AdaTT: Adaptive Task-to-Task Fusion Network for Multitask Learning in Recommendations
KDD 2023(2023)
摘要
Multi-task learning (MTL) aims to enhance the performance and efficiency of machine learning models by simultaneously training them on multiple tasks. However, MTL research faces two challenges: 1) effectively modeling the relationships between tasks to enable knowledge sharing, and 2) jointly learning task-specific and shared knowledge. In this paper, we present a novel model called Adaptive Task-to-Task Fusion Network (AdaTT)(1) to address both challenges. AdaTT is a deep fusion network built with task-specific and optional shared fusion units at multiple levels. By leveraging a residual mechanism and a gating mechanism for task-to-task fusion, these units adaptively learn both shared knowledge and task-specific knowledge. To evaluate AdaTT's performance, we conduct experiments on a public benchmark and an industrial recommendation dataset using various task groups. Results demonstrate AdaTT significantly outperforms existing state-of-the-art baselines. Furthermore, our end-to-end experiments reveal that the model exhibits better performance compared to alternatives.
更多查看译文
关键词
multi-task learning,neural network,recommender systems
AI 理解论文
溯源树
样例
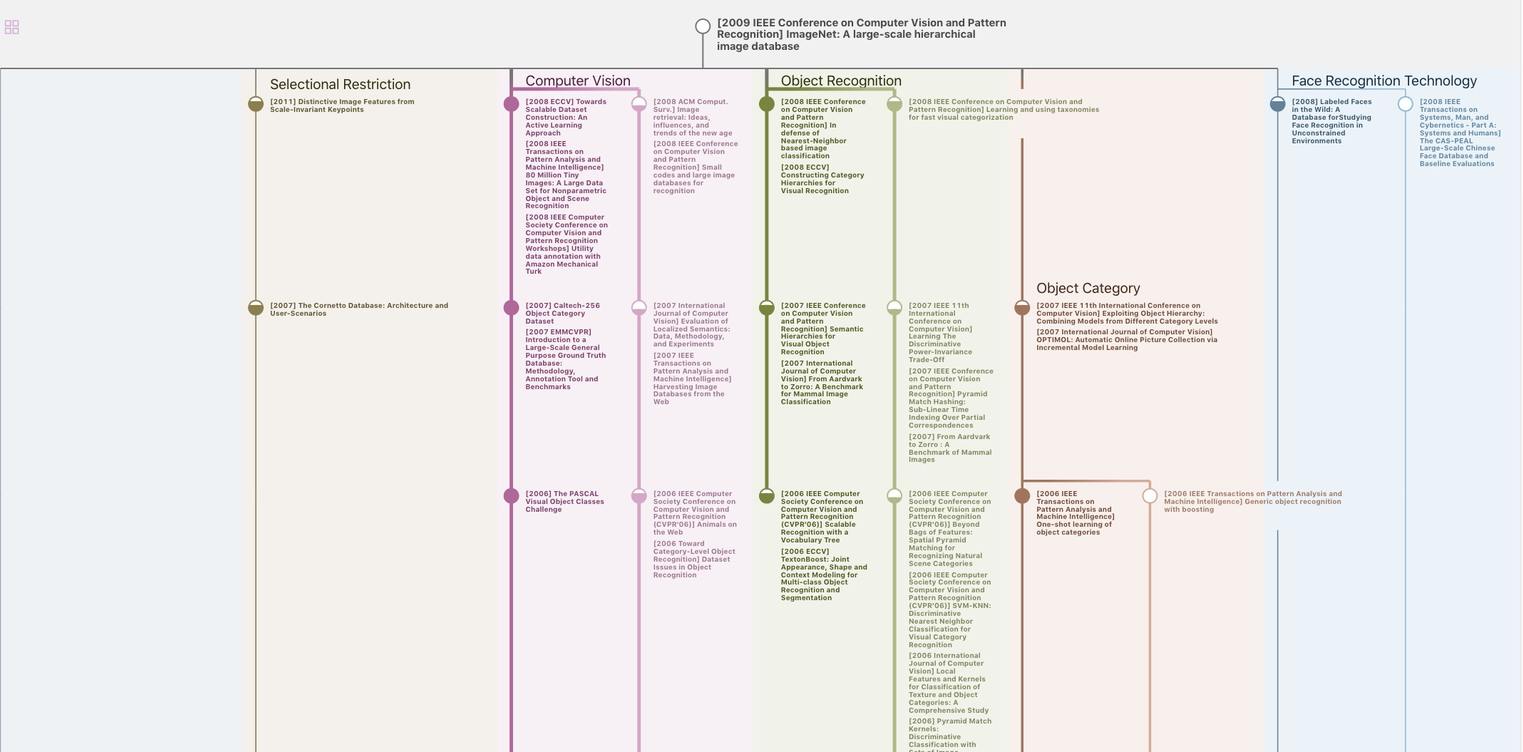
生成溯源树,研究论文发展脉络
Chat Paper
正在生成论文摘要