A Sampling-Based Approach to Urban Motion Planning Games with Stochastic Dynamics
IEEE Transactions on Intelligent Vehicles(2024)
摘要
Urban driving is a challenging task that requires autonomous agents to account for the stochastic dynamics and interactions with other vehicles. In this paper, we propose a novel framework that models urban driving as a stochastic generalized Nash equilibrium problem (SGNEP) and solves it using information-theoretic model predictive control (IT-MPC). By exploiting the cooperative nature of urban driving, we transform the SGNEP into a stochastic potential game (SPG), which has desirable convergence guarantees. Furthermore, we provide an algorithm for isolating interacting vehicles and thus factorizing a game into multiple sub-games. Finally, we solve for the open-loop generalized Nash equilibrium of a stochastic game utilizing a sampling-based technique. We solve the problem in a receding-horizon fashion, and apply our framework to various urban scenarios, such as intersections, lane merges, and ramp merges, and show that it can achieve safe and efficient multi-agent navigation.
更多查看译文
关键词
Intelligent vehicles,Cooperative autonomous driving,Motion planning,Vehicle dynamics and control,Control and optimization
AI 理解论文
溯源树
样例
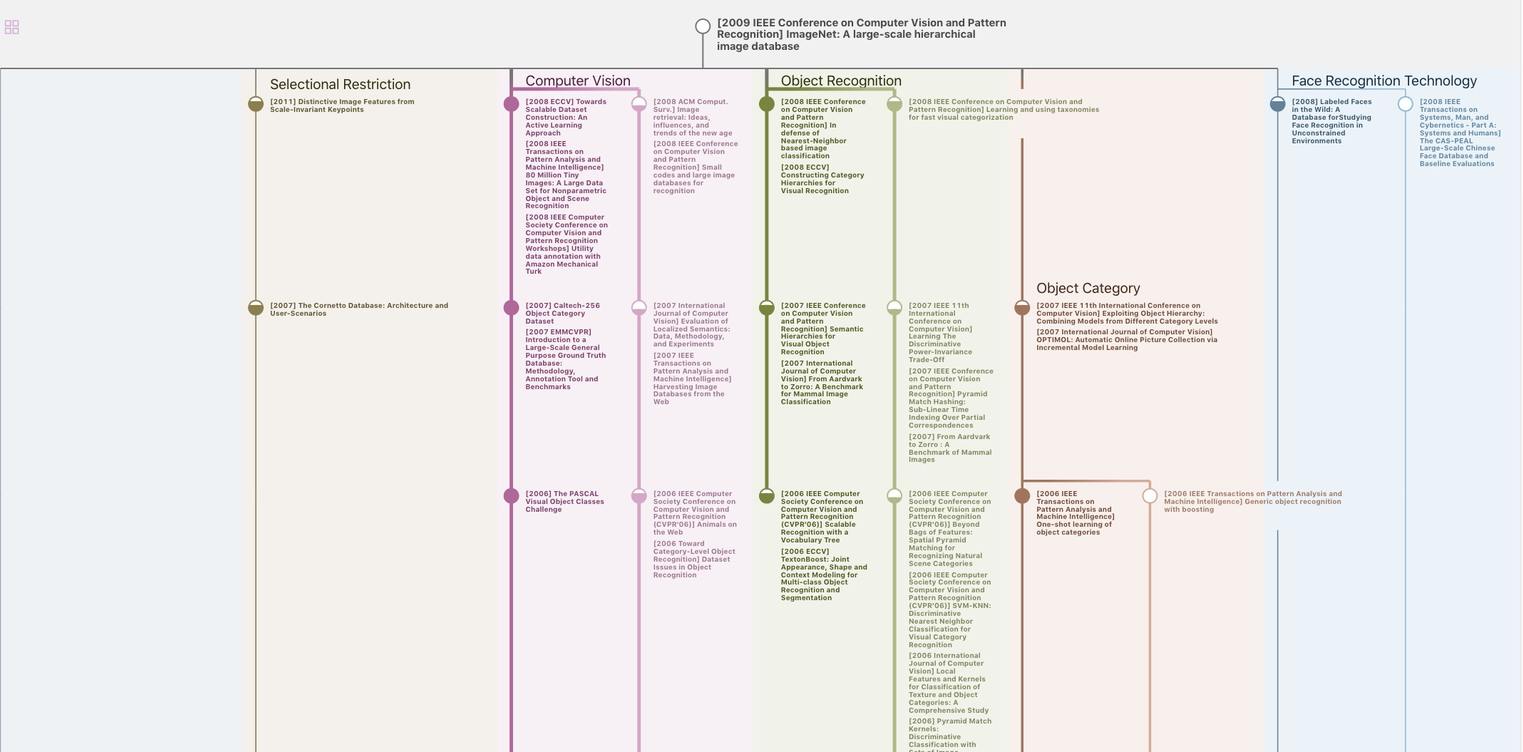
生成溯源树,研究论文发展脉络
Chat Paper
正在生成论文摘要