Data Assimilation Informed model Structure Improvement (DAISI) to improve prediction under climate change
crossref(2024)
摘要
Estimation of future streamflows is generally done using rainfall-runoff models to generate streamflow projections based on future climate inputs. Unfortunately, the performance of these models degrades significantly when predicting values outside of their calibration range, which undermines the credibility of projected scenarios. This abstract presents a method to analyze and improve the equations constituting a rainfall-runoff model structure in the context of climate change scenario modelling demonstrated with an application to the GR2M model and 201 catchments in South-East Australia. The method, termed "Data Assimilation Informed model Structure Improvement" (DAISI), enhances a rainfall-runoff model by combining data assimilation with polynomial updates of the state equations. The method is generic and modular, and consistently improves model performance across various metrics, including KGE, NSE on log-transformed flow, and flow duration curve bias. The updated model exhibits higher elasticity of runoff to rainfall, indicating potential significance for climate change simulations. The DAISI diagnostic identifies a reduced number of update configurations in the GR2M structure, with distinct regional patterns in three sub-regions (Western Victoria, central region, and Northern New South Wales). We suggest potential improvements for DAISI, such as incorporating additional observed variables like actual evapotranspiration to better constrain internal model fluxes.
更多查看译文
AI 理解论文
溯源树
样例
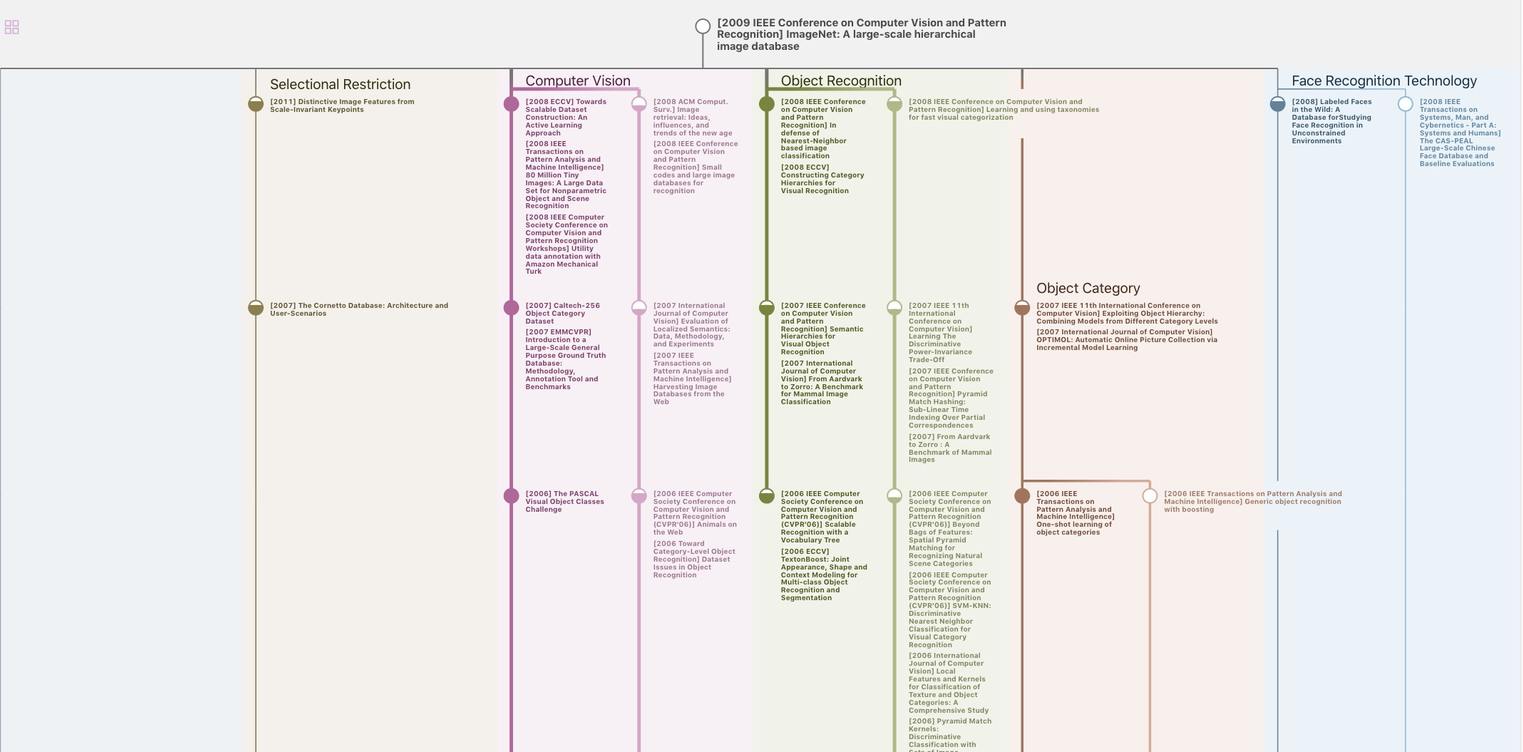
生成溯源树,研究论文发展脉络
Chat Paper
正在生成论文摘要