Machine learning-based discrete post-processing of visibility ensemble forecasts
crossref(2024)
摘要
In aviation meteorology, as well as in water and road transportation, the accurate andreliable prediction of visibility is of utmost importance. Despite various meteorologicalservices offering ensemble forecasts for visibility, the predictive accuracy and reliability forthis parameter are notably lower compared to variables like temperature or wind speed.Therefore, it is strongly recommended to implement some form of calibration, typicallyinvolving the estimation of the predictive distribution through parametric or non-parametricmethods, including machine learning techniques. The World Meteorological Organizationsuggests that visibility observations should be reported in discrete values, turning thepredictive distribution into a discrete probability law. Consequently, the calibration processcan be simplified to a classification problem. This study investigates the predictiveperformance of locally, semi-locally, and regionally trained proportional odds logisticregression (POLR) and multilayer perceptron (MLP) neural network classifiers usingvisibility ensemble forecasts from the European Centre for medium-range weatherforecasts. The findings reveal that while climatological forecasts surpass the rawensemble, post-processing leads to a substantial improvement in forecast skill. Overall,POLR models exhibit superiority over their MLP counterparts. ReferenceBaran, S., Lakatos, M., Statistical post-processing of visibility ensemble forecasts.Meteorol. Appl. 30 (2023), paper e2157, doi:10.1002/met.2157. *Research is supported by the ÚNKP-23-3 New National Excellence Program of theHungarian Ministry for Culture and Innovation from the source of the National Research,Development and Innovation Fund and the Hungarian National Research, Developmentand Innovation Office under Grant No. K142849.
更多查看译文
AI 理解论文
溯源树
样例
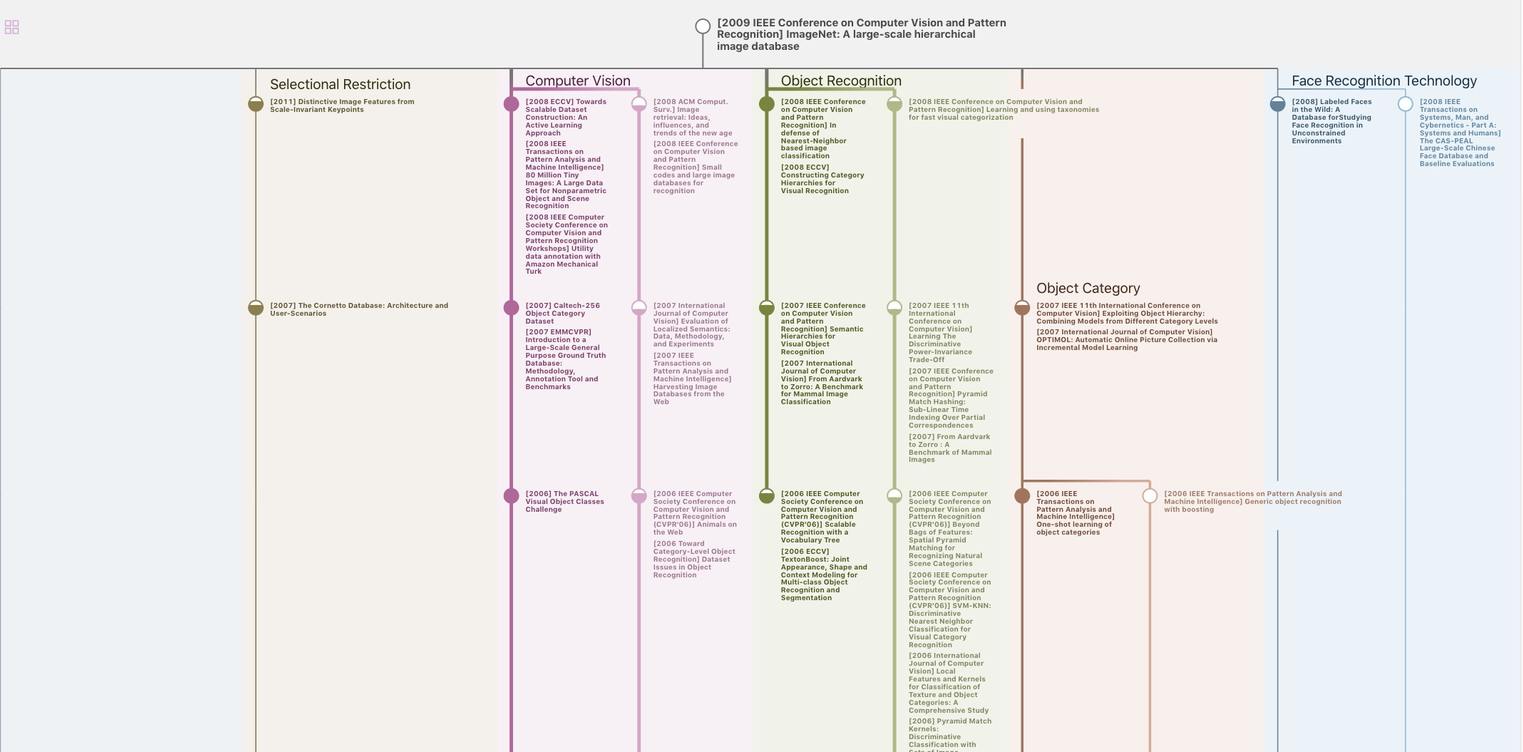
生成溯源树,研究论文发展脉络
Chat Paper
正在生成论文摘要