Effective Transferred Knowledge Identified by Bipartite Graph for Multiobjective Multitasking Optimization
Knowledge-Based Systems(2024)
摘要
Multiobjective Multitasking Optimization (MO-MTO) has become a hot research spot in the field of evolutionary computing. The fundamental problem of MO-MTO is to inhibit the negative transfer phenomenon. Mining the relationship among multiple optimization tasks and identifying the effective transferred knowledge have been proven a feasible way for the inhibition of negative transfer. In this paper, the solutions of different tasks are regarded as the vertices of two separate sets. Through constructing the bipartite graph of the vertices from these two sets, the relationship of different populations can be expressed and the valuable knowledge can be identified to transfer. Furthermore, a historical knowledge correction strategy is designed to deal with some special cases when identifying knowledge by the bipartite graph. A series of experiments are conducted on two MO-MTO test suits, and the results have demonstrated the efficacy of the proposed algorithm.
更多查看译文
关键词
Multiobjective optimization,Evolutionary multitasking,Bipartite graph,Knowledge transfer
AI 理解论文
溯源树
样例
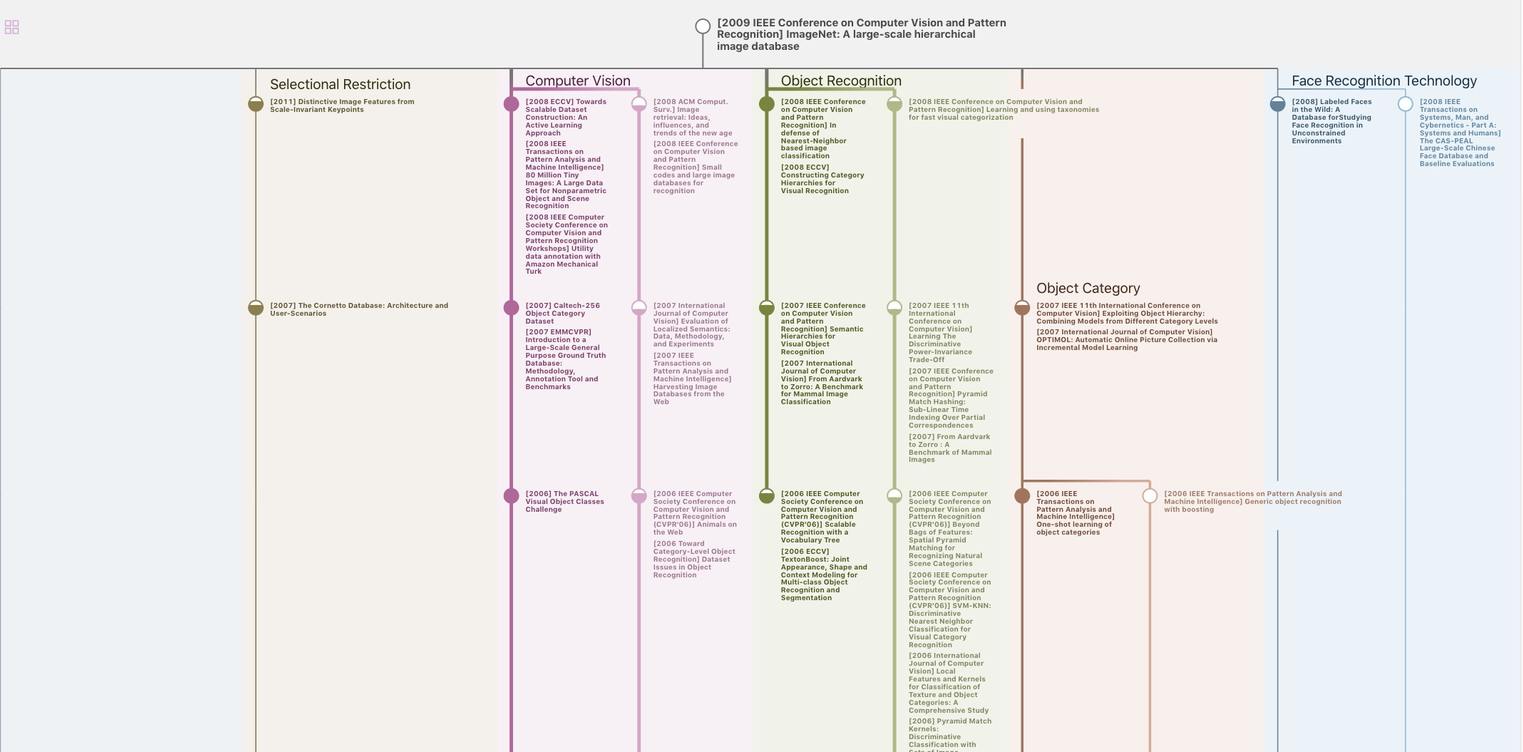
生成溯源树,研究论文发展脉络
Chat Paper
正在生成论文摘要