Harnessing Historic Records and Long-Term Monitoring Data to Evaluate Amphibian Extinction Dynamics
Biological Conservation(2024)
Univ Melbourne | Dept Planning & Environm | Arthur Rylah Inst Environm Res | Dept Energy Environm & Climate Act
Abstract
Understanding the nature and extent of global amphibian declines has been hampered because pre-decline data rarely exist, post-decline data can be incomplete and amphibian population fluctuations can be influenced by multiple factors. Importantly, data deficiencies have impeded assessments of the roles of the pathogen, chytrid fungus Batrachochytrium dendrobatidis, and co-occurring host or competitor species in amphibian population declines. We used dynamic occupancy models to examine the influence of chytrid upon two sympatric frog species and their interactions. Models combined sporadic historic data and more intensive post-decline data collected over a 55 -year period (1958-2012) for the threatened Spotted Tree Frog Litoria spenceri and nonthreatened Lesueur's Frog L. lesueurii. Our analysis indicated an approximate 50 % decline in L. spenceri site occupancy. This decline was most severe at sites when both chytrid and L. lesueurii were present. Further L. spenceri declines are predicted without intervention. In contrast, L. lesueurii tended to become more prevalent over time, and changes in L. lesueurii occurrence over time were not associated with chytrid or L. spenceri occurrence. Efforts to conserve L. spenceri when threatened by chytrid may be most effective at sites where L. lesueurii (a potential pathogen reservoir) is absent. Our work highlights the risk posed by sympatric pathogen reservoir species for susceptible species. This study not only demonstrates the value of long -term population monitoring, but how incorporation of sporadic, historic records may provide valuable insights into processes underlaying species decline and recovery.
MoreTranslated text
Key words
Dynamic occupancy model,Pathogen host reservoir,Long-term monitoring,Amphibian,Novel pathogen,PVA,Chytridiomycosis
求助PDF
上传PDF
View via Publisher
AI Read Science
AI Summary
AI Summary is the key point extracted automatically understanding the full text of the paper, including the background, methods, results, conclusions, icons and other key content, so that you can get the outline of the paper at a glance.
Example
Background
Key content
Introduction
Methods
Results
Related work
Fund
Key content
- Pretraining has recently greatly promoted the development of natural language processing (NLP)
- We show that M6 outperforms the baselines in multimodal downstream tasks, and the large M6 with 10 parameters can reach a better performance
- We propose a method called M6 that is able to process information of multiple modalities and perform both single-modal and cross-modal understanding and generation
- The model is scaled to large model with 10 billion parameters with sophisticated deployment, and the 10 -parameter M6-large is the largest pretrained model in Chinese
- Experimental results show that our proposed M6 outperforms the baseline in a number of downstream tasks concerning both single modality and multiple modalities We will continue the pretraining of extremely large models by increasing data to explore the limit of its performance
Upload PDF to Generate Summary
Must-Reading Tree
Example
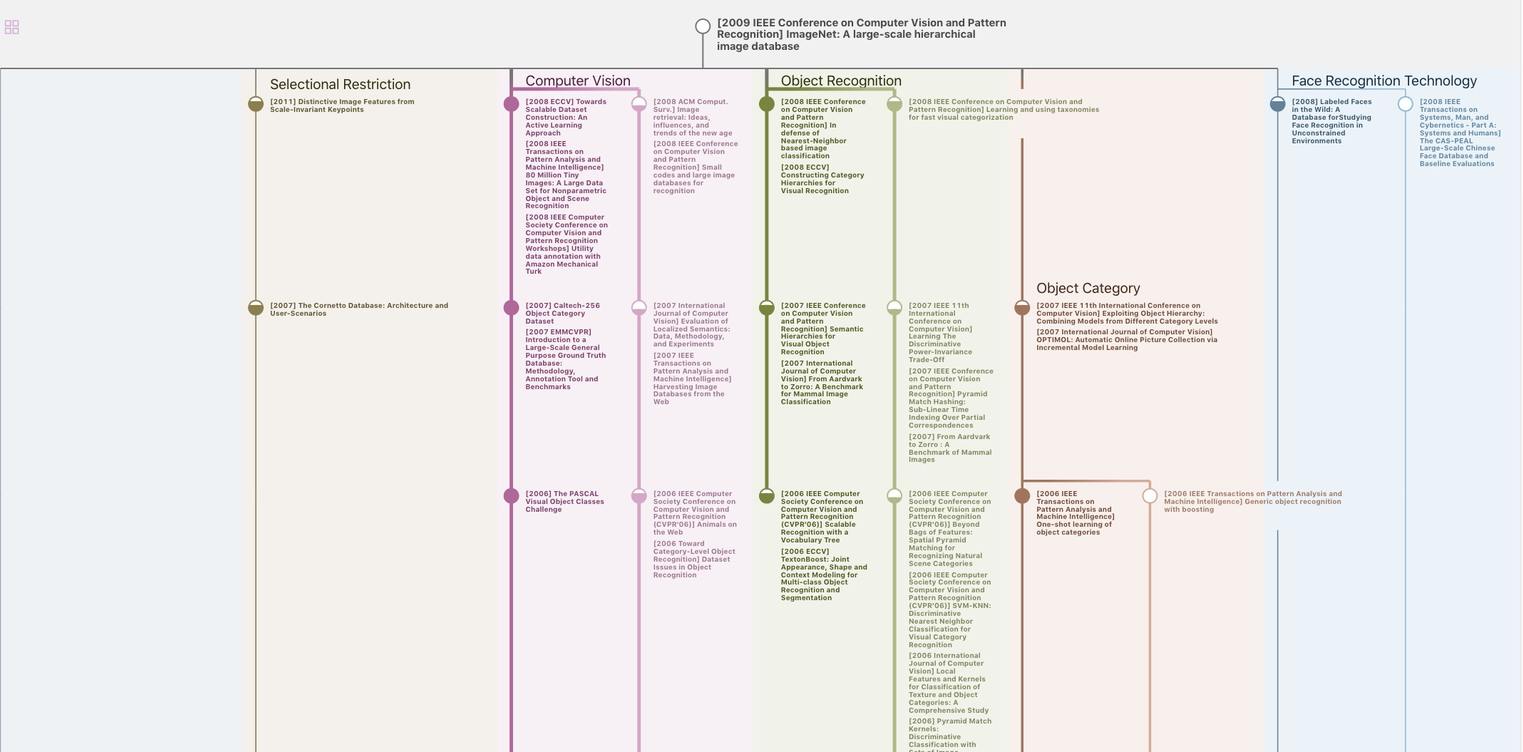
Generate MRT to find the research sequence of this paper
Related Papers
2005
被引用79 | 浏览
1994
被引用1102 | 浏览
1998
被引用2816 | 浏览
ESTIMATING SITE OCCUPANCY, COLONIZATION, AND LOCAL EXTINCTION WHEN A SPECIES IS DETECTED IMPERFECTLY
2003
被引用1475 | 浏览
2001
被引用103 | 浏览
2008
被引用1483 | 浏览
2006
被引用1480 | 浏览
2011
被引用256 | 浏览
2007
被引用719 | 浏览
2013
被引用101 | 浏览
2014
被引用155 | 浏览
2014
被引用26 | 浏览
2018
被引用49 | 浏览
Data Disclaimer
The page data are from open Internet sources, cooperative publishers and automatic analysis results through AI technology. We do not make any commitments and guarantees for the validity, accuracy, correctness, reliability, completeness and timeliness of the page data. If you have any questions, please contact us by email: report@aminer.cn
Chat Paper