A Diagnostic Algorithm Using Peanut SPT and Ara H 2 Sige Reduces Number of OFCs and Cost of Peanut Allergy Diagnosis in a Clinical Setting: a Cost Comparison Analysis.
Journal of Allergy and Clinical Immunology(2024)
Murdoch Childrens Res Inst | Univ Queensland
Abstract
Background: Peanut allergy prevalence is reported at 3% and consumes a significant volume of oral food challenges (OFC) in the diagnosis of food allergy. Compared to skin prick test (SPT) or sIgE to whole peanut, sIgE to peanut component ara h 2 has greater diagnostic accuracy. Two-step algorithms involving both SPT and ara h 2 sIgE demonstrate increased accuracy in diagnosis in modelled studies, resulting in fewer OFCs. We aimed to determine whether there is a similar reduction in OFCs in a clinical uptake of this two-step diagnostic algorithm compared to using peanut SPT alone and perform a cost comparison between these two approaches. Methods: In 2015, The Royal Children’s Hospital, Australia (RCH) implemented a clinical guideline using peanut SPT followed by sIgE to ara h 2 if the SPT was between 3 and 8mm and clinician is considering an OFC for a patient with suspected peanut allergy. We performed an audit of patients presenting to RCH allergy outpatient clinics for assessment of peanut allergy to determine the outcomes and potential reduction in need for OFC using the two-step algorithm. We used modelled data of the same patient cohort to estimate the number of OFCs that were avoided as a result of a high (≥1.0kUA/L) ara h 2 sIgE. A cost comparison was performed comparing the two-step algorithm to using peanut SPT alone. Costs were constructed based on unit prices from hospital admissions, medicare data and individual data on allergic reaction types, applying a health system perspective. Results: 8826 patients presented to RCH for peanut allergy assessment between May 2016 and August 2020. Of these, 9.2% proceeded to an OFC, with positive results seen in 20.1% and anaphylaxis in 1.1%. 42.0% (364/867) of patients with a SPT between 3-8mm followed the diagnostic algorithm appropriately. Of those who followed the diagnostic algorithm, there was a reduction of 27.8% in OFCs as a result of an elevated (>1.0kUA/L) ara h 2 result. The diagnostic algorithm pathway resulted in a 32.05% cost reduction compared to the modelled SPT-only pathway. Sensitivity analysis demonstrated that the number of patients proceeding to OFC gives greatest impact to the overall cost of diagnosis, rather than the cost of the OFC or ara h 2 itself. Conclusions: A combined algorithm incorporating peanut SPT followed by sIgE to ara h 2 resulted in a reduction in patients requiring oral food challenges. There is also a cost saving for this approach. There is a large proportion of patients that did not follow the algorithm, and this may relate to clinical history.
MoreTranslated text
求助PDF
上传PDF
View via Publisher
AI Read Science
AI Summary
AI Summary is the key point extracted automatically understanding the full text of the paper, including the background, methods, results, conclusions, icons and other key content, so that you can get the outline of the paper at a glance.
Example
Background
Key content
Introduction
Methods
Results
Related work
Fund
Key content
- Pretraining has recently greatly promoted the development of natural language processing (NLP)
- We show that M6 outperforms the baselines in multimodal downstream tasks, and the large M6 with 10 parameters can reach a better performance
- We propose a method called M6 that is able to process information of multiple modalities and perform both single-modal and cross-modal understanding and generation
- The model is scaled to large model with 10 billion parameters with sophisticated deployment, and the 10 -parameter M6-large is the largest pretrained model in Chinese
- Experimental results show that our proposed M6 outperforms the baseline in a number of downstream tasks concerning both single modality and multiple modalities We will continue the pretraining of extremely large models by increasing data to explore the limit of its performance
Upload PDF to Generate Summary
Must-Reading Tree
Example
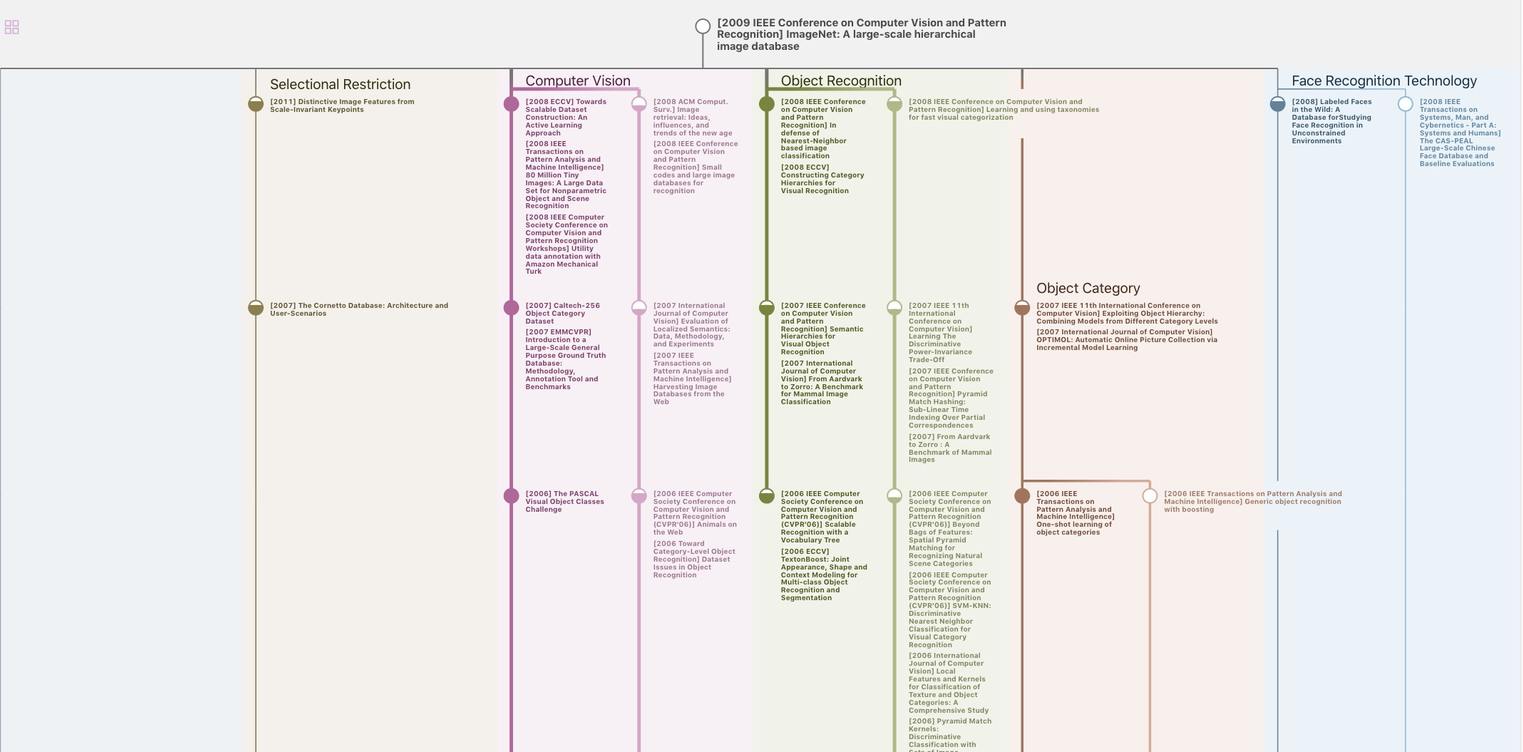
Generate MRT to find the research sequence of this paper
Data Disclaimer
The page data are from open Internet sources, cooperative publishers and automatic analysis results through AI technology. We do not make any commitments and guarantees for the validity, accuracy, correctness, reliability, completeness and timeliness of the page data. If you have any questions, please contact us by email: report@aminer.cn
Chat Paper