Statistical and neural network-based AOD data fusion with Geostationary satellite instruments: GEMS, AMI, and GOCI-II.
crossref(2024)
摘要
Aerosol optical depth (AOD) data fusion for aerosol datasets obtained from the Geostationary Korea Multi-Purpose Satellite (GEO-KOMPSAT; GK) series was conducted through the application of both statistical and deep neural network (DNN)-based methodologies. The GK mission incorporates the Advanced Meteorological Imager (AMI) on GK-2A, as well as the Geostationary Environment Monitoring Spectrometer (GEMS) and Geostationary Ocean Color Imager-II (GOCI-II) on GK-2B. The statistical fusion approach rectified biases in each aerosol product by assuming a Gaussian error distribution. Utilizing Maximum Likelihood Estimation (MLE) fusion, the technique accounted for pixel-level uncertainties by weighting the root-mean-square error of each AOD product for individual pixels. A DNN-based fusion model was trained to align with Aerosol Robotic Network AOD values through fully connected hidden layers. The results of both statistical and DNN-based fusion generally surpassed the performance of individual GEMS and AMI AOD datasets in East Asia (R = 0.888; RMSE = −0.188; MBE = −0.076; 60.6% within EE for MLE AOD; R = 0.905; RMSE = 0.161; MBE = −0.060; 65.6% within EE for DNN AOD). Particularly, focusing on AOD around the Korean peninsula, encompassing all aerosol products, yielded significantly improved outcomes (R = 0.911; RMSE = 0.113; MBE = −0.047; 73.3% within EE for MLE AOD; R = 0.912; RMSE = 0.102; MBE = −0.028; 78.2% within EE for DNN AOD). The DNN AOD demonstrated effective handling of the rapid increase in uncertainty at higher aerosol loadings. Overall, the fusion AOD, particularly DNN AOD, closely matched with the performance of the Moderate Resolution Imaging Spectroradiometer Dark Target algorithm, exhibiting slightly less variance and a negative bias. Both fusion algorithms stabilized diurnal error variations and provided additional insights into hourly aerosol evolution.
更多查看译文
AI 理解论文
溯源树
样例
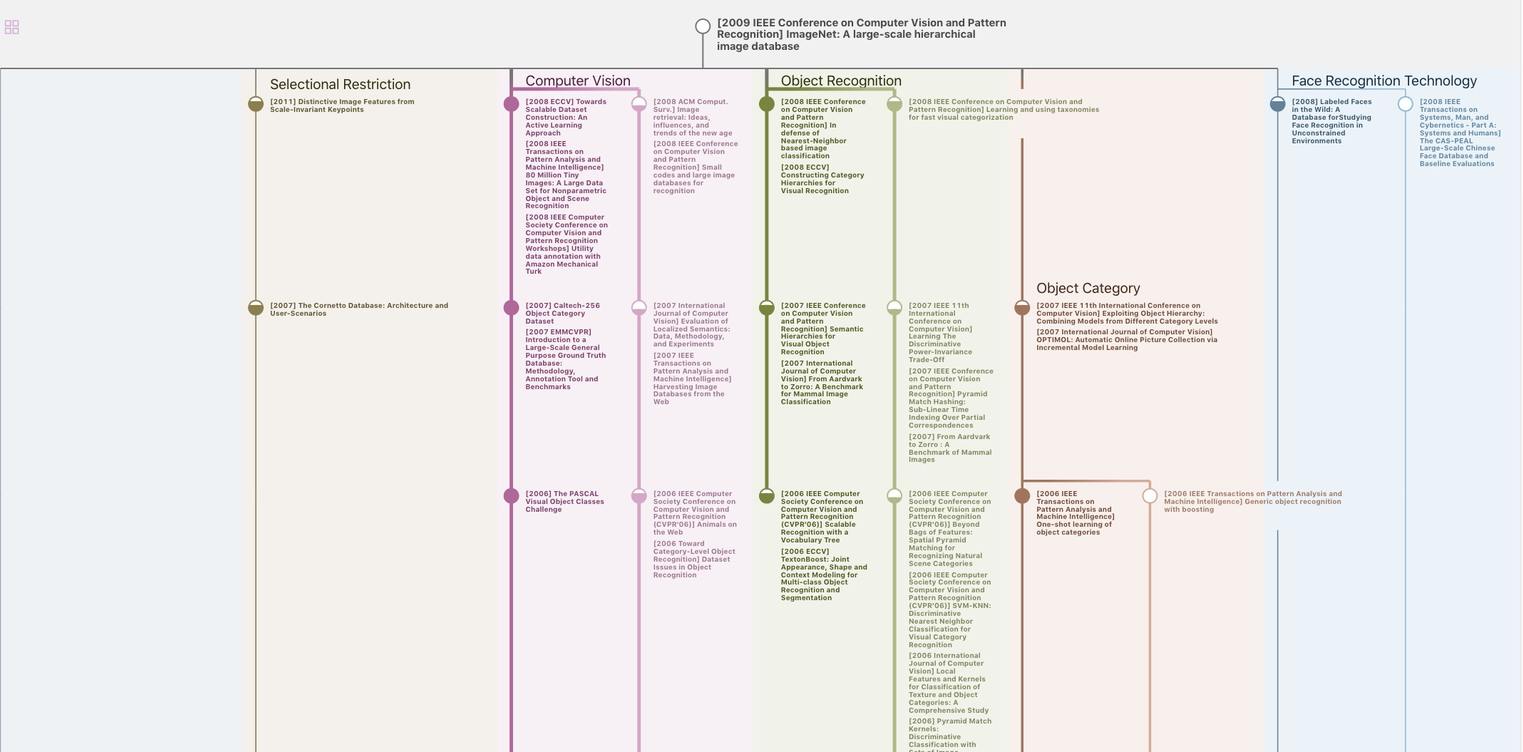
生成溯源树,研究论文发展脉络
Chat Paper
正在生成论文摘要