PEPSI: Pathology-Enhanced Pulse-Sequence-Invariant Representations for Brain MRI
Lecture Notes in Computer Science Medical Image Computing and Computer Assisted Intervention – MICCAI 2024(2024)
摘要
Remarkable progress has been made by data-driven machine-learning methods inthe analysis of MRI scans. However, most existing MRI analysis approaches arecrafted for specific MR pulse sequences (MR contrasts) and usually requirenearly isotropic acquisitions. This limits their applicability to diversereal-world clinical data, where scans commonly exhibit variations inappearances due to being obtained with varying sequence parameters,resolutions, and orientations – especially in the presence of pathology. Inthis paper, we propose PEPSI, the first pathology-enhanced, andpulse-sequence-invariant feature representation learning model for brain MRI.PEPSI is trained entirely on synthetic images with a novel pathology encodingstrategy, and enables co-training across datasets with diverse pathologies andmissing modalities. Despite variations in pathology appearances acrossdifferent MR pulse sequences or the quality of acquired images (e.g.,resolution, orientation, artifacts, etc), PEPSI produces a high-resolutionimage of reference contrast (MP-RAGE) that captures anatomy, along with animage specifically highlighting the pathology. Our experiments demonstratePEPSI's remarkable capability for image synthesis compared with thestate-of-the-art, contrast-agnostic synthesis models, as it accuratelyreconstructs anatomical structures while differentiating between pathology andnormal tissue. We further illustrate the efficiency and effectiveness of PEPSIfeatures for downstream pathology segmentations on five public datasetscovering white matter hyperintensities and stroke lesions. Code is available athttps://github.com/peirong26/PEPSI.
更多查看译文
关键词
Perfusion Imaging,Parallel Imaging,Susceptibility Weighted Imaging,Magnetic Resonance Imaging,Functional MRI
AI 理解论文
溯源树
样例
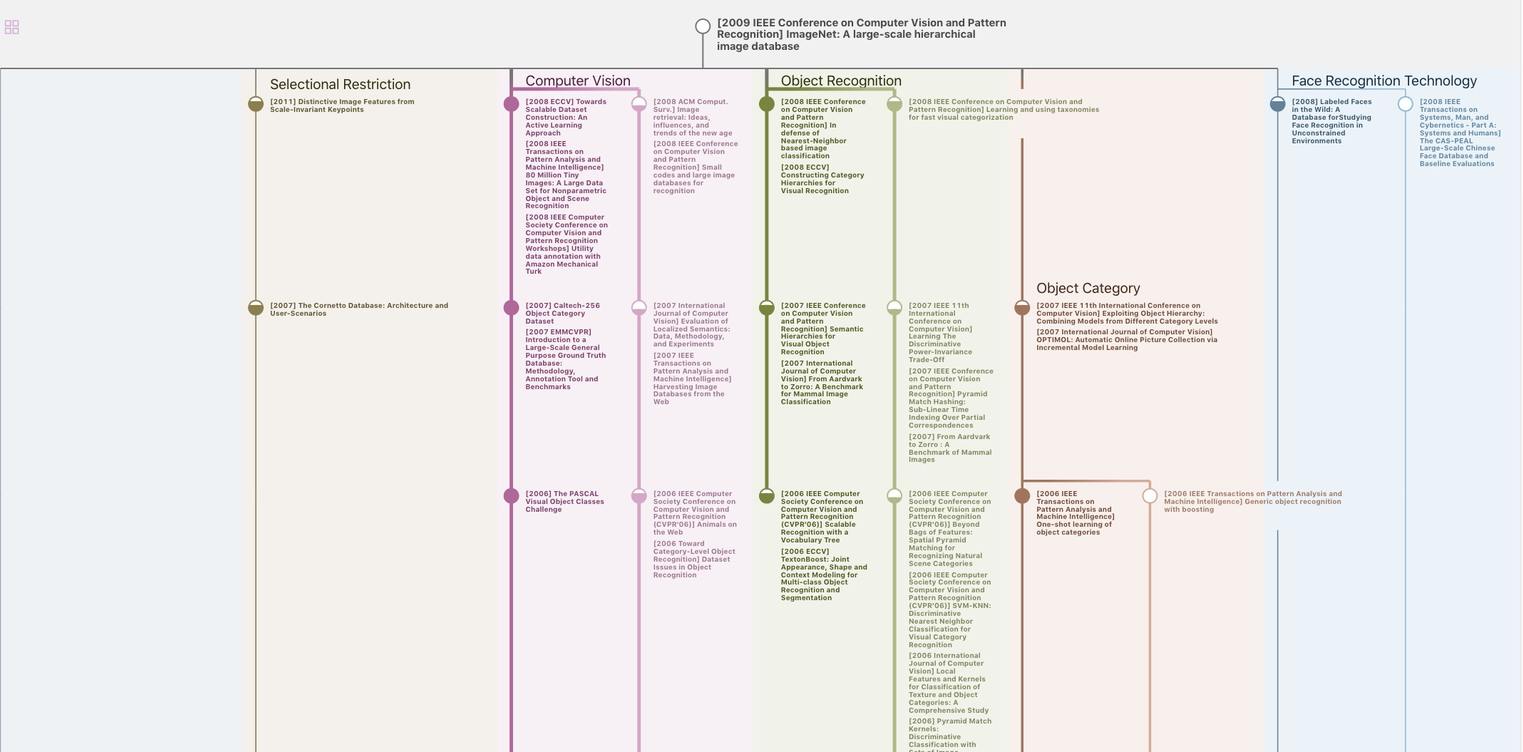
生成溯源树,研究论文发展脉络
Chat Paper
正在生成论文摘要