A Density-Guided Temporal Attention Transformer for Indiscernible Object Counting in Underwater Video
IEEE International Conference on Acoustics, Speech, and Signal Processing(2024)
摘要
Dense object counting or crowd counting has come a long way thanks to therecent development in the vision community. However, indiscernible objectcounting, which aims to count the number of targets that are blended withrespect to their surroundings, has been a challenge. Image-based objectcounting datasets have been the mainstream of the current publicly availabledatasets. Therefore, we propose a large-scale dataset called YoutubeFish-35,which contains a total of 35 sequences of high-definition videos with highframe-per-second and more than 150,000 annotated center points across aselected variety of scenes. For benchmarking purposes, we select threemainstream methods for dense object counting and carefully evaluate them on thenewly collected dataset. We propose TransVidCount, a new strong baseline thatcombines density and regression branches along the temporal domain in a unifiedframework and can effectively tackle indiscernible object counting withstate-of-the-art performance on YoutubeFish-35 dataset.
更多查看译文
AI 理解论文
溯源树
样例
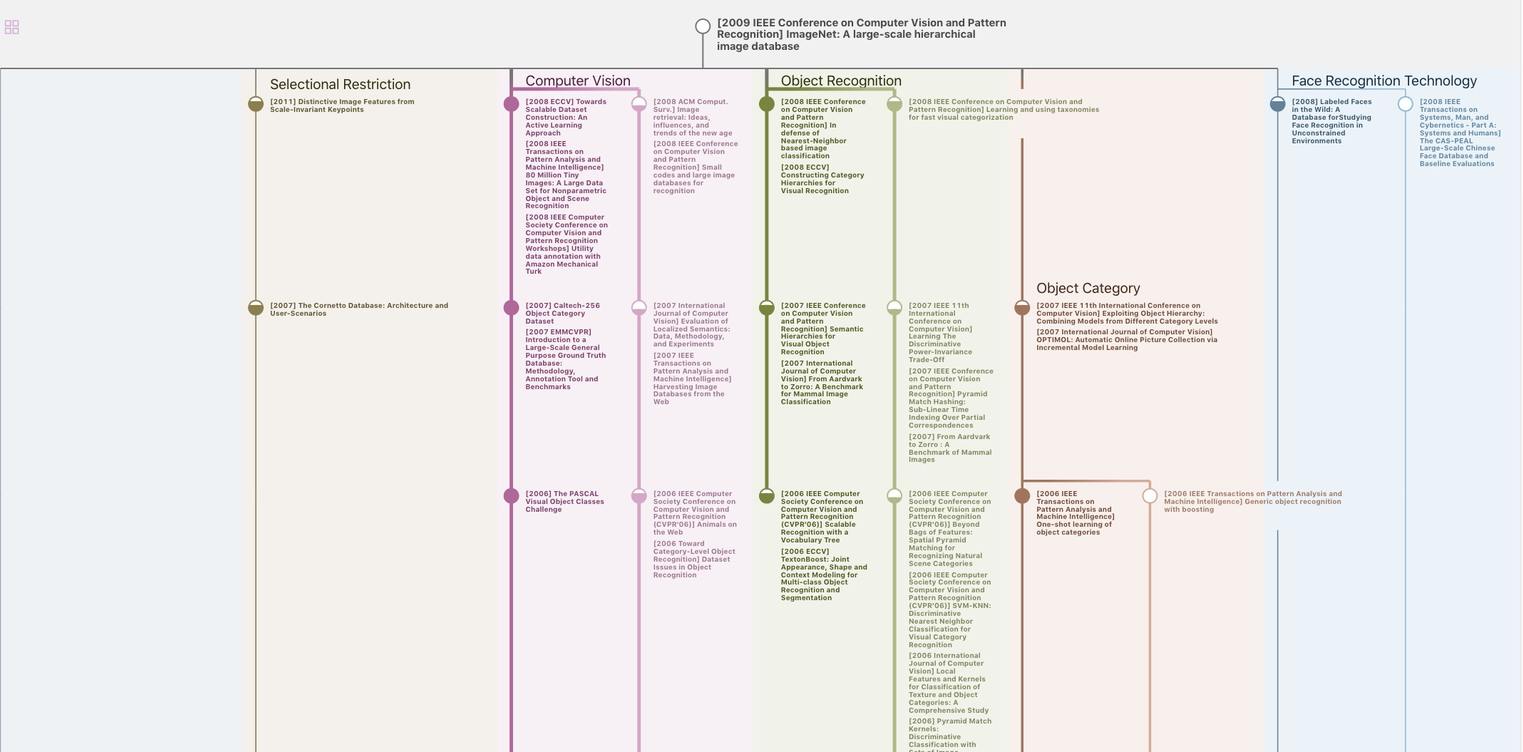
生成溯源树,研究论文发展脉络
Chat Paper
正在生成论文摘要