Chemical Transferability and Accuracy of Ionic Liquid Simulations with Machine Learning Interatomic Potentials
arxiv(2024)
摘要
Ionic liquids (ILs) are an exciting class of electrolytes finding
applications in many areas from energy storage to solvents, and have been
touted as “designer solvents”, as they can be mixed to precisely tailor the
physiochemical properties. As using machine learning interatomic potentials
(MLIPs) to simulate ILs is still relatively unexplored, several questions need
to be answered to see if MLIPs can be transformative for ILs. Since ILs are
often not pure, but are either mixed together or contain additives, we first
demonstrate that a MLIP can be trained to be chemically transferable, i.e., can
be applied to compositions not directly train on, but with similar chemistries,
whilst only being trained on a few compositions. We also investigate the
accuracy of MLIPs for a novel IL, which we experimentally synthesis and
characterise. Our MLIP trained on ∼200 DFT frames is in reasonable
agreement with our experiments and good agreement against DFT, demonstrating
the data-efficient power of this method.
更多查看译文
AI 理解论文
溯源树
样例
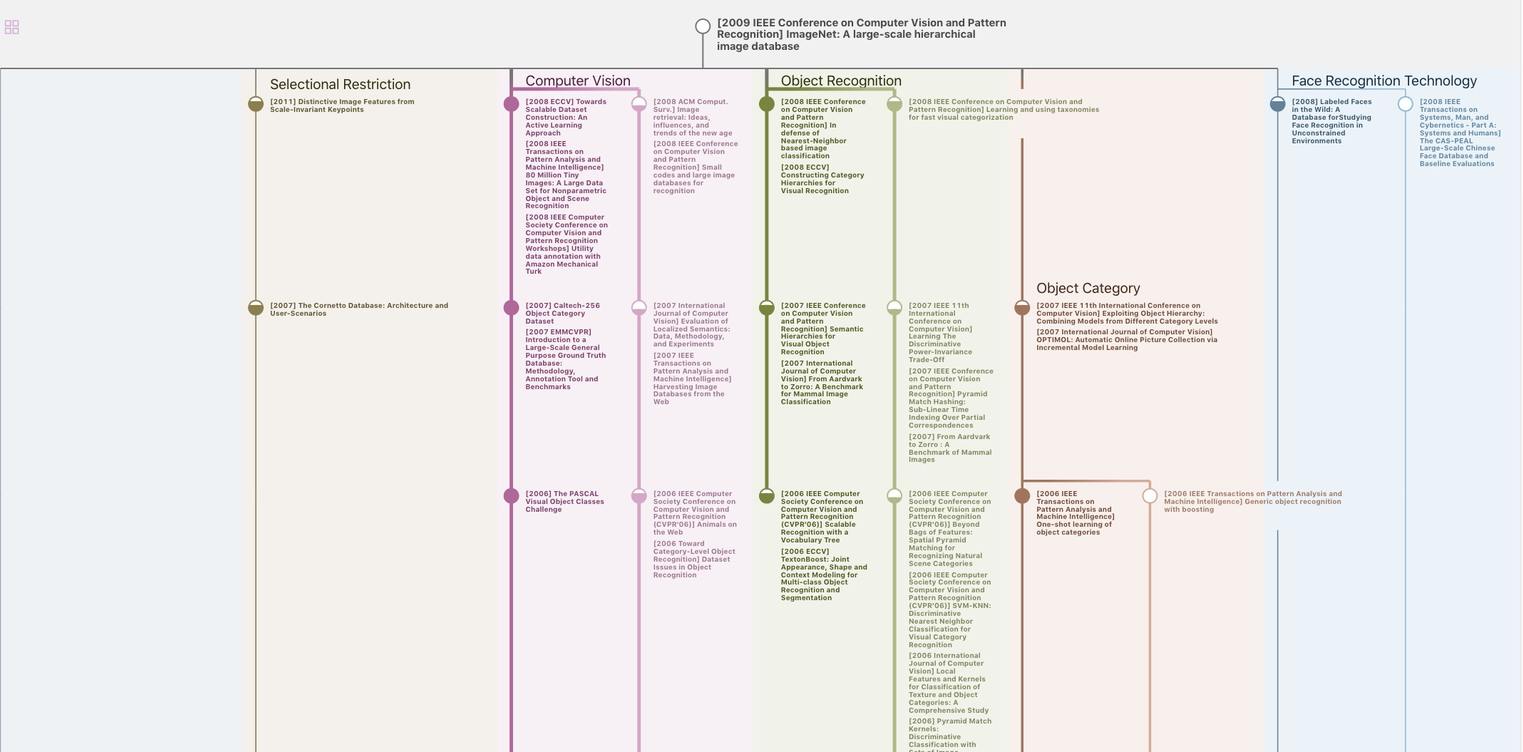
生成溯源树,研究论文发展脉络
Chat Paper
正在生成论文摘要