Fine-Grained Quantitative Emotion Editing for Speech Generation
arXiv (Cornell University)(2024)
摘要
It remains a significant challenge how to quantitatively control theexpressiveness of speech emotion in speech generation. In this work, we presenta novel approach for manipulating the rendering of emotions for speechgeneration. We propose a hierarchical emotion distribution extractor, i.e.Hierarchical ED, that quantifies the intensity of emotions at different levelsof granularity. Support vector machines (SVMs) are employed to rank emotionintensity, resulting in a hierarchical emotional embedding. Hierarchical ED issubsequently integrated into the FastSpeech2 framework, guiding the model tolearn emotion intensity at phoneme, word, and utterance levels. Duringsynthesis, users can manually edit the emotional intensity of the generatedvoices. Both objective and subjective evaluations demonstrate the effectivenessof the proposed network in terms of fine-grained quantitative emotion editing.
更多查看译文
关键词
End-to-End Speech Recognition,Acoustic Modeling
AI 理解论文
溯源树
样例
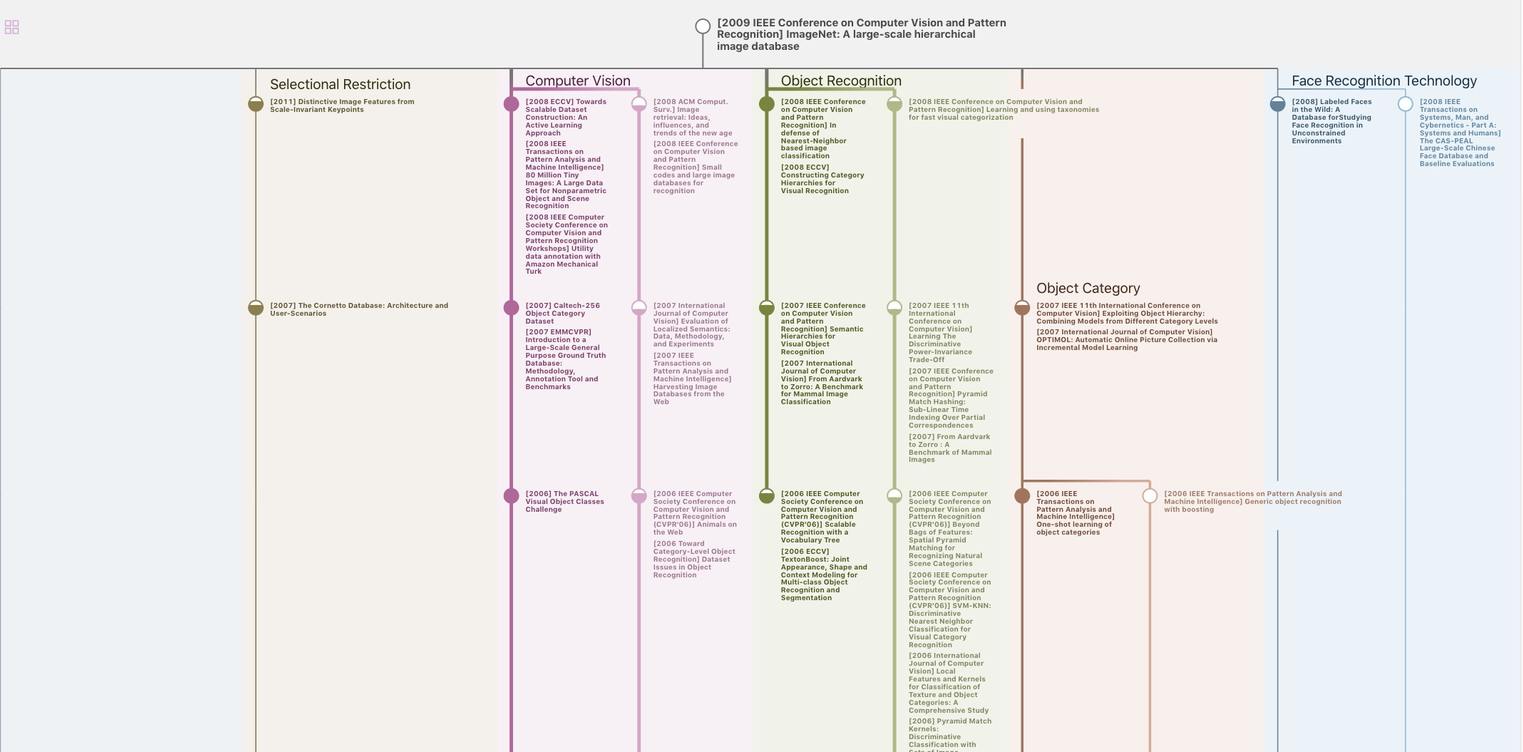
生成溯源树,研究论文发展脉络
Chat Paper
正在生成论文摘要