Alcoholism Detection in EEG Signals using GLCM-Based Texture Analysis of Image-Converted Signals
2023 6th International Conference on Information and Communications Technology (ICOIACT)(2023)
Abstract
Alcoholism is a prevalent and serious global issue, causing detrimental effects on individuals’ physical and mental health, social relationships, and productivity. The World Health Organization (WHO) reports that harmful alcohol consumption leads to approximately 3 million deaths annually, accounting for 5.3% of all global deaths. Moreover, alcohol abuse contributes significantly to various health problems, including liver cirrhosis, cancers, and mental disorders. In light of this, efficient methods for detecting alcohol-related disorders are essential for early intervention and effective treatment. This study proposes a feature extraction technique using GLCM (Gray-Level Co-occurrence Matrix) texture analysis to classify normal and alcoholic EEG signals. The process involves forming a matrix of 64 signal channels from EEG data, and GLCM features such as ASM, IDM, Correlation, Contrast, Variance, and entropy are extracted at different angles. The study achieves a 70% accuracy using Random Forest with all features and directions. Although this accuracy may be lower than other similar studies, the proposed method shows potential for improvement, such as exploring optimal distances or selecting appropriate features. Additionally, the utilization of various GLCM features and other feature extraction methods presents exciting prospects for future research in this domain.
MoreTranslated text
Key words
Alcoholism,EEG,GLCM,Random Forest
AI Read Science
Must-Reading Tree
Example
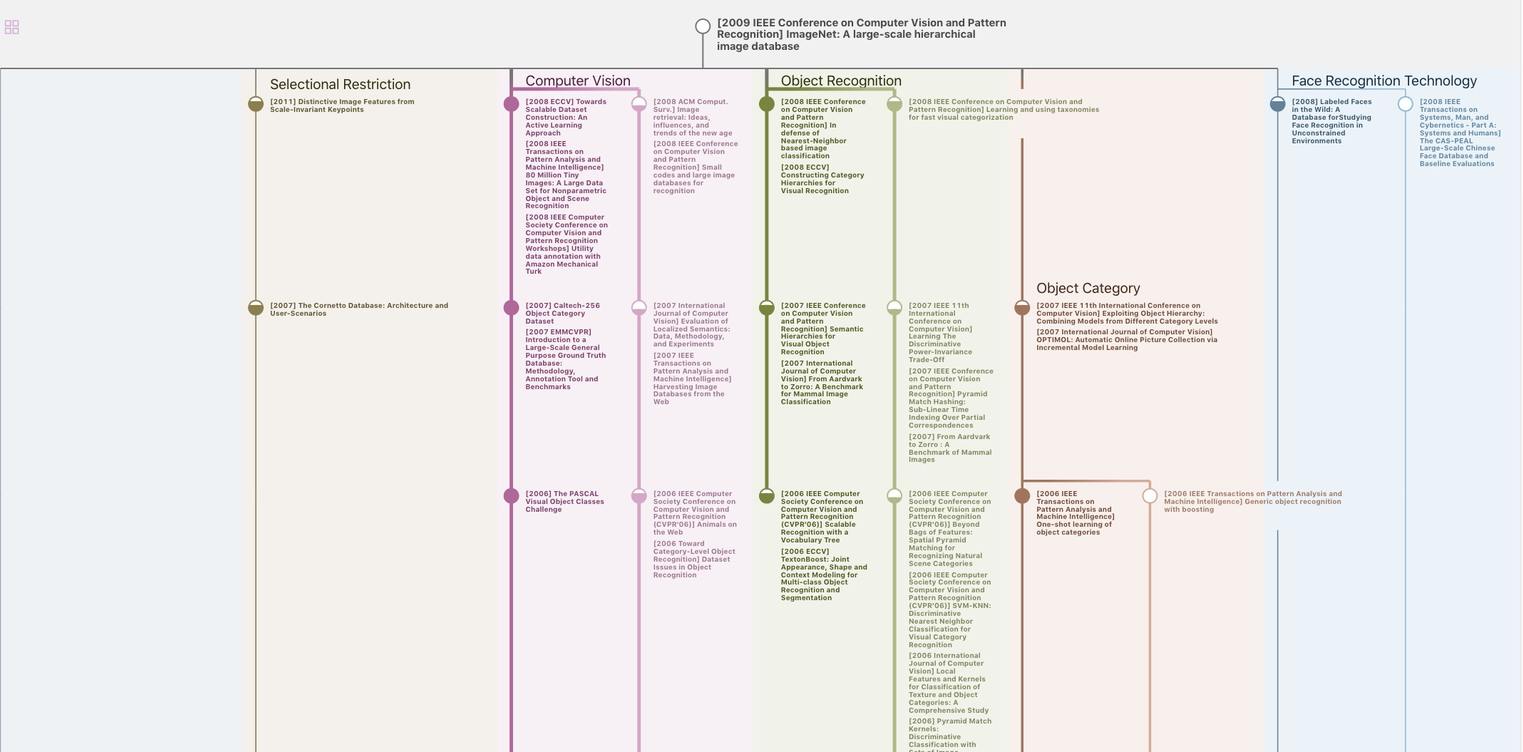
Generate MRT to find the research sequence of this paper
Chat Paper
Summary is being generated by the instructions you defined