Pedestrian Trajectory Prediction Based on Social Interactions Learning with Random Weights
IEEE transactions on multimedia(2024)
摘要
Pedestrian trajectory prediction is a critical technology in the evolution of self-driving cars toward complete artificial intelligence. Over recent years, focusing on the trajectories of pedestrians to model their social interactions has surged with great interest in more accurate trajectory predictions. However, existing methods for modeling pedestrian social interactions rely on pre-defined rules, struggling to capture non-explicit social interactions. In this work, we propose a novel framework named DTGAN, which extends the application of Generative Adversarial Networks (GANs) to graph sequence data, with the primary objective of automatically capturing implicit social interactions and achieving precise predictions of pedestrian trajectory. DTGAN innovatively incorporates random weights within each graph to eliminate the need for pre-defined interaction rules. We further enhance the performance of DTGAN by exploring diverse task loss functions during adversarial training, which yields improvements of 16.7% and 39.3% on metrics ADE and FDE, respectively. The effectiveness and accuracy of our framework are verified on two public datasets. The experimental results show that our proposed DTGAN achieves superior performance and is well able to understand pedestrians' intentions.
更多查看译文
关键词
Pedestrians,Trajectory,Generative adversarial networks,Training,Long short term memory,Task analysis,Predictive models,Social interactions,GAN,graph with random weights
AI 理解论文
溯源树
样例
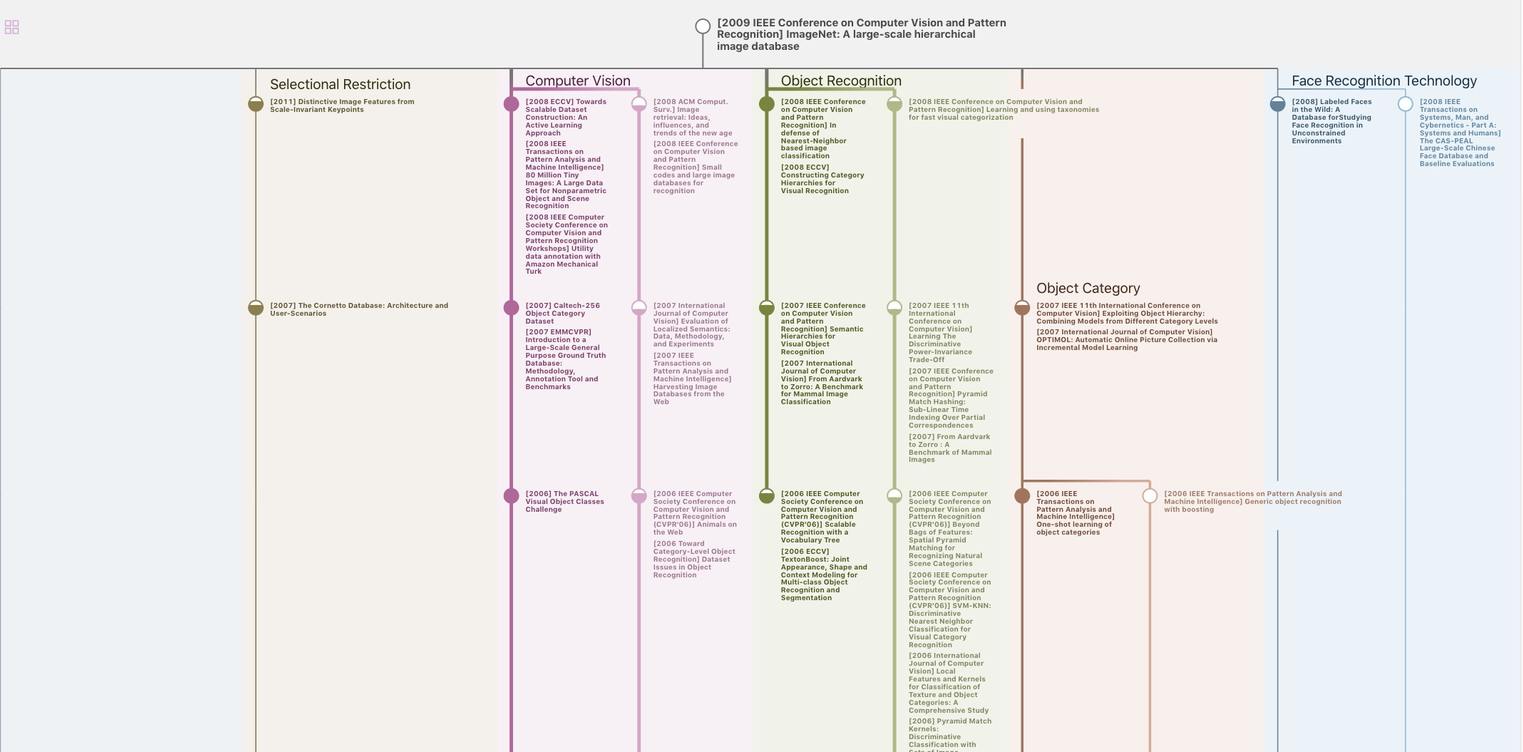
生成溯源树,研究论文发展脉络
Chat Paper
正在生成论文摘要