Tool Wear State Recognition and Prediction Method Based on Laplacian Eigenmap with Ensemble Learning Model
Advanced engineering informatics(2024)
摘要
Accurate prediction of tool wear status plays a critical role in the digital manufacturing industry, and its health level directly affects machining quality, production costs, and overall productivity. In response to the problems of the high dimensionality of extracted features from tool wear characterization sensors, redundant information, and large individual model errors and biases, a novel method for tool wear status identification and prediction that fuses downscaling dimensionality and ensemble models is proposed. First, a multi-algorithm feature filtering based on Random Forest (RF) and extreme gradient boosting (XGBoost) is utilized, and the laplacian eigenmaps (LE) algorithm is combined to perform fusion downscaling on the filtered features. Then, the parameters of the XGBoost algorithm are optimized using grid search (GS). Finally, the performance of the proposed method is evaluated by different tool wear experiments for both regression and classification using model prediction accuracy evaluation metrics (R-squared values and f1 values) and prediction time. The experimental results show that for different tools wear experimental data, the R-squared values of the regression model are higher than 0.98, the f1 values of the classification model are above 0.96, and the prediction speed is improved by an order of magnitude compared with other models. The results are analyzed to verify the effectiveness and applicability of the proposed method, which can provide technical support for the automated machining process.
更多查看译文
关键词
Tool wear,Laplacian eigenmaps,Features fusion,XGBoost algorithm,Ensemble method
AI 理解论文
溯源树
样例
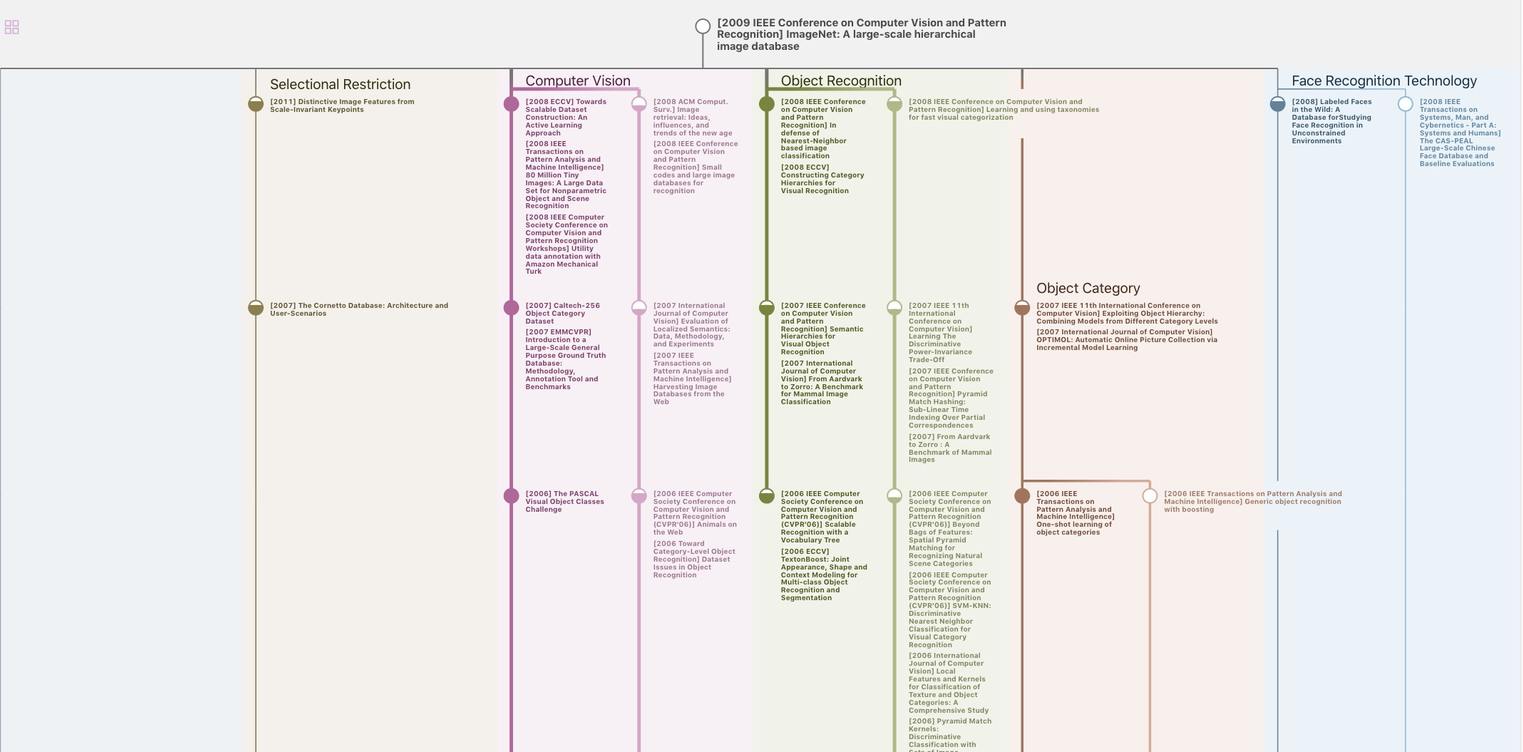
生成溯源树,研究论文发展脉络
Chat Paper
正在生成论文摘要