Impacts of source morphology on the detectability of subhalos in strong lenses
arxiv(2024)
摘要
We provide an analysis of a convolutional neural network's ability to
identify the lensing signal of single dark matter subhalos in strong
galaxy-galaxy lenses in the presence of increasingly complex source light
morphology. We simulate a balanced dataset of 800,000 strong lens images both
perturbed and unperturbed by a single subhalo ranging in virial mass between
10^7.5 M_⊙ - 10^11M_⊙ and characterise the source complexity
by the number of Sersic clumps present in the source plane ranging from 1 to 5.
Using the ResNet50 architecture we train the network to classify images as
either perturbed or unperturbed. We find that the network is able to detect
subhalos at low enough masses to distinguish between dark matter models even
with complex sources and that source complexity has little impact on the
accuracy beyond 3 clumps. The model was more confident in its classification
when the clumps in the source were compact, but cared little about their
spatial distribution. We also tested for the resolution of the data, finding
that even in conditions akin to natural seeing the model was still able to
achieve an accuracy of 74
this is heavily dominated by the high mass subhalos. It's robustness against
resolution is attributed to the model learning the flux ratio anomalies in the
perturbed lenses which are conserved in the lower resolution data.
更多查看译文
AI 理解论文
溯源树
样例
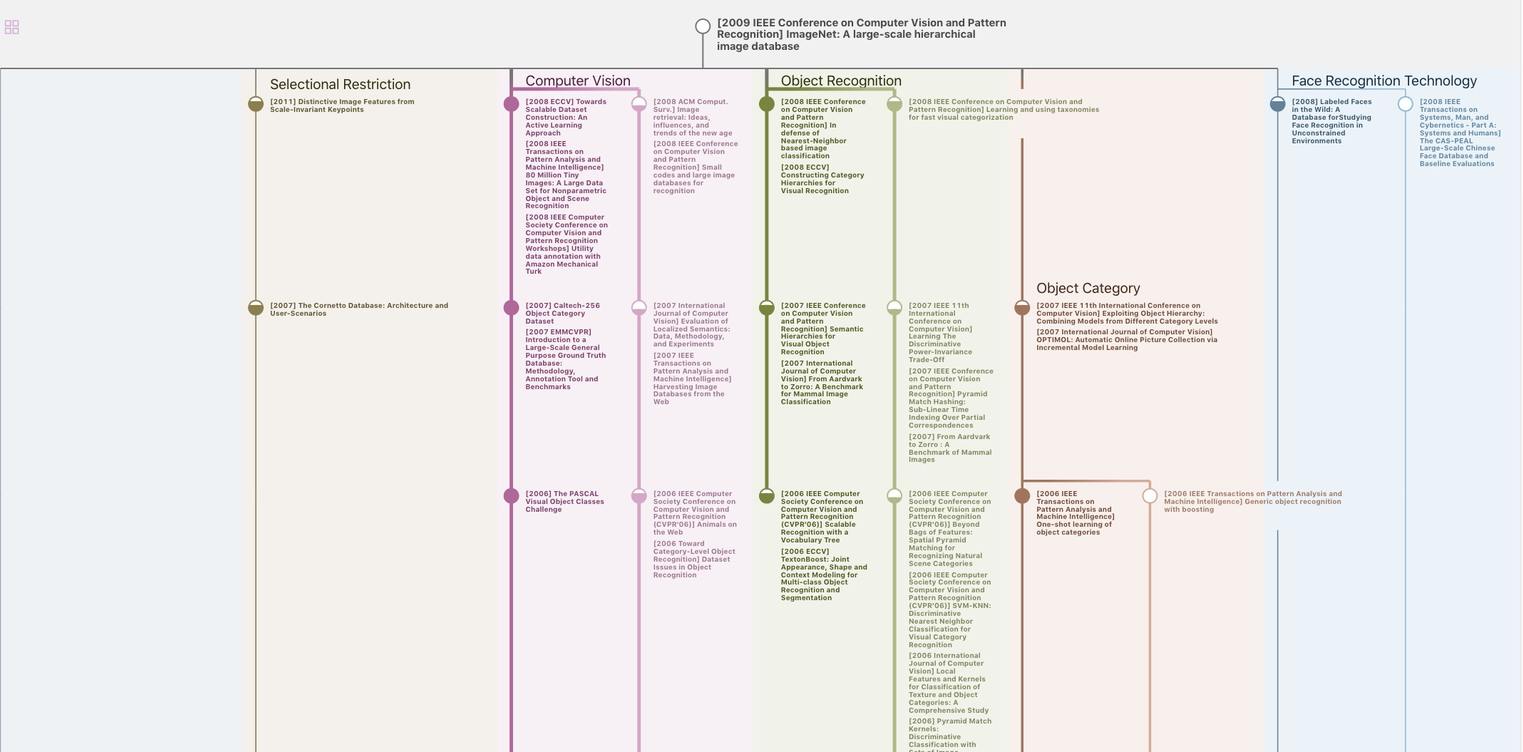
生成溯源树,研究论文发展脉络
Chat Paper
正在生成论文摘要