Biomass Higher Heating Value Prediction Machine Learning Insights into Ultimate, Proximate, and Structural Analysis Datasets
ENERGY SOURCES PART A-RECOVERY UTILIZATION AND ENVIRONMENTAL EFFECTS(2024)
摘要
In this study machine learning (ML) models have been employed to predict the higher heating value (HHV) of biomass by utilizing input variables derived from ultimate, proximate, and structural analyses. In total, 180 models were developed, with 124 utilizing ultimate analysis data, 28 based on proximate analysis, and 28 relying on structural analysis. Various ML techniques, including polynomial models (SOP), support vector machines (SVM), random forest regression (RFR), and artificial neural networks (ANN), were employed for analysis. The study found that ANN models, when '' fed '' with FC and VM data, provided considerable accuracy in prediction results, with the best results obtained with 2-12-1 architecture (R2 = 0.96). In addition, a separate model configuration that processed inputs on biomass constituents such as cellulose, lignin, and hemicellulose showed remarkable agreement with empirical data. Additional findings revealed that the models created using SOP (R2 = 0.95), SVM (R2 = 0.95), and RFR (R2 = 0.90) demonstrated minimal discrepancies when predicting HHV. This study provides significant insights into the investigation of biomass analysis techniques employing ML tools, paving the way for future research aimed at constructing a robust tool for HHV prediction. Subsequent models may explore integrating inputs from diverse analysis methods and leveraging advanced machine learning techniques to enhance accuracy further.
更多查看译文
关键词
Energy properties,mathematical modeling,ultimate analysis,proximate analysis,structural analysis
AI 理解论文
溯源树
样例
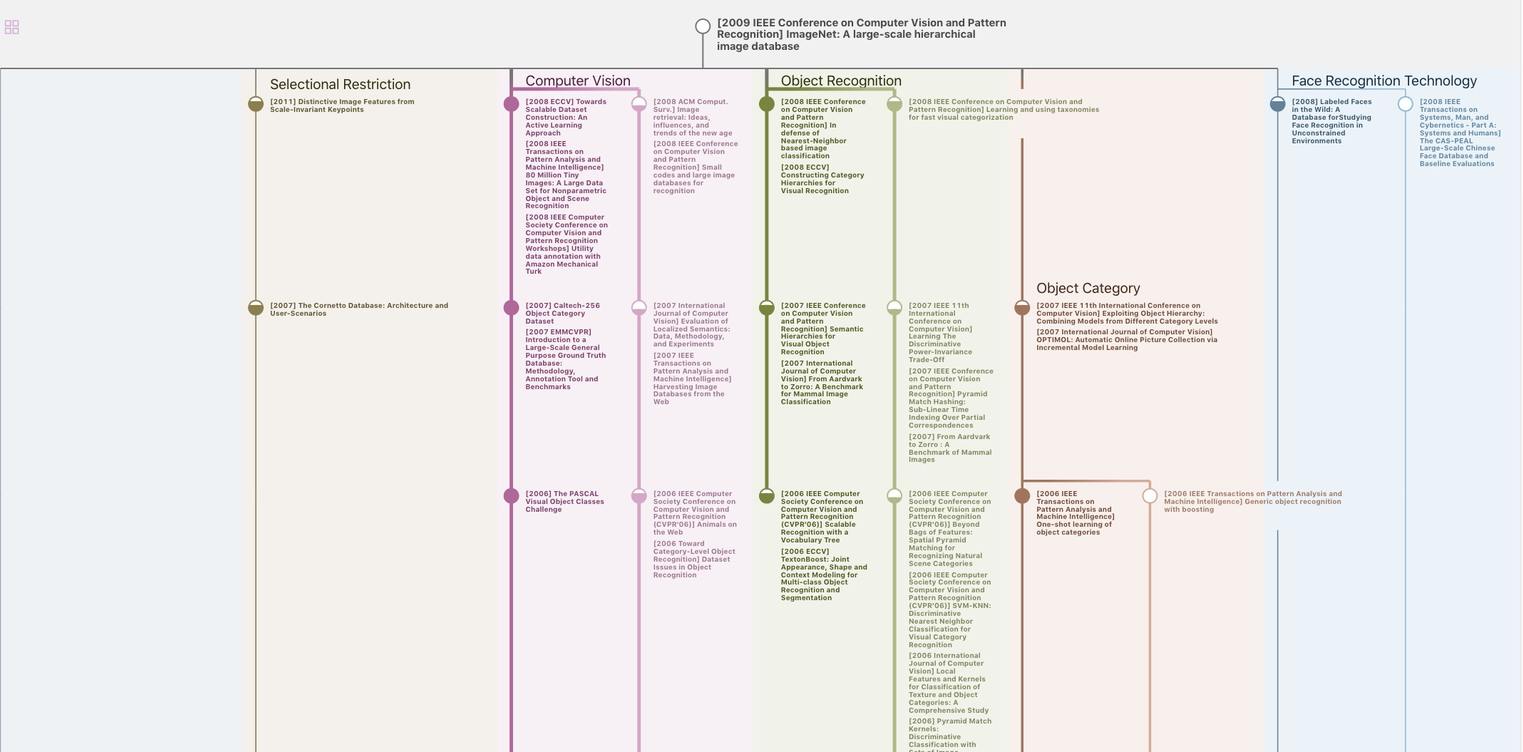
生成溯源树,研究论文发展脉络
Chat Paper
正在生成论文摘要