Data-Analytics-Driven Selection of Die Material in Multi-Material Co-Extrusion of Ti-Mg Alloys
MATHEMATICS(2024)
摘要
The selection of the most suitable material is one of the key decisions to be made during the design stage of a manufacturing process. Traditional approaches, such as Ashby maps based on material properties, are widely used in industry. However, in the production of multi-material components, the criteria for the selection can include antagonistic approaches. The aim of this work is to implement a methodology based on the results of process simulations for several materials and to classify them by applying an advanced data analytics method based on machine learning (ML)—in this case, the support vector regression (SVR) or multi-criteria decision-making (MCDM) methodology. Specifically, the multi-criteria optimization and compromise solution (VIKOR) was combined with entropy weighting methods. To achieve this, a finite element model (FEM) was built to evaluate the extrusion force and the die wear during the multi-material co-extrusion process of bimetallic Ti6Al4V-AZ31B billets. After applying SVR and VIKOR in combination with the entropy weighting methodology, a comparison was established based on material selection and the complexity of the methodology used. The results show that the material chosen in both methodologies is very similar, but the MCDM method is easier to implement because there is no need for evaluating the error of the prediction model, and the time required for data preprocessing is less than the time needed when applying SVR. This new methodology is proven to be effective as an alternative to traditional approaches and is aligned with the new trends in industry based on simulation and data analytics.
更多查看译文
关键词
data analytics,methodologies,multi-material,co-extrusion,FEM,machine learning,SVR,MCDM
AI 理解论文
溯源树
样例
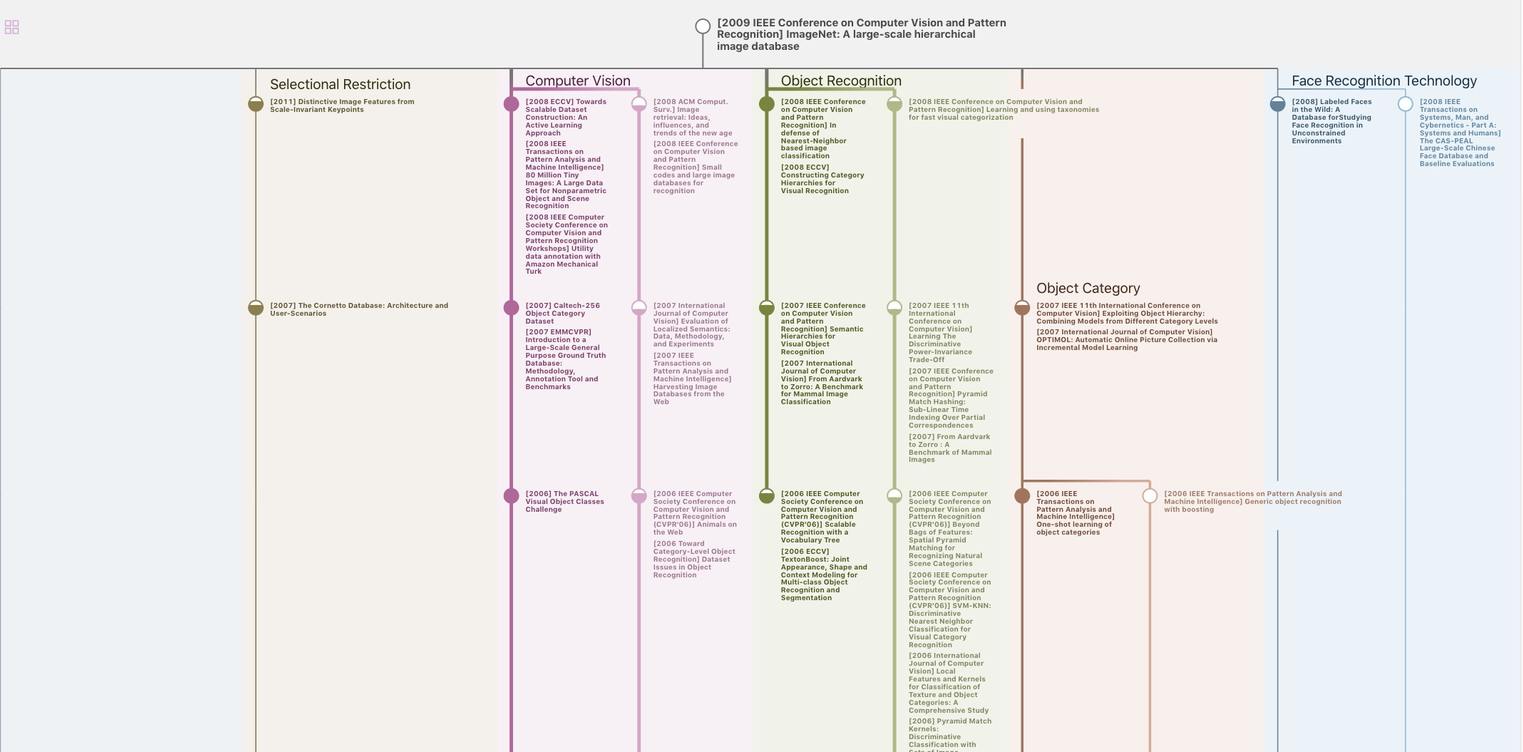
生成溯源树,研究论文发展脉络
Chat Paper
正在生成论文摘要