Multi-task Supervised Contrastive Learning for Chest X-ray Diagnosis: A Two-Stage Hierarchical Classification Framework for COVID-19 Diagnosis
Applied soft computing(2024)
摘要
Global pandemics have posed great challenges, such as limited samples and the scarcity of carefully curated datasets, in creating reliable models for chest X-ray (CXR) diagnosis. A common approach is to leverage pre-trained deep learning models using large datasets and then fine-tune model parameters on the target CXR dataset. However, in the presence of data bias, it is prone to shortcut learning, where the model learns non-disease-related features. To enable the model to learn robust features from the target dataset, we propose a new training framework called Multi-task Supervised Contrastive Learning (MTSCL). In MTSCL, we concatenate the weighted representations of two supervised tasks and implicitly contrast the representations from the large-scale and target datasets. It helps the model focus on disease-related features in the target dataset. This paper applies the MTSCL model to a two-stage hierarchical classification framework. In the first stage, the MTSCL model detects lung abnormalities, and then tree-based models are applied to classify the abnormalities as pneumonia or COVID-19 in the second stage. The experimental results show that, in the first stage, the MTSCL model outperforms other training methods in terms of generalization with an average AUC improvement of 1.03%-5.33% and has better abnormality localization capability. In the second stage, the model generalization is enhanced by incorporating lung masks and using MTSCL model-generated lesion masks for image preprocessing. Furthermore, the addition of wavelet components manipulation on top of the previous improvements further increases the generalization performance for distinguishing COVID-19 from typical pneumonia with an average AUC improvement of 12.38%. Overall, the two-stage hierarchical classification framework exhibits superior generalization ability for normal, pneumonia, and COVID-19 classification, achieving an average accuracy of 75.19% in two external datasets, outperforming state-of-the-art deep learning models (71.00%-74.08%) and classical machine learning models (67.80%-68.67%).
更多查看译文
关键词
Chest X-ray,Contrastive learning,COVID-19,Model generalization,Multi -task learning
AI 理解论文
溯源树
样例
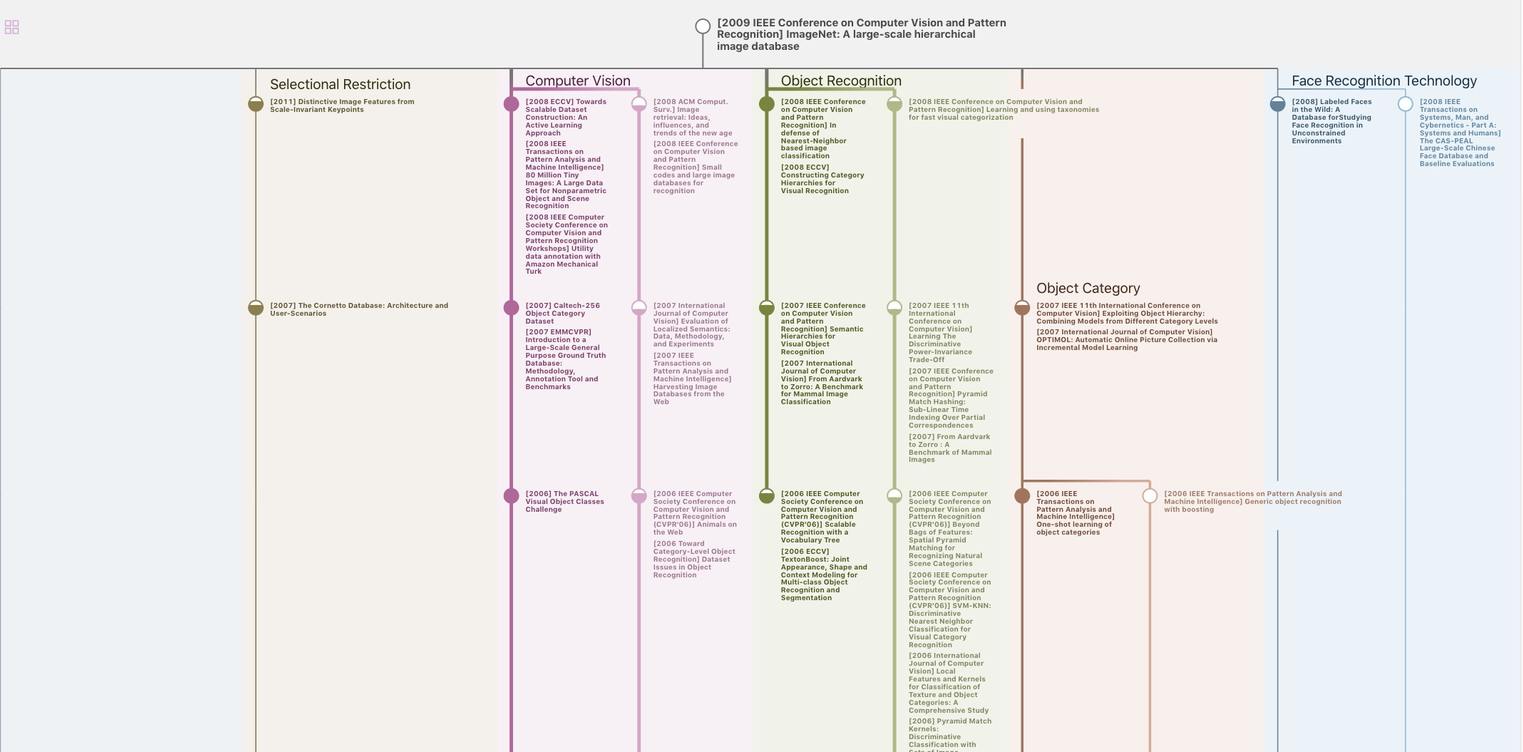
生成溯源树,研究论文发展脉络
Chat Paper
正在生成论文摘要