LUMNet: Land Use Knowledge Guided Multiscale Network for Height Estimation From Single Remote Sensing Images
IEEE GEOSCIENCE AND REMOTE SENSING LETTERS(2024)
摘要
For estimating ground object height from single remote sensing images, this study proposes a land use knowledge guided multiscale height estimation network (LUMNet), which takes single image and land use data as inputs and produces an estimated height map as an output. First, in the encoder part, the visual geometry group network (VGGNet) is used to extract multilevel deep semantic features from images. Second, the features of the encoder part are fused with those of the decoder part through skip connection with land use knowledge attention weight and dense atrous spatial pyramid pooling (DenseASPP). Third, in the decoder part, the features are decoded using upsampling and convolution, and a joint loss function is constructed to supervise network training. Experiments show that the proposed method achieves the best visualizations and quantitative evaluation results among all the tested methods. For the Vaihingen dataset, the root mean square error (RMSE), mean absolute error (MAE), and zero-mean normalized cross correlation (ZNCC) of LUMNet are 1.523, 0.969, and 0.908, respectively. For the Potsdam dataset, these are 2.158, 1.222, and 0.871, respectively. The source code of LUMNet has been made public at the following link: https://figshare.com/s/eaf206879ade35a61f88.
更多查看译文
关键词
Estimation,Feature extraction,Data mining,Convolution,Remote sensing,Convolutional neural networks,Training,Attention weight,dense atrous spatial pyramid pooling (DenseASPP),height estimation,joint loss function,land use data,single remote sensing image
AI 理解论文
溯源树
样例
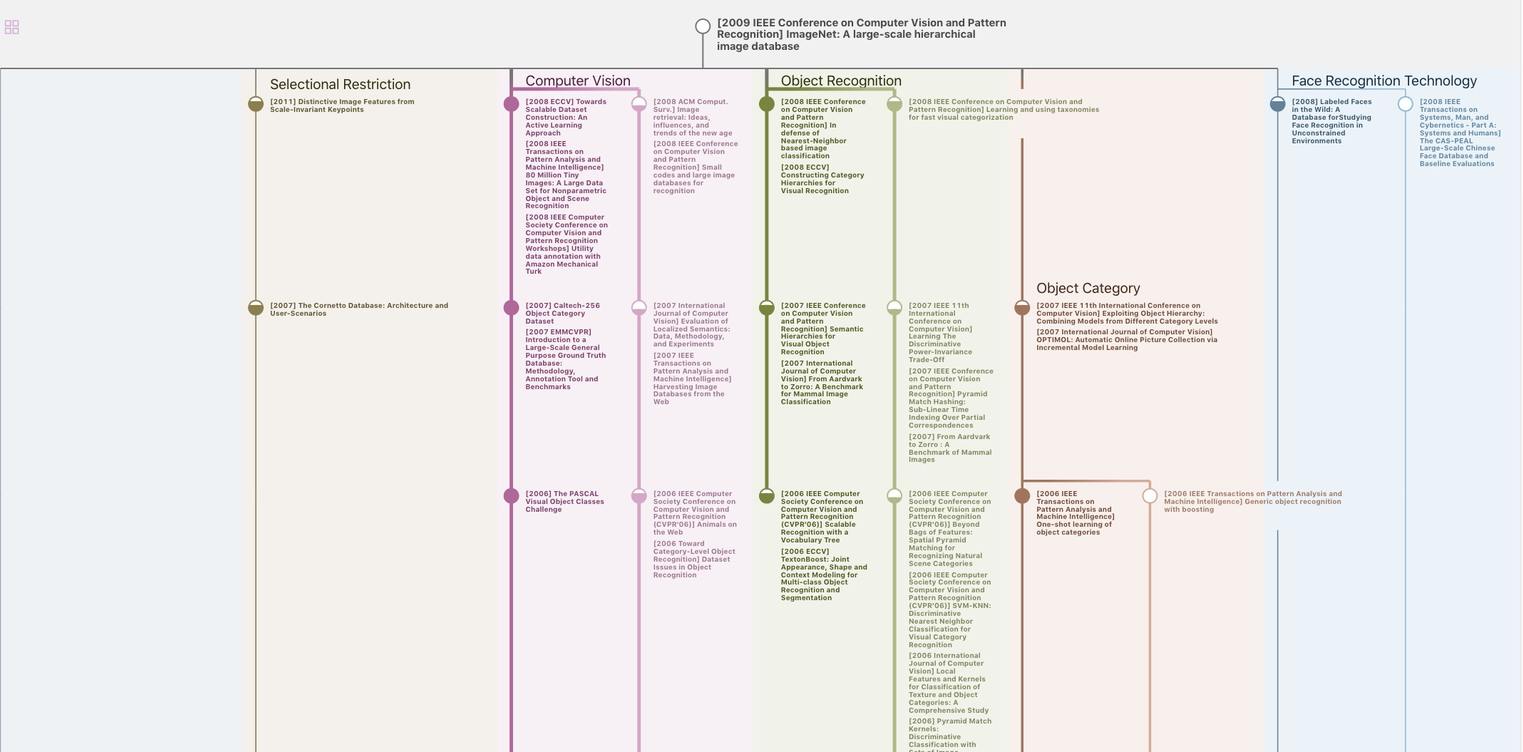
生成溯源树,研究论文发展脉络
Chat Paper
正在生成论文摘要