Answerability in Retrieval-Augmented Open-Domain Question Answering
CoRR(2024)
摘要
The performance of Open-Domain Question Answering (ODQA) retrieval systems
can exhibit sub-optimal behavior, providing text excerpts with varying degrees
of irrelevance. Unfortunately, many existing ODQA datasets lack examples
specifically targeting the identification of irrelevant text excerpts. Previous
attempts to address this gap have relied on a simplistic approach of pairing
questions with random text excerpts. This paper aims to investigate the
effectiveness of models trained using this randomized strategy, uncovering an
important limitation in their ability to generalize to irrelevant text excerpts
with high semantic overlap. As a result, we observed a substantial decrease in
predictive accuracy, from 98
an efficient approach for training models to recognize such excerpts. By
leveraging unanswerable pairs from the SQuAD 2.0 dataset, our models achieve a
nearly perfect ( 100
excerpts.
更多查看译文
AI 理解论文
溯源树
样例
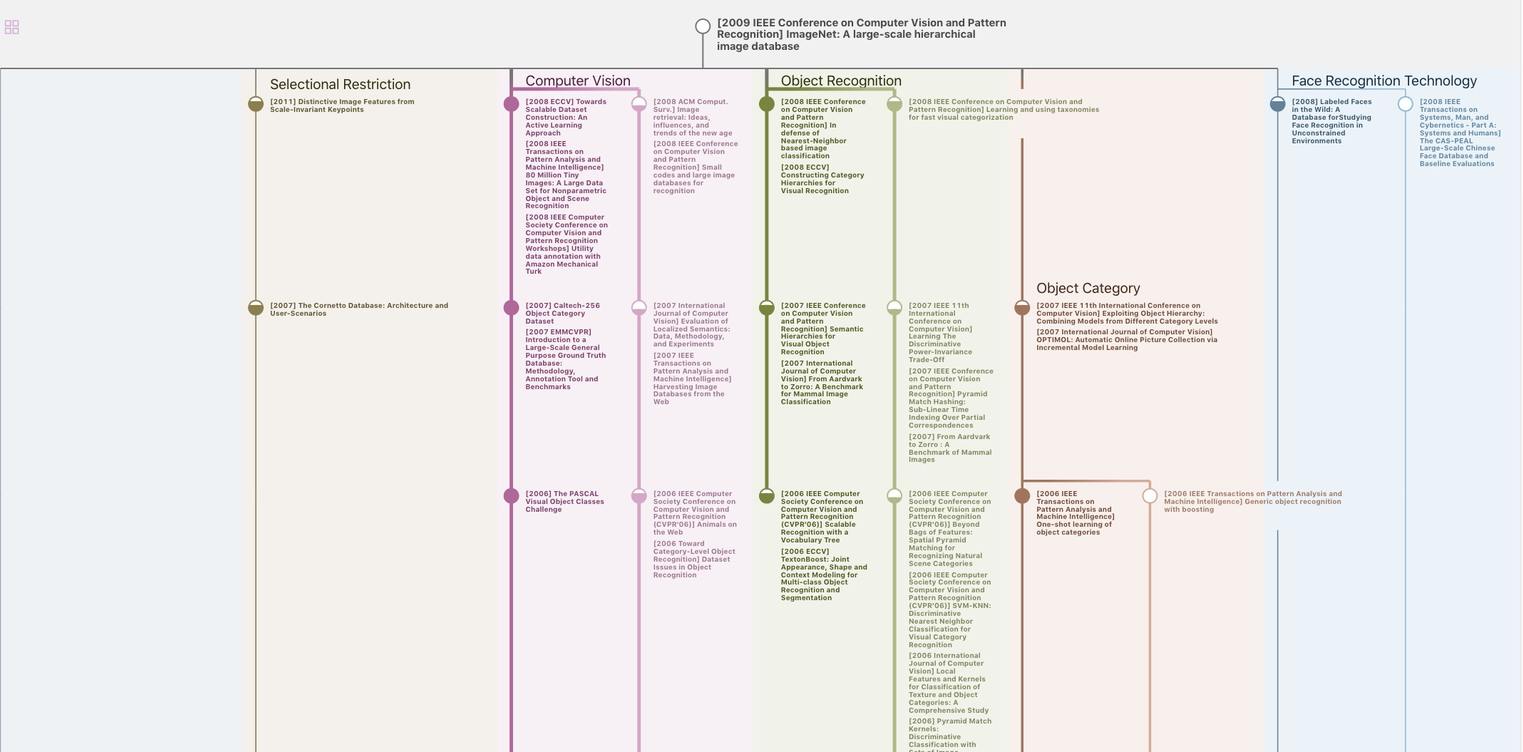
生成溯源树,研究论文发展脉络
Chat Paper
正在生成论文摘要