NPSV-deep: a deep learning method for genotyping structural variants in short read genome sequencing data
BIOINFORMATICS(2024)
摘要
Motivation Structural variants (SVs) play a causal role in numerous diseases but can be difficult to detect and accurately genotype (determine zygosity) with short-read genome sequencing data (SRS). Improving SV genotyping accuracy in SRS data, particularly for the many SVs first detected with long-read sequencing, will improve our understanding of genetic variation.Results NPSV-deep is a deep learning-based approach for genotyping previously reported insertion and deletion SVs that recasts this task as an image similarity problem. NPSV-deep predicts the SV genotype based on the similarity between pileup images generated from the actual SRS data and matching SRS simulations. We show that NPSV-deep consistently matches or improves upon the state-of-the-art for SV genotyping accuracy across different SV call sets, samples and variant types, including a 25% reduction in genotyping errors for the Genome-in-a-Bottle (GIAB) high-confidence SVs. NPSV-deep is not limited to the SVs as described; it improves deletion genotyping concordance a further 1.5 percentage points for GIAB SVs (92%) by automatically correcting imprecise/incorrectly described SVs.Availability and implementation Python/C++ source code and pre-trained models freely available at https://github.com/mlinderm/npsv2.
更多查看译文
AI 理解论文
溯源树
样例
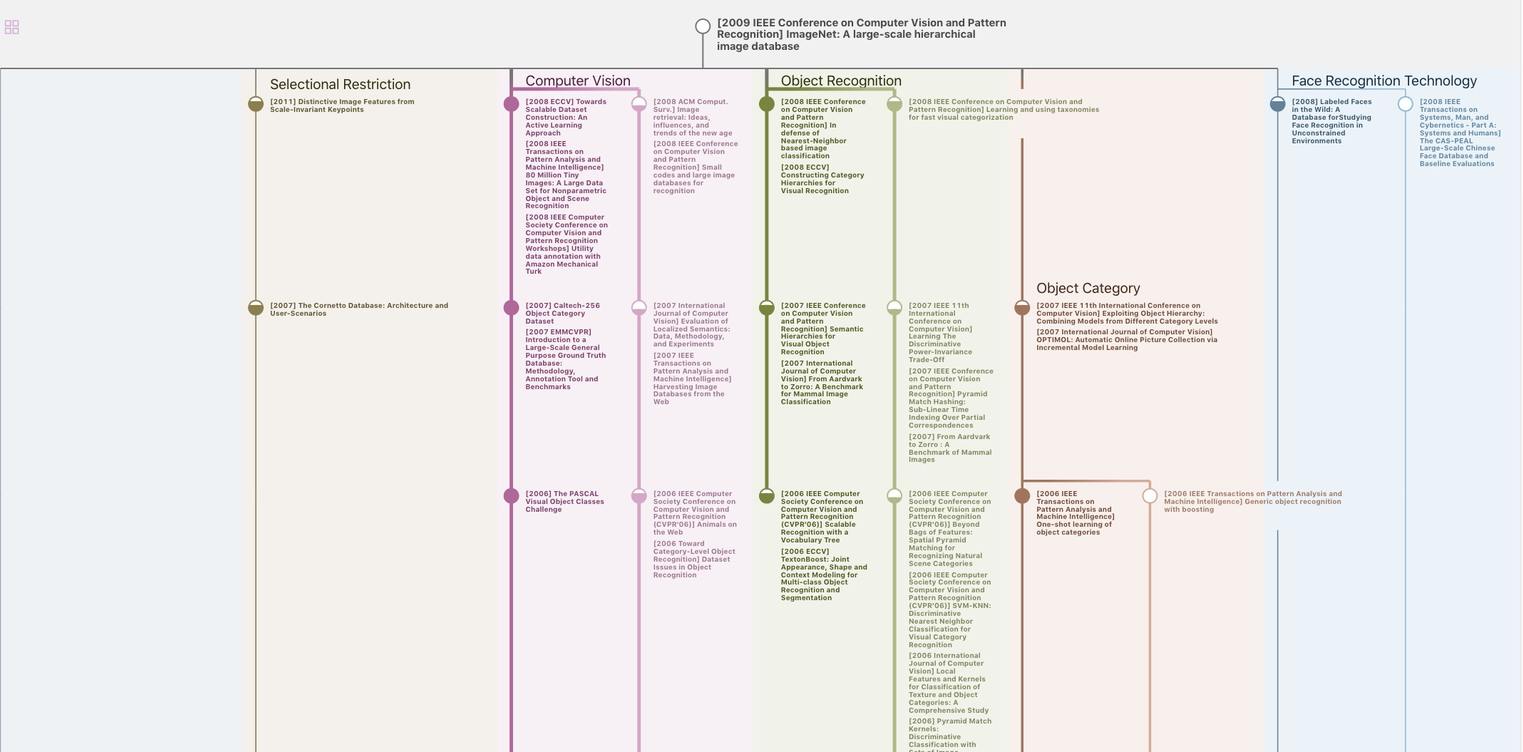
生成溯源树,研究论文发展脉络
Chat Paper
正在生成论文摘要