20.5 C-Transformer: A 2.6-18.1Μj/token Homogeneous DNN-Transformer/Spiking-Transformer Processor with Big-Little Network and Implicit Weight Generation for Large Language Models
2024 IEEE International Solid-State Circuits Conference (ISSCC)(2024)
摘要
Recently, transformer-based large language models (LLMs), shown in Fig. 20.5.1, are widely used, and even on-device LLM systems with real-time responses are anticipated [1]. Many transformer processors [2–4] enhance energy efficiency by increasing hardware utilization and reducing power consumption, but their system power consumption and response time are still not suitable for mobile devices. Since LLMs, such as GPT-2, have many parameters (400-700M), their External Memory Access (EMA) consumes 68% of the total power. Pruning can increase sparsity to alleviate the EMA bottleneck, but can be applied only to a simple task (language modeling) to predict the next word [5]. For high-level tasks such as translation, question answering, and summarization, it is impossible to achieve high sparsity (<~40%) [6].
更多查看译文
关键词
Language Model,Large Language Models,Energy Consumption,Power Consumption,Sparsity,Input Values,Random Values,Question Answering,Input Channels,Spiking Neural Networks,Perplexity,Least Significant Bit,Real-time Response,Increase Energy Efficiency,Datapath,Position Embedding,Output Spike,Area Overhead
AI 理解论文
溯源树
样例
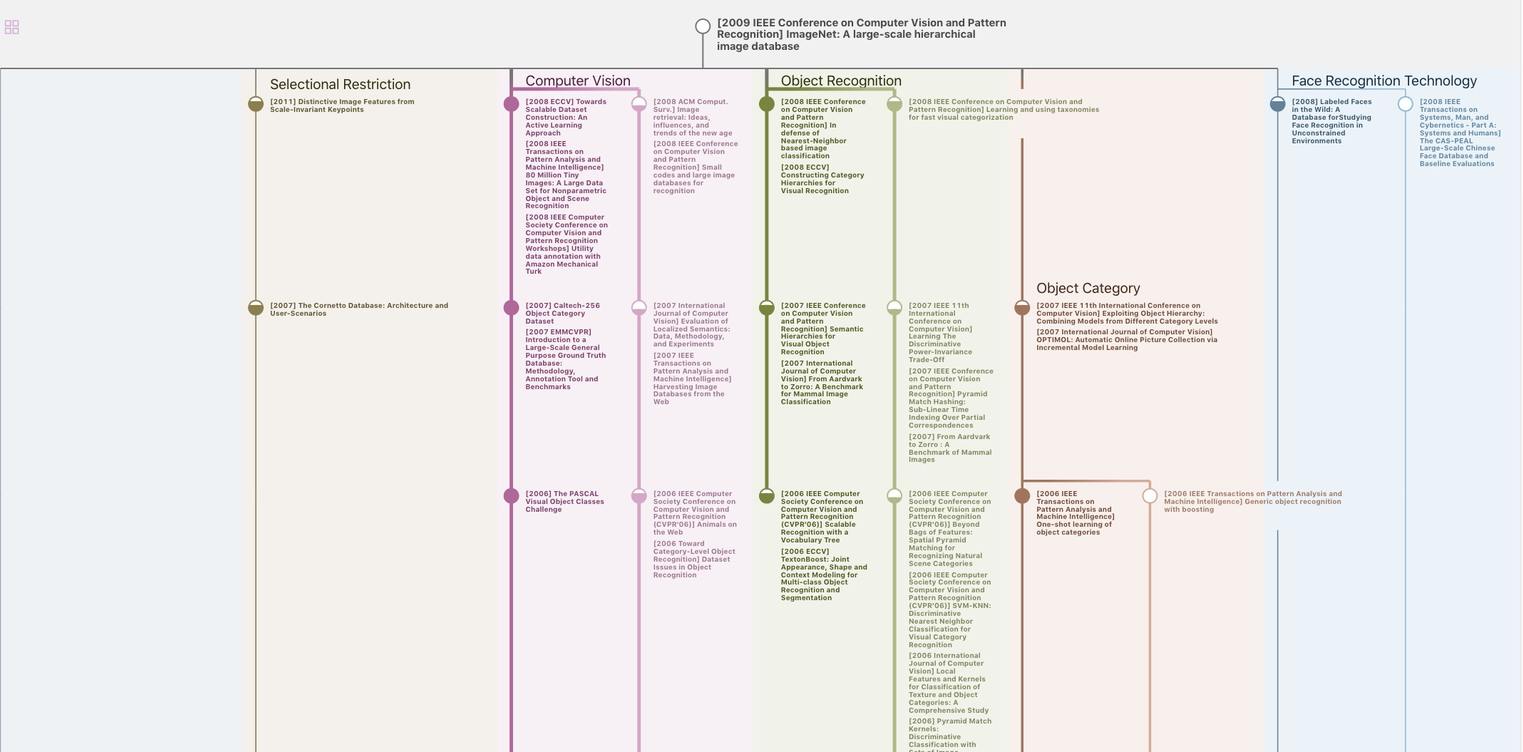
生成溯源树,研究论文发展脉络
Chat Paper
正在生成论文摘要