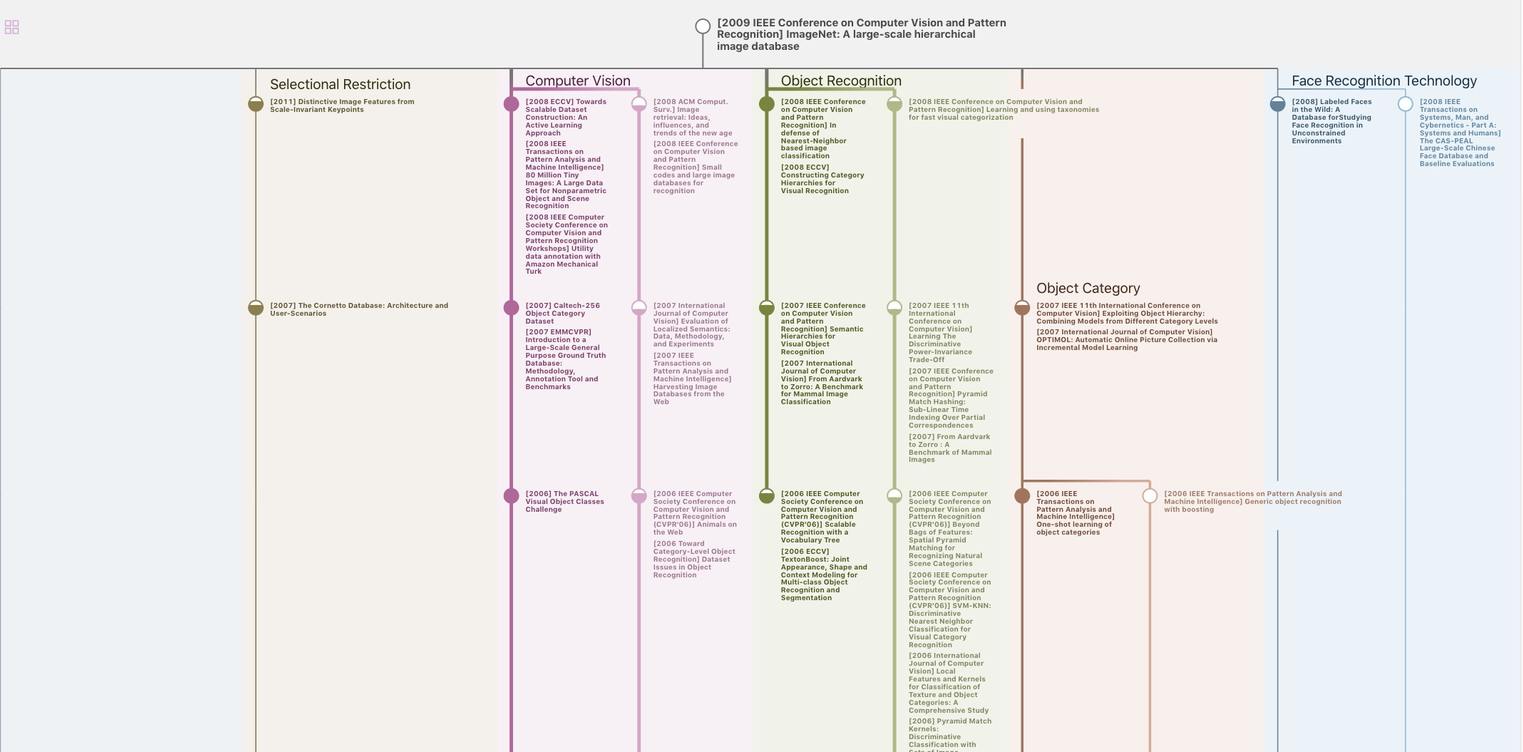
生成溯源树,研究论文发展脉络
Chat Paper
正在生成论文摘要