Bamboo classification based on GEDI, time-series Sentinel-2 images and whale-optimized, dual-channel DenseNet: A case study in Zhejiang province, China
ISPRS JOURNAL OF PHOTOGRAMMETRY AND REMOTE SENSING(2024)
摘要
Regional carbon sink estimation and local forest management require spatially explicit maps of bamboo distribution. However, accurate bamboo mapping is challenging due to the similarity of bamboo's optical spectral with those of other vegetation. To gain a high-precision bamboo forest distribution map circa year 2020, we developed a novel classification framework that integrated in-situ measurements, GEDI, and time-series multispectral Sentinel-2 images in a whale-optimized dual-channel Dense Convolutional Network (DenseNet) classification algorithm. The new method shows that vegetation structure features from GEDI can improve the performance of bamboo mapping by more than 5 percent compared with results obtained using only spectral information. Additionally, ablation studies show our proposed dual-channel DenseNet with whale-optimized DropBlock regularization outperforms traditional deep learning algorithms. The proposed method demonstrates a high level of accuracy in identifying bamboo, with precision, recall (producer's and user's accuracies), and F1-score reaching 90.81%, 91.86%, and 91.33%, respectively, which can provide more detailed and accurate results than previous studies, especially in regions where the terrain is complex or bamboo is intermixed with other vegetation.
更多查看译文
关键词
Bamboo,Land cover,Classification,GEDI,LiDAR,Sentinel-2
AI 理解论文
溯源树
样例
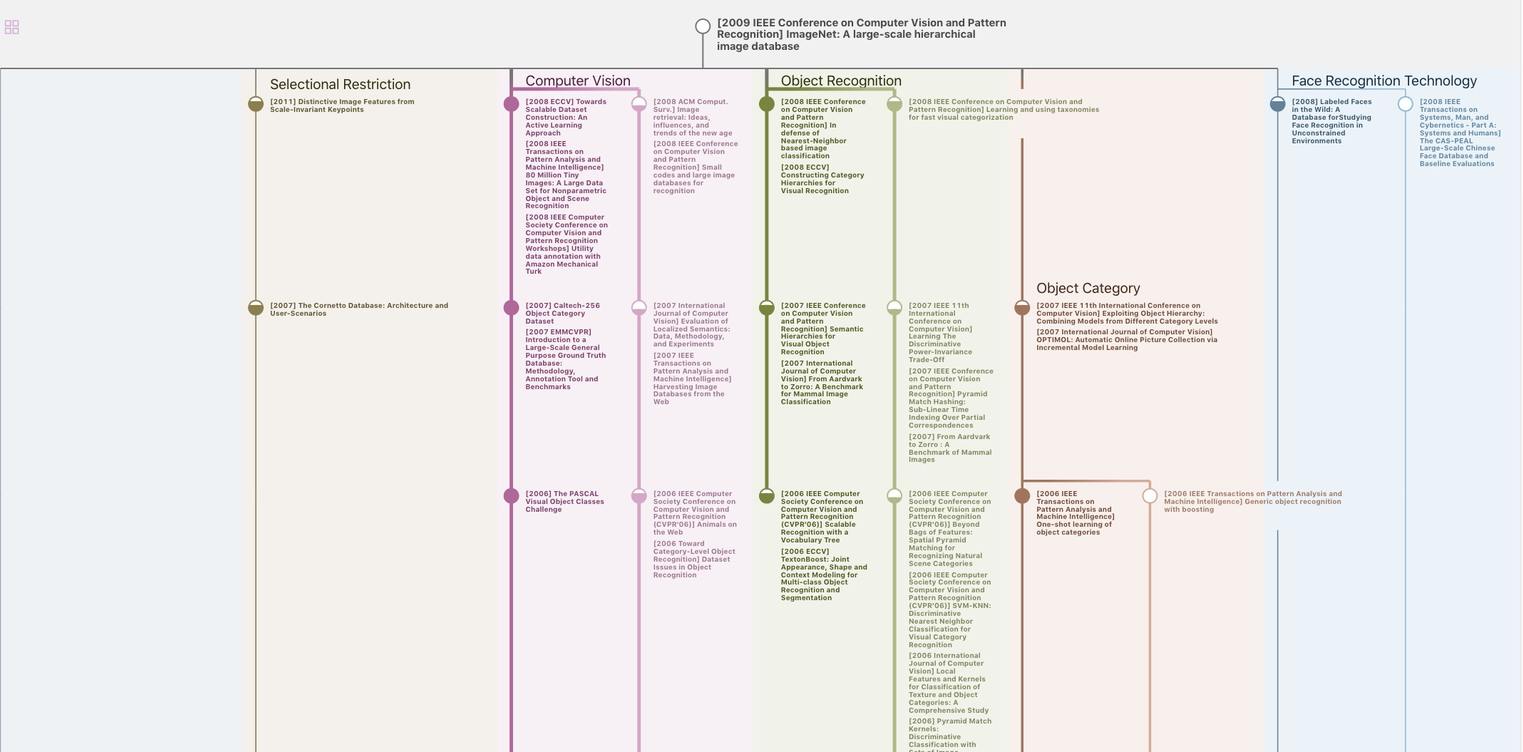
生成溯源树,研究论文发展脉络
Chat Paper
正在生成论文摘要