Learning to Jointly Transform and Rank Difficult Queries
ADVANCES IN INFORMATION RETRIEVAL, ECIR 2024, PT IV(2024)
摘要
Recent empirical studies have shown that while neural rankers exhibit increasingly higher retrieval effectiveness on tasks such as ad hoc retrieval, these improved performances are not experienced uniformly across the range of all queries. There are typically a large subset of queries that are not satisfied by neural rankers. These queries are often referred to as difficult queries. Given the fact that neural rankers operate based on the similarity between the embedding representations of queries and their relevant documents, the poor performance of difficult queries can be due to the sub-optimal representations learnt for difficult queries. As such, the objective of our work in this paper is to learn to rank documents and also transform query representations in tandem such that the representation of queries are transformed into one that shows higher resemblance to their relevant document. This way, our method will provide the opportunity to satisfy a large number of difficult queries that would otherwise not be addressed. In order to learn to jointly rank documents and transform queries, we propose to integrate two forms of triplet loss functions into neural rankers such that they ensure that each query is moved along the embedding space, through the transformation of its embedding representation, in order to be placed close to its relevant document(s). We perform experiments based on the MS MARCO passage ranking task and show that our proposed method has been able to show noticeable performance improvement for queries that were extremely difficult for existing neural rankers. On average, our approach has been able to satisfy 277 queries with an MRR@10 of 0.21 for queries that had a reciprocal rank of zero on the initial neural ranker.
更多查看译文
AI 理解论文
溯源树
样例
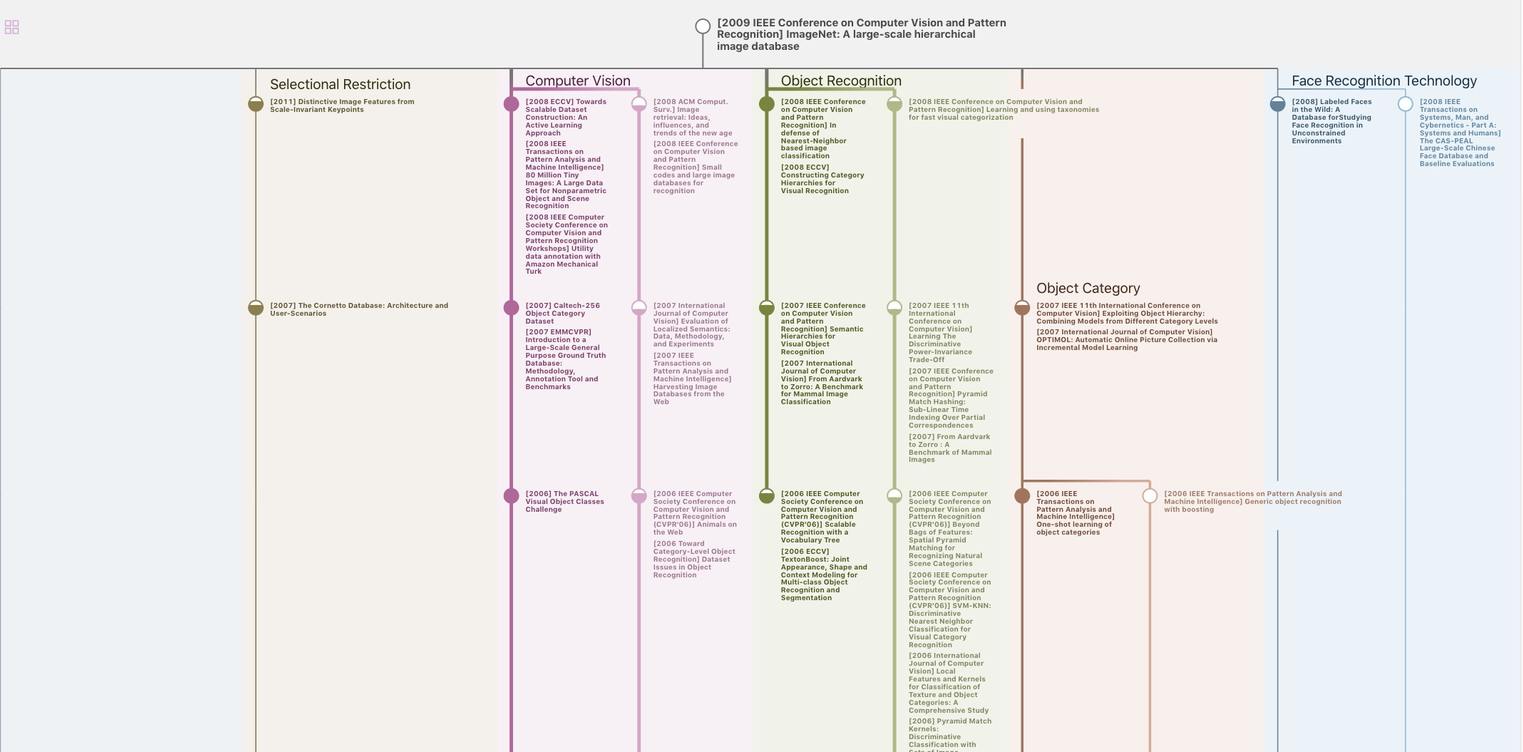
生成溯源树,研究论文发展脉络
Chat Paper
正在生成论文摘要