ASDNet: A Robust Involution‐based Architecture for Diagnosis of Autism Spectrum Disorder Utilising Eye‐tracking Technology
IET COMPUTER VISION(2024)
关键词
autism spectrum disorder,convolutional neural network,eye tracking,involutional neural network,Monte Carlo Dropout,uncertainty analysis
AI 理解论文
溯源树
样例
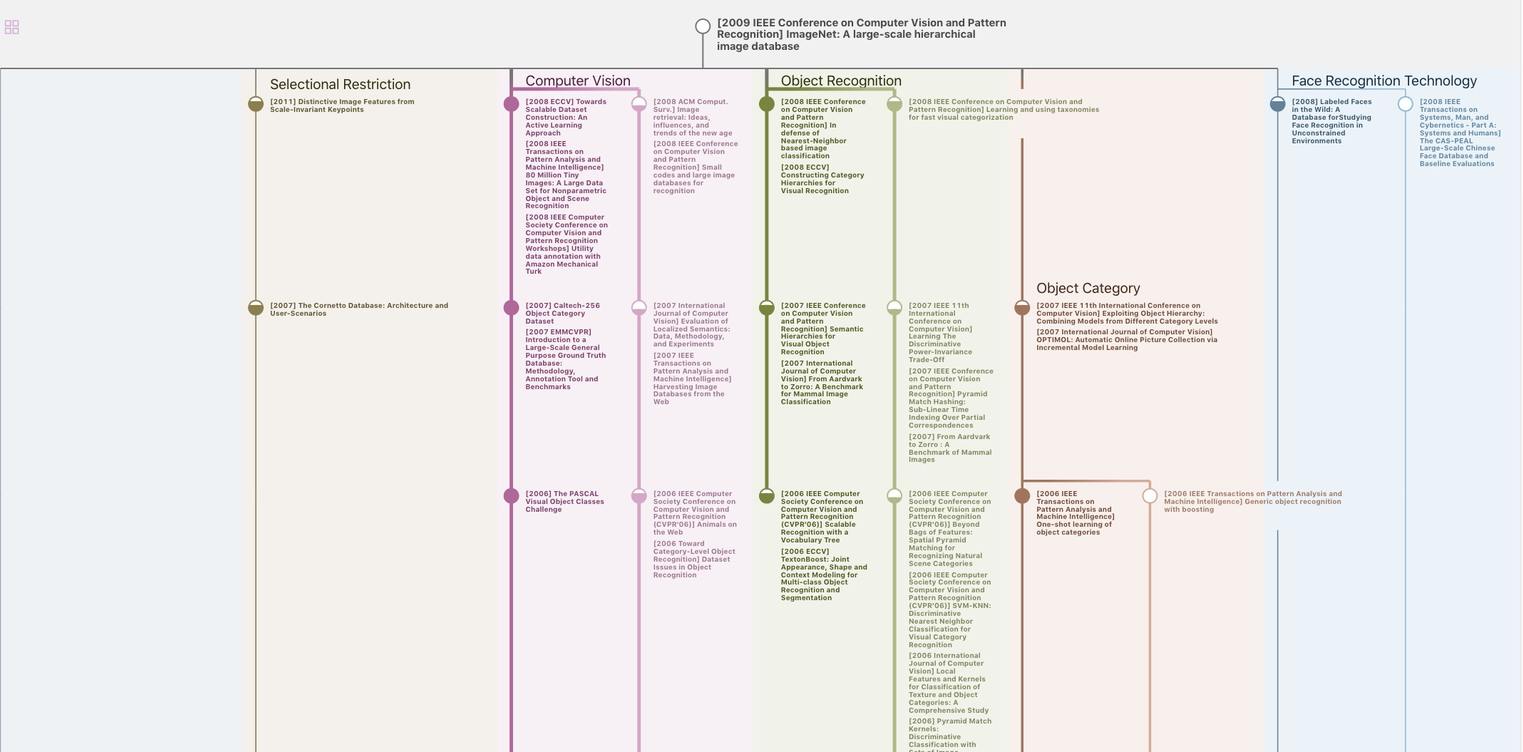
生成溯源树,研究论文发展脉络
Chat Paper
正在生成论文摘要