Habit Classification of PHIPS Stereo-Microscopic Ice Crystal Images
crossref(2024)
摘要
Clouds play a major role in the global radiative budget. Microphysical properties, such as the shape (or habit), of individual ice crystals define their optical properties and consequently affect the cloud radiative effect. Therefore, a better understanding of ice crystal morphology is crucial in improving climate modelling. With the Particle Habit Imaging and Polar Scattering (PHIPS) instrument, a unique airborne measurement probe that simultaneously captures optical and microphysical properties of single ice crystals, we collected a large dataset of high resolution in-situ microscopic images of natural ice crystals. In addition to the outstanding image quality and corresponding visible level of detail, one of the key features of the PHIPS is that it simultaneously takes two images of each individual ice crystal under different viewing angles, resulting in stereo images. Over 100,000 stereo images from multiple field campaigns have been manually classified using a tree-based classification system with 22 leaf nodes. Due to increasing instrument performance and frequent application in field projects an ever-increasing data amount makes manual classification of future PHIPS images impractical, and has motivated us to develop an automated classification scheme. To automate classifying our images, we apply deep learning methods to our data and use convolutional neural networks (CNNs). The previously manually classified data is split into a training, validation and test dataset. A description of our classification system of ice crystal morphology will be given. In addition, performance results for different CNN architectures will be presented.
更多查看译文
AI 理解论文
溯源树
样例
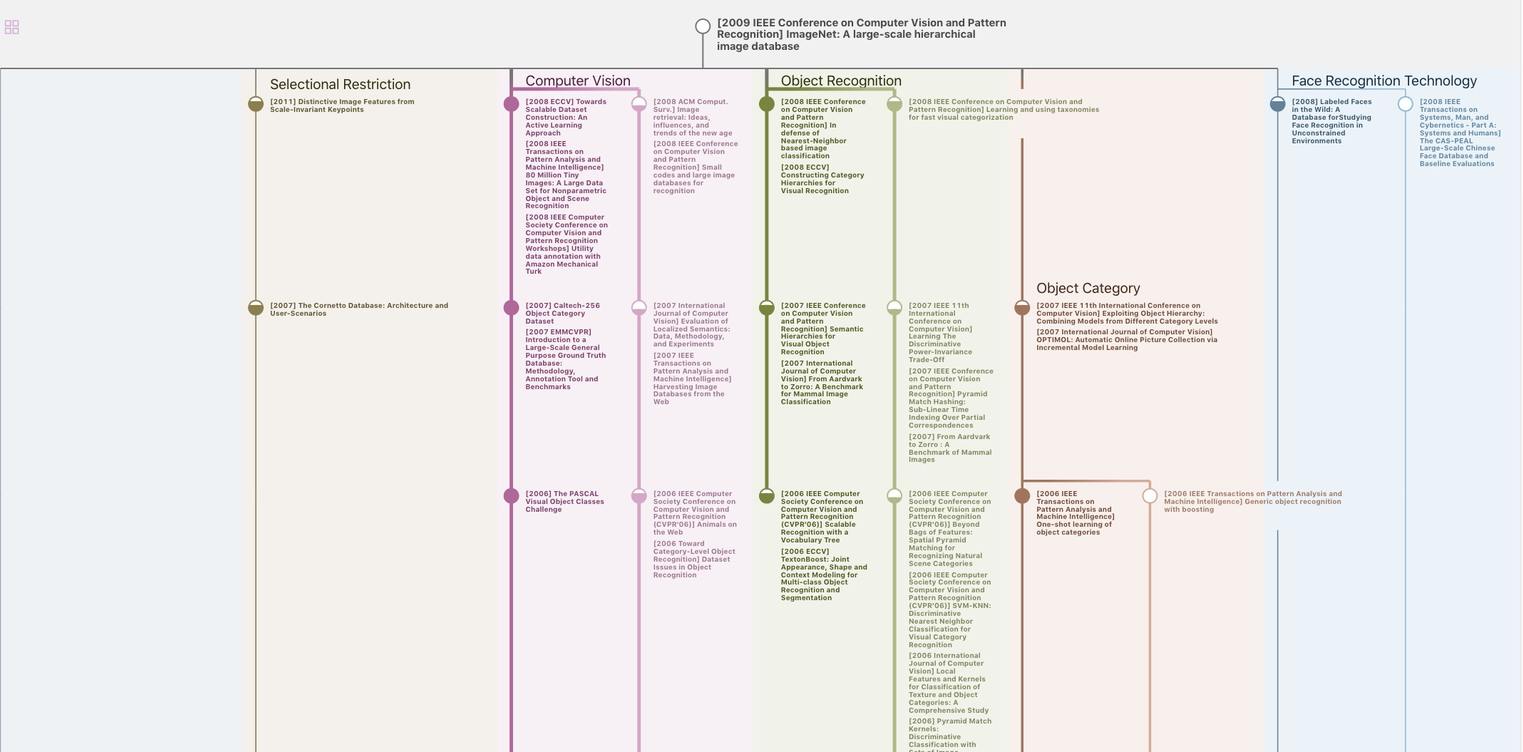
生成溯源树,研究论文发展脉络
Chat Paper
正在生成论文摘要