Faster Nonconvex Low-Rank Matrix Learning for Image Low-Level and High-Level Vision: A Unified Framework
Information Fusion(2024)
摘要
This study introduces a unified approach to tackle challenges in both low-level and high-level vision tasks for image processing. The framework integrates faster nonconvex low-rank matrix computations and continuity techniques to yield efficient and high-quality results. In addressing real-world image complexities like noise, variations, and missing data, the framework exploits the intrinsic low-rank structure of the data and incorporates specific residual measurements. The optimization problem for low-rank matrix learning is effectively solved using the nonconvex Proximal Block Coordinate Descent (PBCD) algorithm, resulting in nearly unbiased estimators. Rigorous theoretical analysis ensures both local and global convergence. The PBCD algorithm updates blocks of variables iteratively with closed-form solutions, adeptly handling nonconvexity and promoting faster convergence. Notably, the framework incorporates the randomized singular value decomposition (RSVD) technique and introduces a continuous strategy for adaptive model parameter updates. These strategic choices reduce computational complexity while maintaining result quality. They offer fine-tuned control over the desired rank of the learned matrix and enhance robustness in a straightforward manner. Furthermore, the versatility of the proposed nonconvex PBCD algorithm extends to handling problems with multiple variables, as supported by theoretical analysis. Experimental evaluations, spanning various image low-level and high-level vision tasks such as inpainting, classification, and clustering, validate the effectiveness and efficiency of our framework across diverse databases. The source code is available at https://github.com/ZhangHengMin/FNPBCD_LR.In a nutshell, our framework provides a unified solution to tackle both low-level and high-level vision tasks in images. By combining fast nonconvex low-rank matrix learning with adaptive parameter updates, we achieve efficient computation, yielding high-quality results that demonstrate robustness against various types of noise. The evaluations further endorse the reliability and applicability of our proposed framework.
更多查看译文
关键词
Faster low-rank matrix learning,Nonconvex PBCD algorithm,Nonconvex relaxation functions,Randomized SVD,Image low-level and high-level vision
AI 理解论文
溯源树
样例
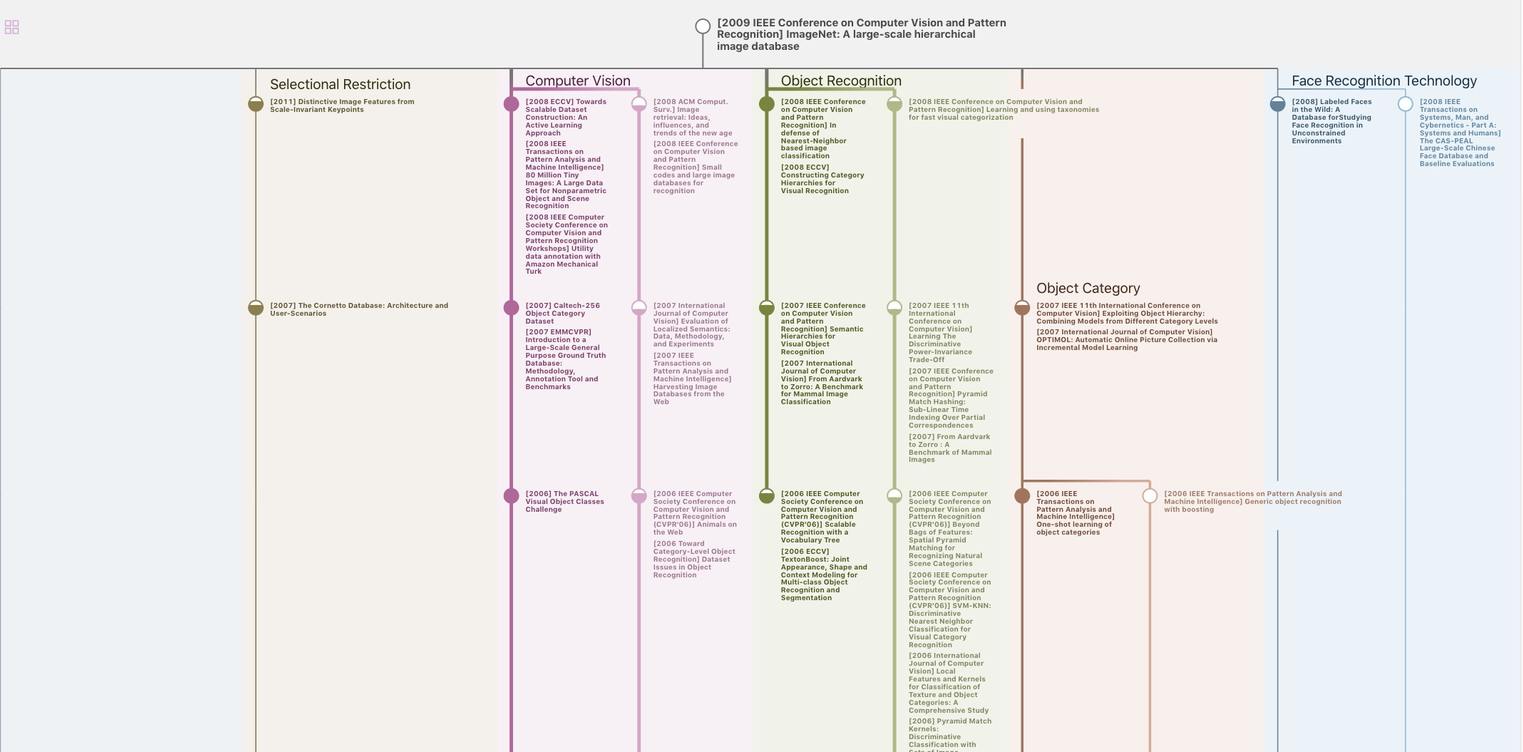
生成溯源树,研究论文发展脉络
Chat Paper
正在生成论文摘要