Towards the Development of a Citizens’ Science-Based Acoustic Rainfall Sensing System
Journal of Hydrology(2024)
Abstract
Floods have become more frequent and intense, causing human fatalities and economic losses worldwide. A robust rainfall estimation plays a pivotal role in flood forecasting and mitigation. It becomes even more critical in tropical urban areas, having rainfall events with high intensity and patchily distributed at local scales compared to rainfall in temperate climates. Therefore, measuring rainfall with high temporal and spatial resolutions is necessary. Unfortunately, several countries lack dense rainfall gauge networks and sufficient radar coverage. In this regard, several studies proposed incorporating citizens' science rainfall gauge networks to provide complementary daily data to existing rainfall sensing networks. However, limited to no efforts have been presented in the literature to develop new citizen science tools for event-based rainfall sensing or at a sub-daily level. Therefore, this study presents a proof of concept on utilising rainfall audio collected from a professional recorder, smartphone, or any other potential audio source for rainfall sensing in an urban area. The investigation is based on a dataset collected using a professional audio recorder at five different environments (locations) with various physical and acoustic characteristics over two years. The change in loudness levels as an input to a model was hypothesised to be a major acoustic feature that allows a model to distinguish rainfall intensities from each other at a specific location. However, using it alone would not be sufficient if the model deals with multiple locations in an urban area. Thus, a total of 40 acoustic features from different acoustic domains were examined and analysed to calibrate a machine-learning (ML) model to convert 1-min of rainfall audio recorded at different locations into 1-min of rainfall intensity. The research results showed that combining a loudness feature with complementary features from the cepstral and frequency domains could improve the model's performance on the validation dataset from R2 = 0.405 to R2 = 0.713. The developed ML model was able to generalise on a testing dataset collected both on-site and off-site (outside the five locations that were used in the calibration process). The model performance in estimating 5-min rainfall data on the on-site testing dataset reached an R2 = 0.893. Finally, the developed model was validated for estimating rainfall intensity using rainfall audio recorded by an Android smartphone. The results of this study present a promising step toward developing an acoustic rainfall sensing tool for citizen science applications; however, further investigation is necessary to upgrade this proof-of-concept study for practical applications.
MoreTranslated text
Key words
Rainfall sensing,Acoustic features,Machine learning techniques,Urban soundscape
AI Read Science
Must-Reading Tree
Example
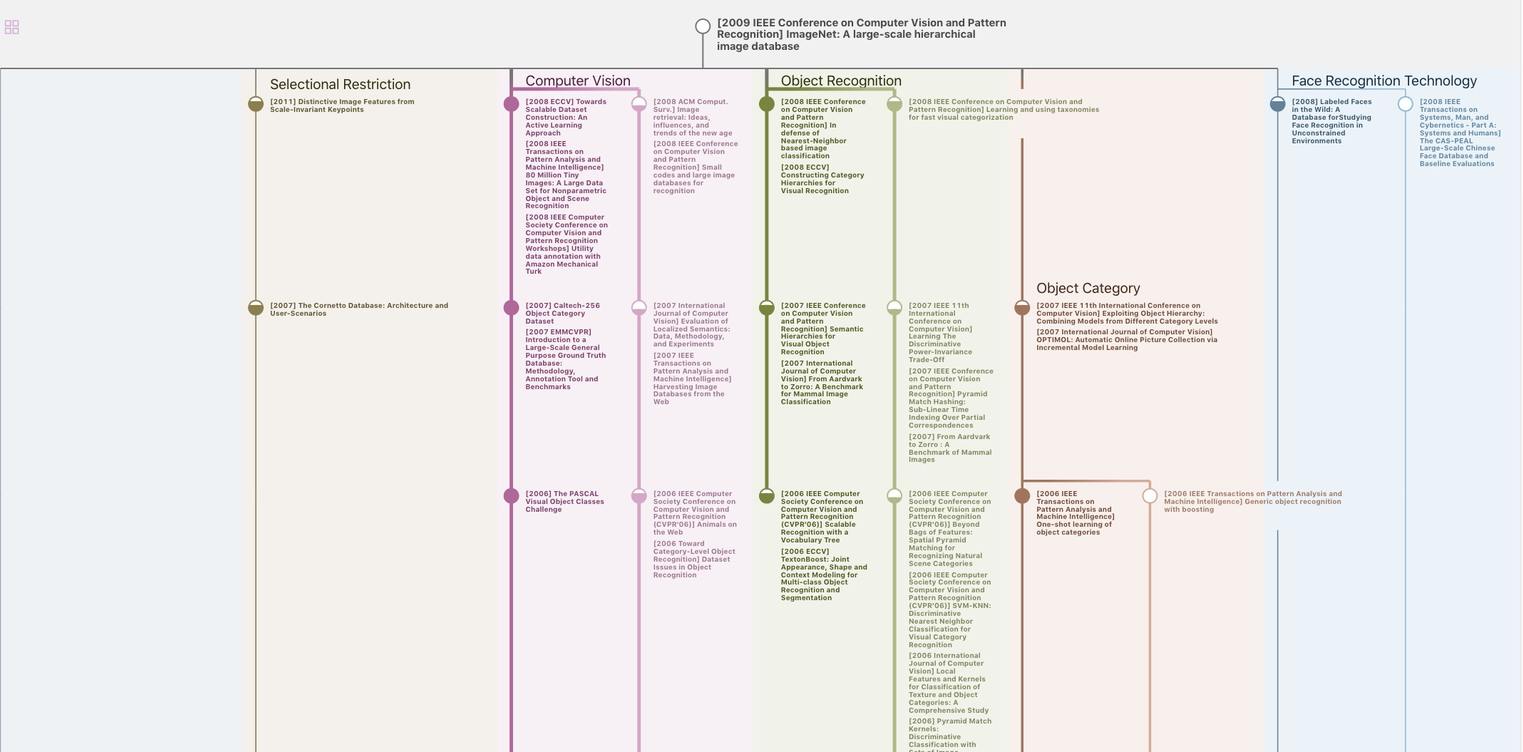
Generate MRT to find the research sequence of this paper
Chat Paper
Summary is being generated by the instructions you defined