Better mapping of groundwater-surface water exchanges over the Seine River catchment in a surface hydrological model
crossref(2024)
摘要
Surface hydrological models usually define their modelling units using topographic catchment boundaries, which are then connected by the river network. Inter-catchment Groundwater Flows (IGFs) are water fluxes that do not respect these topographic boundaries. They can significantly influence river discharge. Therefore, surface hydrological models usually estimate IGFs indirectly, by adjusting the water balance across the topographic catchment. As they cannot be measured directly, the realism of these simulated fluxes can be questioned. Here, we investigate how a model calibration strategy could help to improve the physical realism of simulated IGFs. We propose a multi-objective calibration strategy, where we optimise the model simulation on two fluxes: river discharge and actual evapotranspiration using MODIS satellite estimates. Indeed, we hypothesize that better IGFs could be estimated if the water balance is more constrained by evaporation. We explore different objective functions to identify the most efficient way to use satellite data by looking at the model robustness in time and space. The Seine catchment is characterised by a complex, multi-layered aquifer system where the river loses water in some places and gains water in others. We evaluate the ability of the GRSD model, a semi-distributed hydrological model that implements the lumped GR5J in each subcatchment, to consistently describe this system thanks to this calibration strategy. The influence of four upstream dams is also considered in the modelling, as they have a significant impact on the hydrology. In particular, this work could help to understand the extent to which low flows are maintained naturally by groundwater or artificially by these dams. This work is partly funded by the ANR (CIPRHES project) and by the European Union’s HORIZON Research and Innovation Actions Programme under Grant Agreement No. 101059372 (STARS4Water project).
更多查看译文
AI 理解论文
溯源树
样例
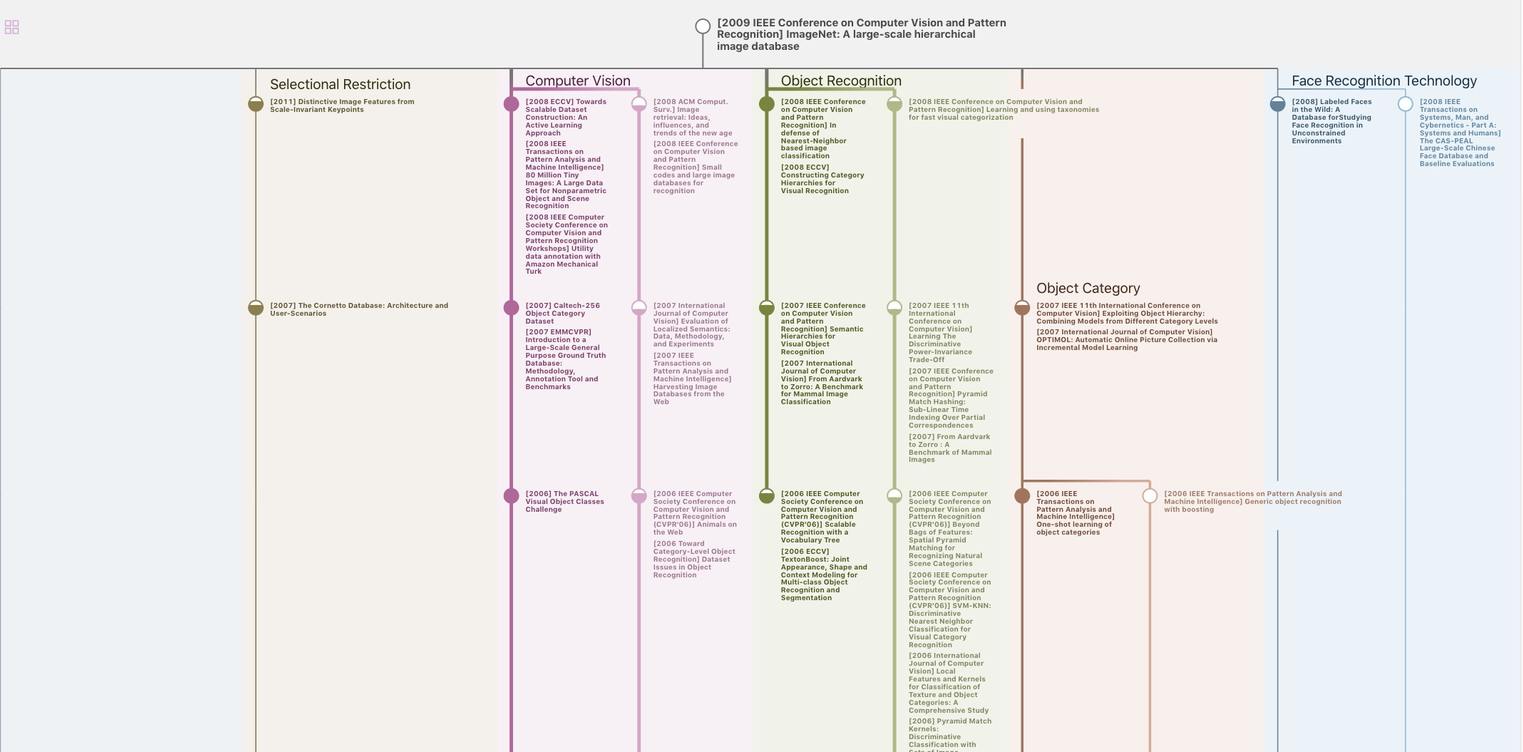
生成溯源树,研究论文发展脉络
Chat Paper
正在生成论文摘要