Joint Knowledge Graph and Large Language Model for Fault Diagnosis and Its Application in Aviation Assembly
IEEE TRANSACTIONS ON INDUSTRIAL INFORMATICS(2024)
摘要
In complex assembly industry settings, fault localization involves rapidly and accurately identifying the source of a fault and obtaining a troubleshooting solution based on fault symptoms. This study proposes a knowledge-enhanced joint model that incorporates aviation assembly knowledge graph (KG) embedding into large language models (LLMs). This model utilizes graph-structured Big Data within KGs to conduct prefix-tuning of the LLMs. The KGs for prefix-tuning enable an online reconfiguration of the LLMs, which avoids a massive computational load. Through the subgraph embedding learning process, the specialized knowledge of the joint model within the aviation assembly domain, especially in fault localization, is strengthened. In the context of aviation assembly functional testing, the joint model can generate knowledge subgraphs, fuse knowledge through retrieval augmentation, and ultimately provide knowledge-based reasoning responses. In practical industrial scenario experiments, the joint enhancement model demonstrates an accuracy of 98.5% for fault diagnosis and troubleshooting schemes.
更多查看译文
关键词
Data-driven,fault localization,intelligent fault diagnosis,knowledge graph (KG),large language model (LLM)
AI 理解论文
溯源树
样例
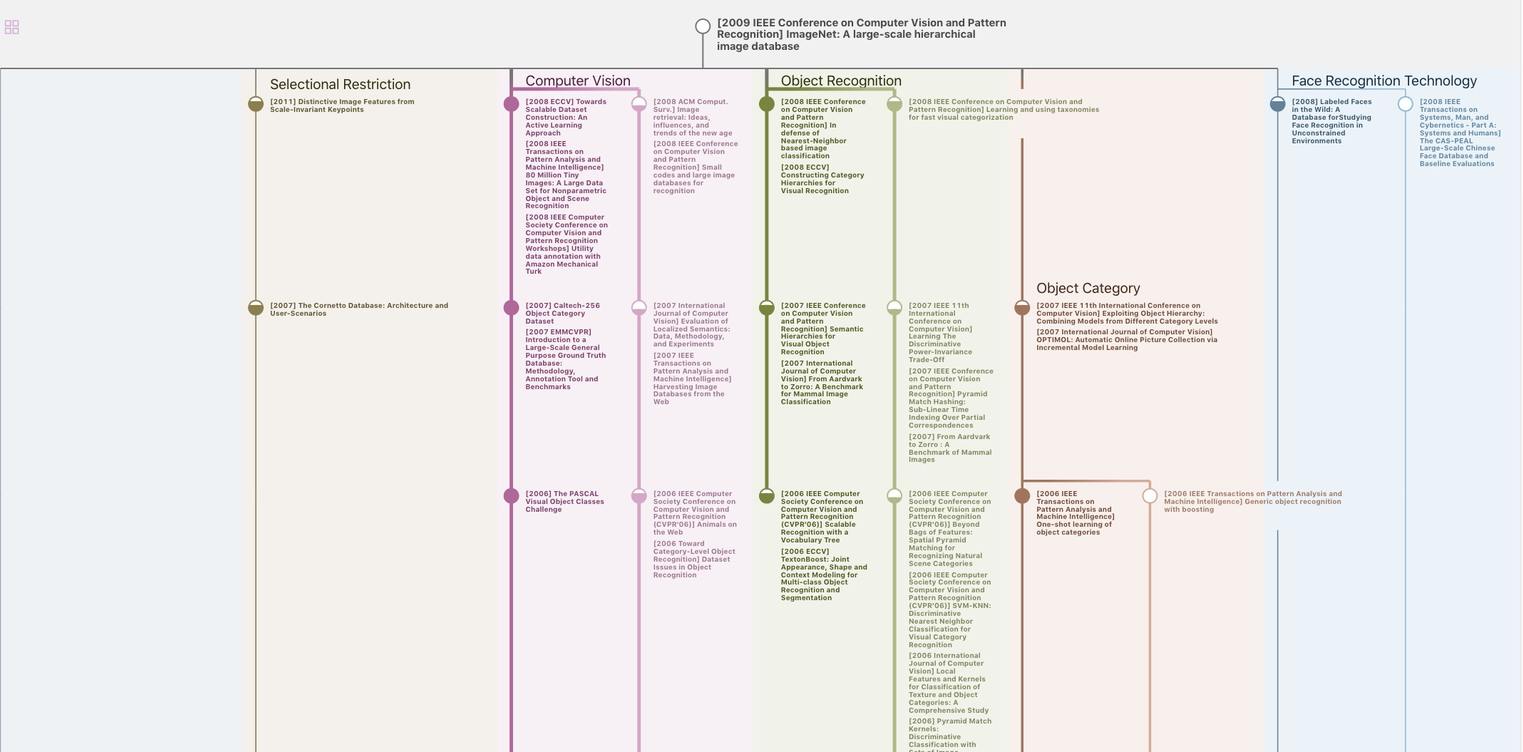
生成溯源树,研究论文发展脉络
Chat Paper
正在生成论文摘要