Towards Controllable Time Series Generation
arXiv (Cornell University)(2024)
摘要
Time Series Generation (TSG) has emerged as a pivotal technique insynthesizing data that accurately mirrors real-world time series, becomingindispensable in numerous applications. Despite significant advancements inTSG, its efficacy frequently hinges on having large training datasets. Thisdependency presents a substantial challenge in data-scarce scenarios,especially when dealing with rare or unique conditions. To confront thesechallenges, we explore a new problem of Controllable Time Series Generation(CTSG), aiming to produce synthetic time series that can adapt to variousexternal conditions, thereby tackling the data scarcity issue. In this paper, we propose Controllable Time Series(), an innovative VAE-agnostic framework tailored for CTSG. A keyfeature of is that it decouples the mapping process from standardVAE training, enabling precise learning of a complex interplay between latentfeatures and external conditions. Moreover, we develop a comprehensiveevaluation scheme for CTSG. Extensive experiments across three real-world timeseries datasets showcase 's exceptional capabilities in generatinghigh-quality, controllable outputs. This underscores its adeptness inseamlessly integrating latent features with external conditions. Extending to the image domain highlights its remarkable potential forexplainability and further reinforces its versatility across differentmodalities.
更多查看译文
关键词
Dynamic Time Warping
AI 理解论文
溯源树
样例
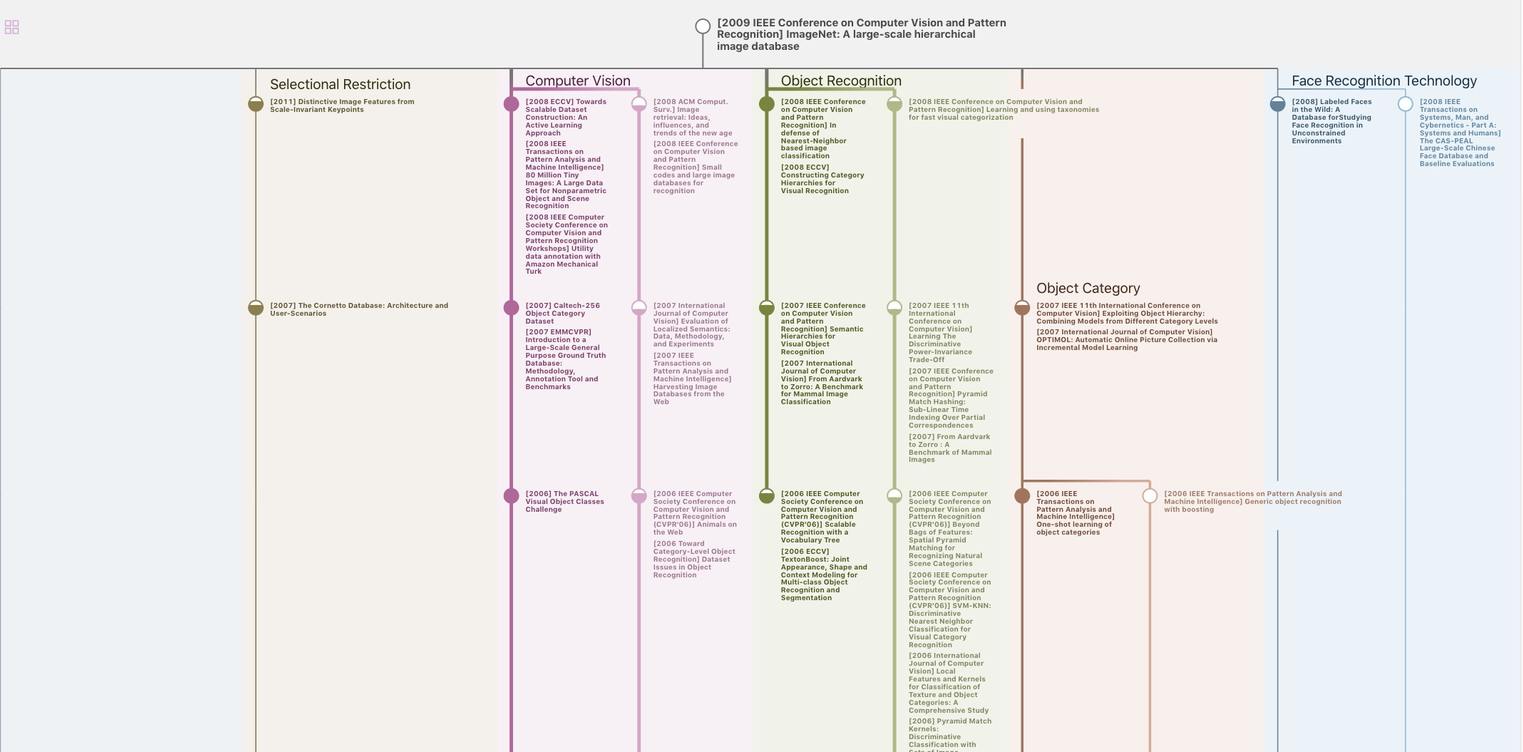
生成溯源树,研究论文发展脉络
Chat Paper
正在生成论文摘要