Improved camouflaged detection in the large-scale images and videos with minimum boundary contrast in detection technique
EXPERT SYSTEMS WITH APPLICATIONS(2024)
摘要
Recognizing camouflaged items in large-scale images or movies is a complex challenge requiring close attention to detail present here. These items have no discernible edges and are blended with the surrounding pixels. This solution makes use of the high variability and low contrast. The Minimum Boundary Contrast Detection (MBCD) technique is presented to determine the pixel distribution patterns across a picture's x and y axes. In recurrent learning, textures, and bounds are confirmed in n*n regions across the x and y axes after being derived from the pixels. As texture-based boundary changes are discovered, the feature extraction algorithm adjusts accordingly. Therefore, within the established limits and their min-max variations, learning occurs iteratively until the greatest precision in object detection is obtained via boundary detection. Object detection is halted, and complexity and time needs are reduced if the boundary closure arises within any part of the distribution. Features in texture and pixels at the distribution's start and end are used to determine where the boundary occurs. The deep recurrent learning uses the lowest and maximum differences in texture to determine the variations. This variation identification is repeated until a saturating or least resolution is found. Object detection may be limited to a small, specified region with the help of region segregation. As a result, this study examines the region where distribution stopped in more detail, searching for combinations of comparable pixels from the training set. The proposed technique successfully overcomes the difficulty of detecting camouflaged objects by applying boundary detection and leveraging the characteristics of low contrast and high variance. It reduces complexity by 12.49 % and saves time by 11.52 % using a recurrent learning method that accounts for shifting boundaries. The process detects the camouflage with high accuracy by 9.17 %.
更多查看译文
关键词
Boundary Identification,Camouflage,Object Detection,Recurrent Learning,Pixel Distribution,Large-Scale Images
AI 理解论文
溯源树
样例
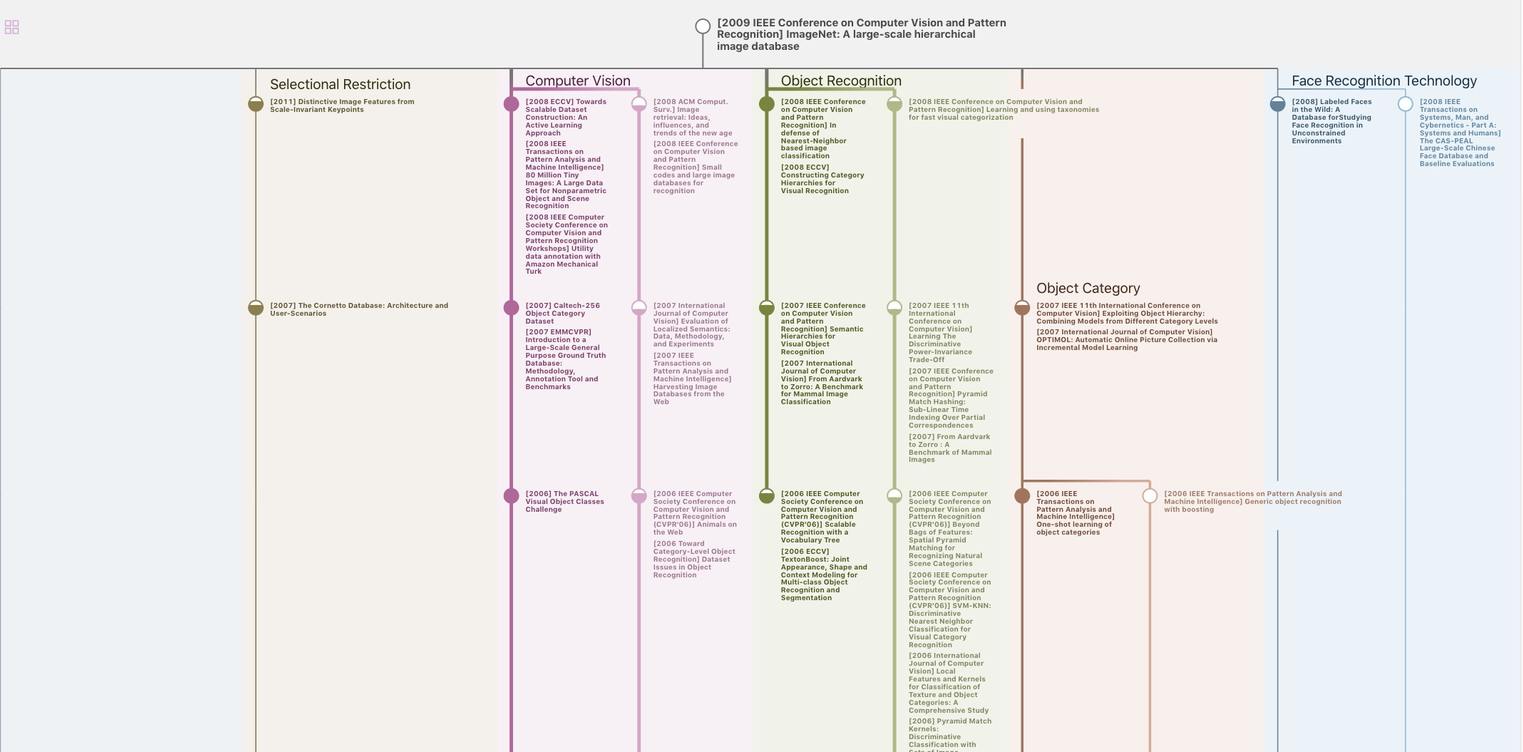
生成溯源树,研究论文发展脉络
Chat Paper
正在生成论文摘要