Are Models Biased on Text Without Gender-related Language?
ICLR 2024(2024)
摘要
In the large language models era, it is imperative to measure and understand how gender biases present in the training data influence model behavior. Previous works construct benchmarks around known stereotypes (e.g., occupations) and demonstrate high levels of gender bias in large language models, raising serious concerns about models exhibiting undesirable behaviors. We expand on existing literature by asking the question: \textit{Do large language models still favor one gender over the other in non-stereotypical settings?} To tackle this question, we restrict language model evaluation to a \textit{neutral} subset, in which sentences are free of pronounced word-gender associations. After characterizing these associations in terms of pretraining data statistics, we use them to (1) create a new benchmark with low gender-word associations, and (2) repurpose popular benchmarks in the gendered pronoun setting | WinoBias and \Winogender |, removing pronounced gender-correlated words. Surprisingly, when testing $20+$ models (e.g., Llama-2, Pythia, and OPT) in the proposed benchmarks, we still detect critically high gender bias across all tested models. For instance, after adjusting for strong word-gender associations, we find that all models still exhibit clear gender preferences in about $60\%$-$95\%$ of the sentences, representing a small change (up to $5\%$) from the original \textit{stereotypical} setting. By demonstrating that measured bias is not necessarily due to the presence of highly gender-associated words, our work highlights important questions about bias evaluation as well as potentially underlying model biases.
更多查看译文
关键词
Large language models,bias evaluation,gender bias,gender co-occurring words,gender-invariant,pretraining data statistics
AI 理解论文
溯源树
样例
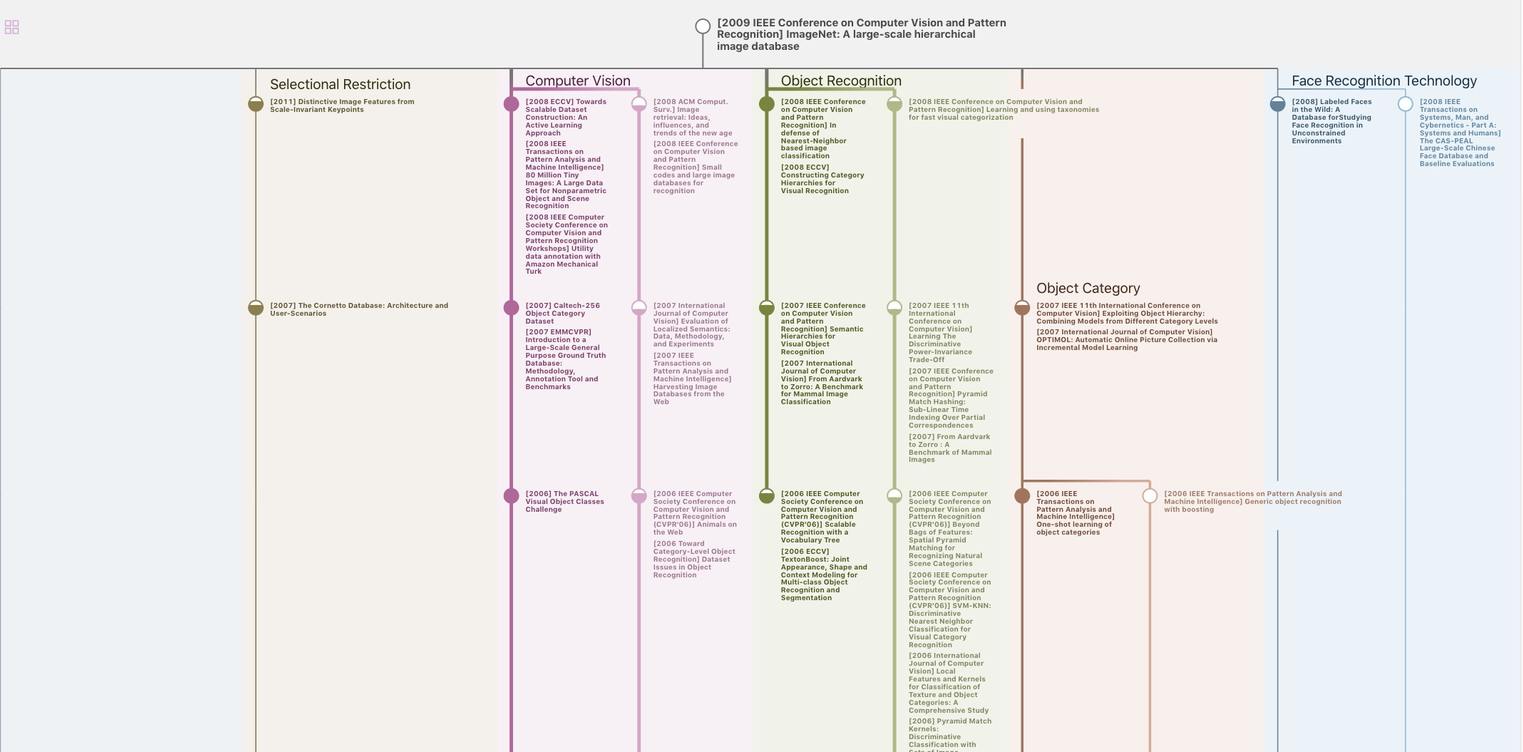
生成溯源树,研究论文发展脉络
Chat Paper
正在生成论文摘要