Properties and Practicability of Convergence-Guaranteed Optimization Methods Derived from Weak Discrete Gradients
Numerical Algorithms(2024)
摘要
The ordinary differential equation (ODE) models of optimization methods allow for concise proofs of convergence rates through discussions based on Lyapunov functions. The weak discrete gradient (wDG) framework discretizes ODEs while preserving the properties of convergence, serving as a foundation for deriving optimization methods. Although various optimization methods have been derived through wDG, their properties and practical applicability remain underexplored. Hence, this study elucidates these aspects through numerical experiments. Particularly, although wDG yields several implicit methods, we highlight the potential utility of these methods in scenarios where the objective function incorporates a regularization term.
更多查看译文
关键词
Convex optimization,Proximal gradient method,Numerical analysis,Discrete gradient
AI 理解论文
溯源树
样例
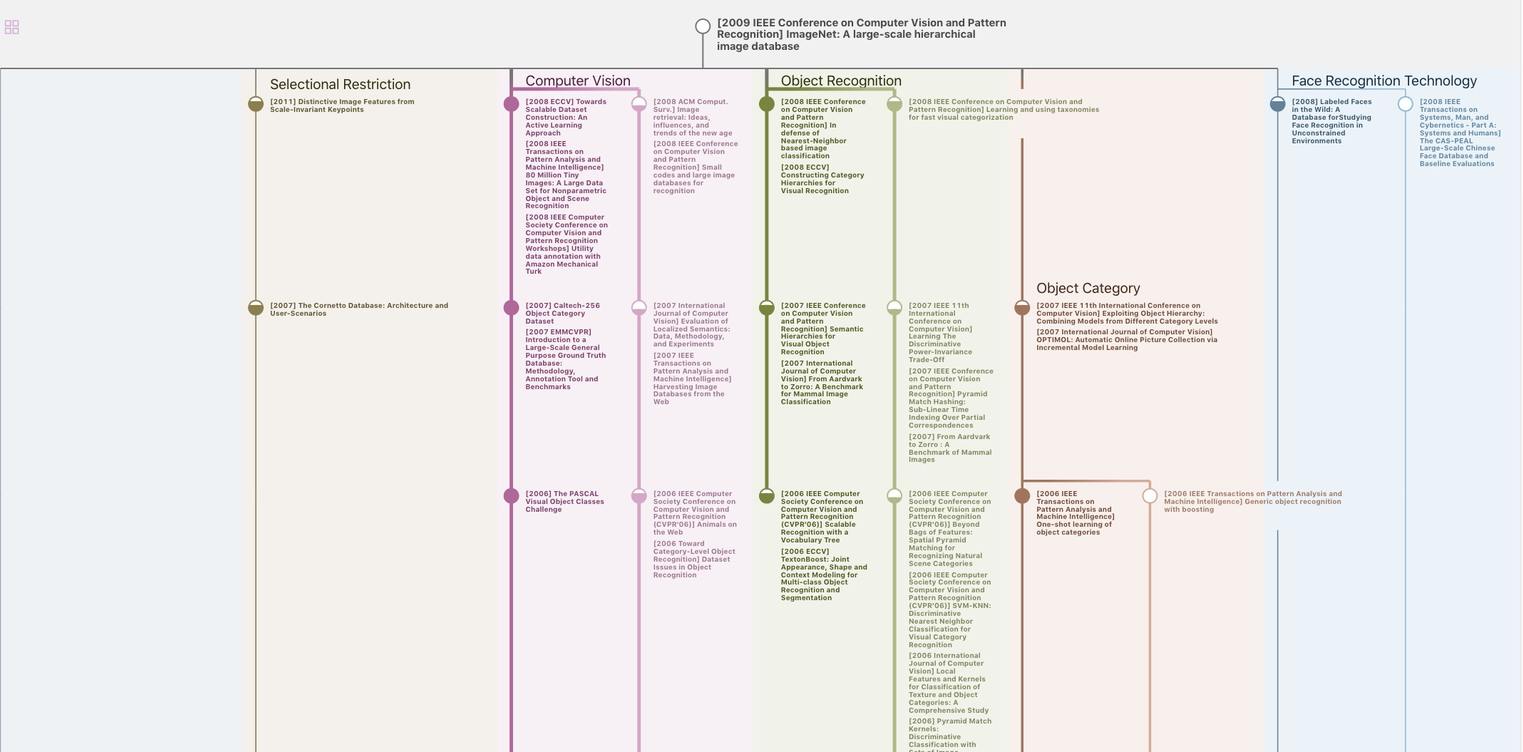
生成溯源树,研究论文发展脉络
Chat Paper
正在生成论文摘要