Exposing the Deception: Uncovering More Forgery Clues for Deepfake Detection
AAAI 2024(2024)
摘要
Deepfake technology has given rise to a spectrum of novel and compelling applications. Unfortunately, the widespread proliferation of high-fidelity fake videos has led to pervasive confusion and deception, shattering our faith that seeing is believing. One aspect that has been overlooked so far is that current deepfake detection approaches may easily fall into the trap of overfitting, focusing only on forgery clues within one or a few local regions. Moreover, existing works heavily rely on neural networks to extract forgery features, lacking theoretical constraints guaranteeing that sufficient forgery clues are extracted and superfluous features are eliminated. These deficiencies culminate in unsatisfactory accuracy and limited generalizability in real-life scenarios.
In this paper, we try to tackle these challenges through three designs: (1) We present a novel framework to capture broader forgery clues by extracting multiple non-overlapping local representations and fusing them into a global semantic-rich feature. (2) Based on the information bottleneck theory, we derive Local Information Loss to guarantee the orthogonality of local representations while preserving comprehensive task-relevant information. (3) Further, to fuse the local representations and remove task-irrelevant information, we arrive at a Global Information Loss through the theoretical analysis of mutual information. Empirically, our method achieves state-of-the-art performance on five benchmark datasets. Our code is available at https://github.com/QingyuLiu/Exposing-the-Deception, hoping to inspire researchers.
更多查看译文
关键词
CV: Computational Photography, Image & Video Synthesis,CV: Biometrics, Face, Gesture & Pose,CV: Representation Learning for Vision
AI 理解论文
溯源树
样例
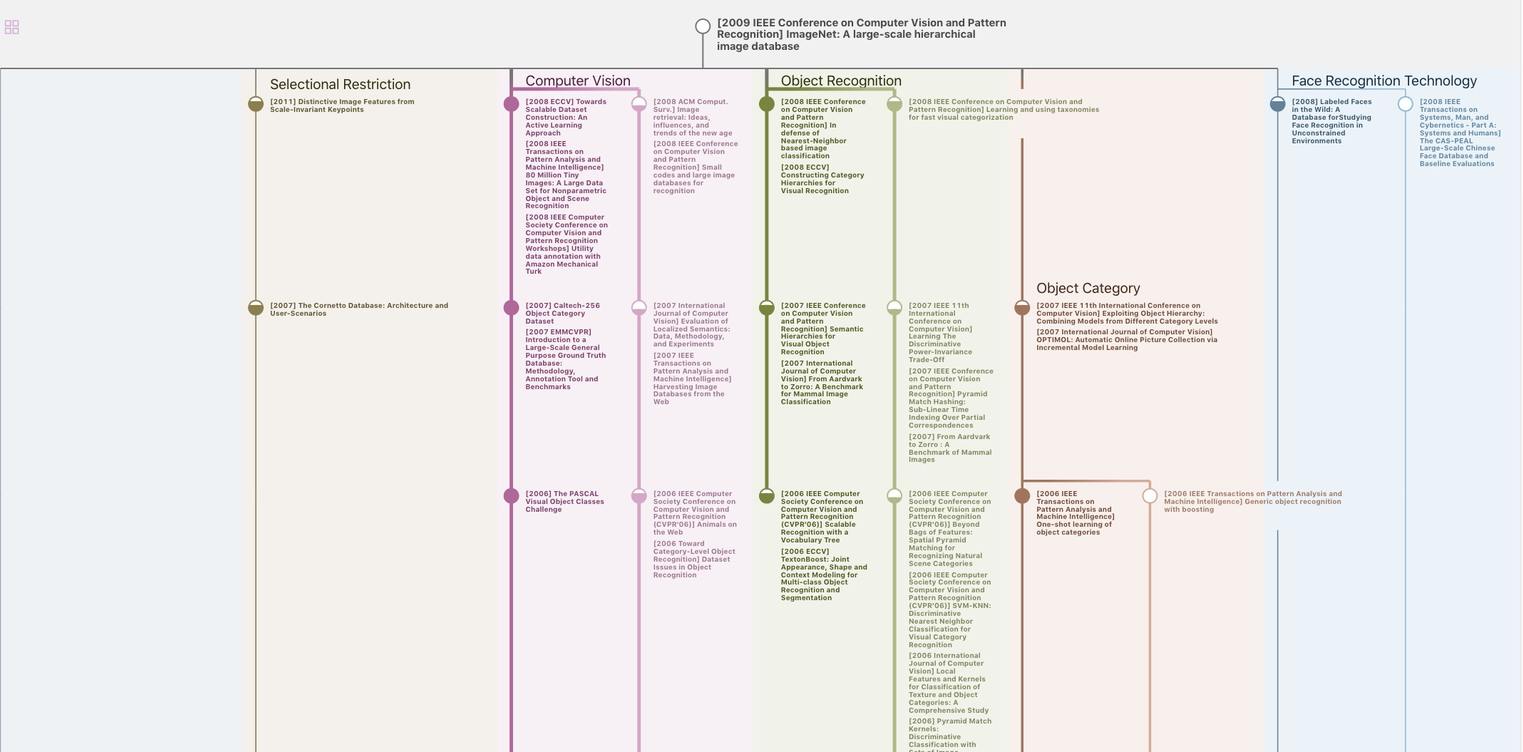
生成溯源树,研究论文发展脉络
Chat Paper
正在生成论文摘要