Unsupervised Incremental Learning with Dual Concept Drift Detection for Identifying Anomalous Sequences
CoRR(2024)
摘要
In the contemporary digital landscape, the continuous generation of extensive
streaming data across diverse domains has become pervasive. Yet, a significant
portion of this data remains unlabeled, posing a challenge in identifying
infrequent events such as anomalies. This challenge is further amplified in
non-stationary environments, where the performance of models can degrade over
time due to concept drift. To address these challenges, this paper introduces a
new method referred to as VAE4AS (Variational Autoencoder for Anomalous
Sequences). VAE4AS integrates incremental learning with dual drift detection
mechanisms, employing both a statistical test and a distance-based test. The
anomaly detection is facilitated by a Variational Autoencoder. To gauge the
effectiveness of VAE4AS, a comprehensive experimental study is conducted using
real-world and synthetic datasets characterized by anomalous rates below 10%
and recurrent drift. The results show that the proposed method surpasses both
robust baselines and state-of-the-art techniques, providing compelling evidence
for their efficacy in effectively addressing some of the challenges associated
with anomalous sequence detection in non-stationary streaming data.
更多查看译文
AI 理解论文
溯源树
样例
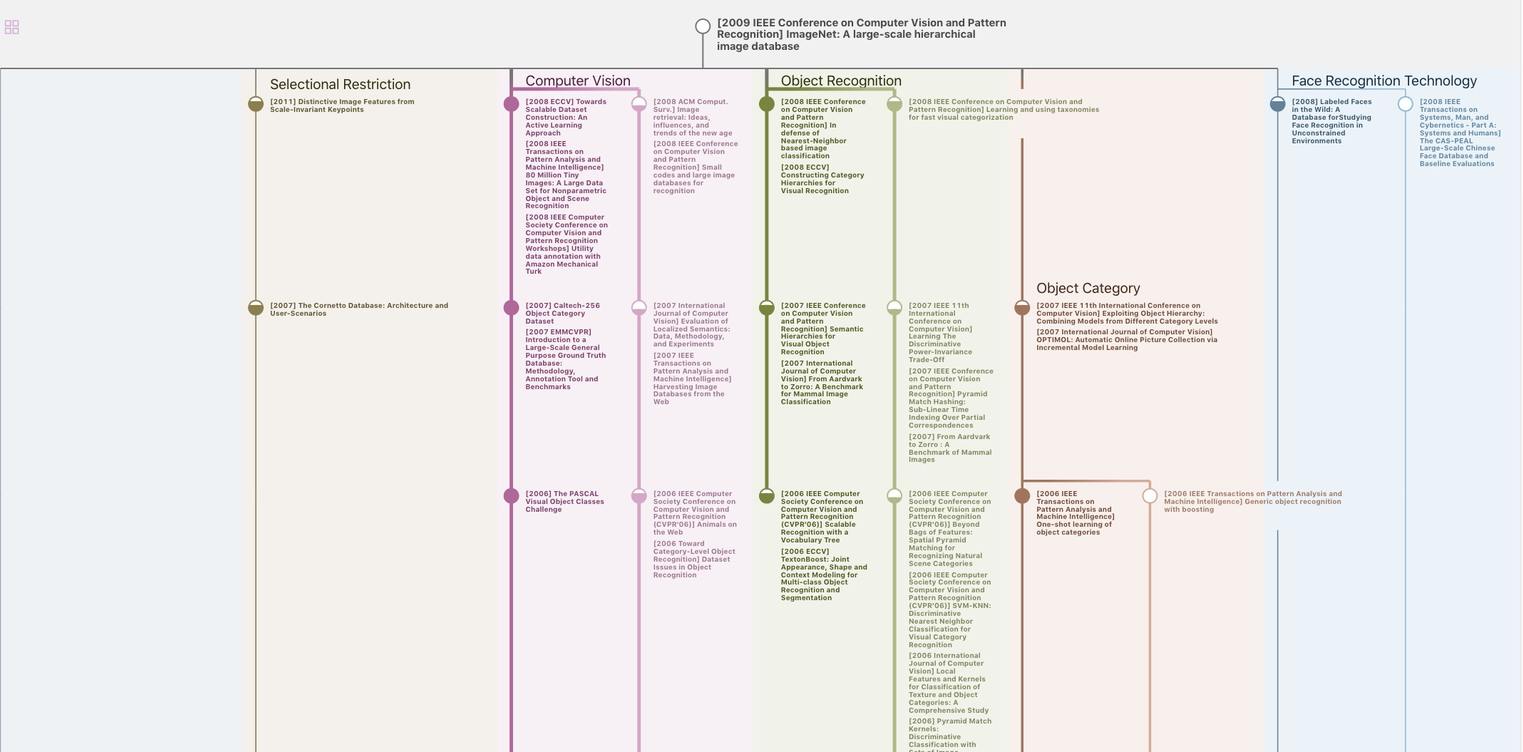
生成溯源树,研究论文发展脉络
Chat Paper
正在生成论文摘要