Species Gain and Loss Per Degree Celsius
OIKOS(2024)
Key words
Australian plants,beta diversity,climate change,climate gradients,temperature,turnover
AI Read Science
Must-Reading Tree
Example
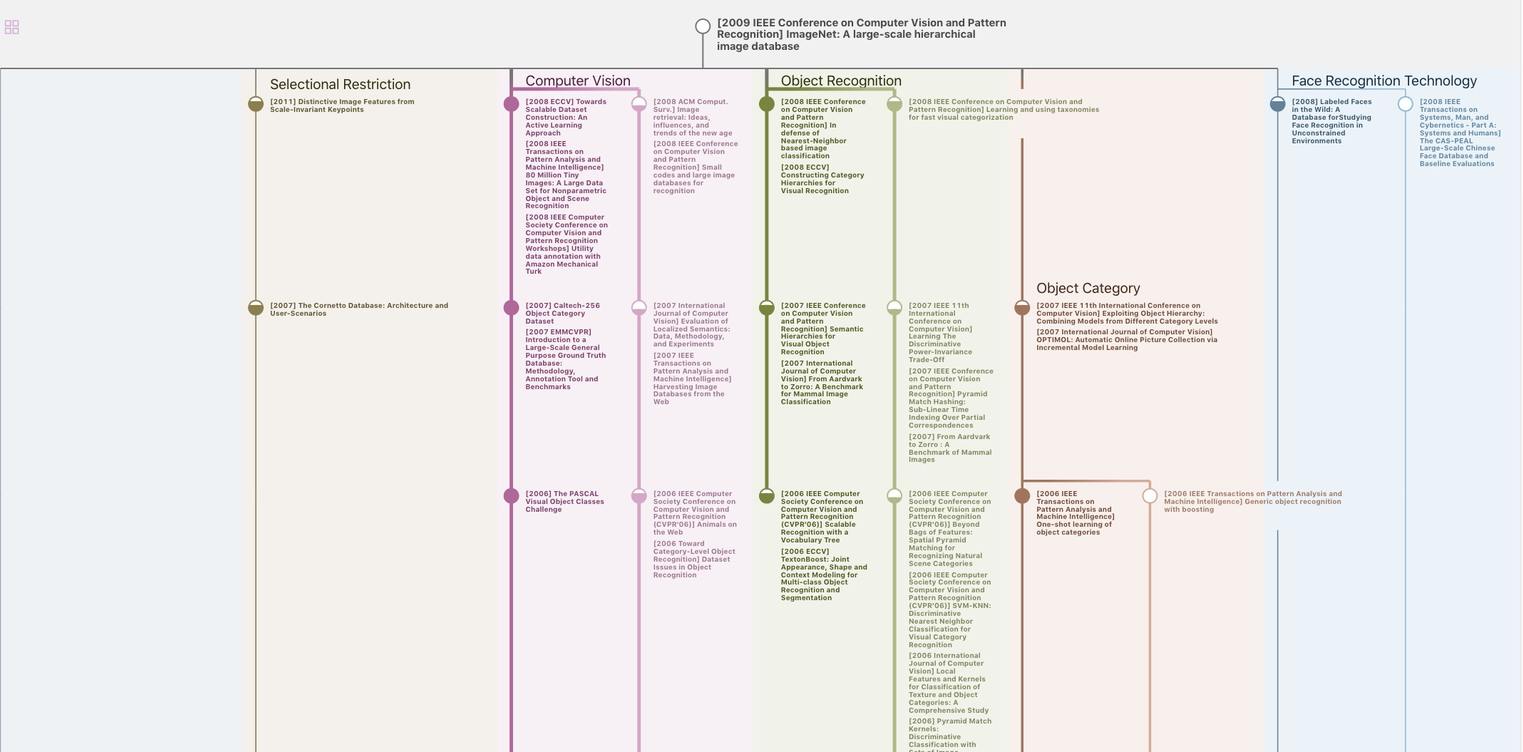
Generate MRT to find the research sequence of this paper
Chat Paper
Summary is being generated by the instructions you defined