MamlFormer: Priori-experience Guiding Transformer Network Via Manifold Adversarial Multi-modal Learning for Laryngeal Histopathological Grading
Information fusion(2024)
摘要
Pathologic grading of laryngeal squamous cell carcinoma (LSCC) plays a crucial role in diagnosis, prognosis, and migration. However, the grading performance and interpretability of the intelligent grading model based on LSCC low magnification images are poor. This is because it lacks the delicate nuclear information and information more relevant to grading contained in the high magnification images labeled by pathologists. Yet, low magnification images have information such as tissue texture and contours. Thus, we proposed an end-to-end transformer network with manifold adversarial multi-modal learning (MamlFormer). It effectively fuses and learns LSCC high and low magnification pathology image modalities. Firstly, we demonstrate the feasibility and sufficient conditions for modal fusion of LSCC high and low magnification images from Hoeffding's inequality and multimodal co-regularization. Secondly, we design a new manifold block. It constructs the manifold subspace by some principles. Those principles are divisibility, recoverability, and local distance closest of the feature matrix before and after the mapping of the LSCC each magnification image modalities. Meanwhile it can well solve the problems of redundant feature matrix information and weak modal semantic consistency after multimodal learning. Thirdly, we utilize the encoder and the adversarial loss function to implement adversarial block. It can adaptively learn the latent metrics of the modal distributions of LSCC high and low magnification images. Therefore, it also enhances the complementarity of LSCC high and low magnification image modalities. Then, numerous experiments show that MamlFormer outperforms other SOTA models in both grading performance and interpretability. Finally, we also performed generalization experiments on highly prevalent cervix squamous cell carcinoma. The MamlFormer over is superior to other SOTA models in terms of grading performance and interpretability. This indicates its excellent generalization performance and clinical practicability.
更多查看译文
关键词
Larynx cancer,Pathological grading,Histopathological images,Multi-modal,Magnification fusion,Transformer
AI 理解论文
溯源树
样例
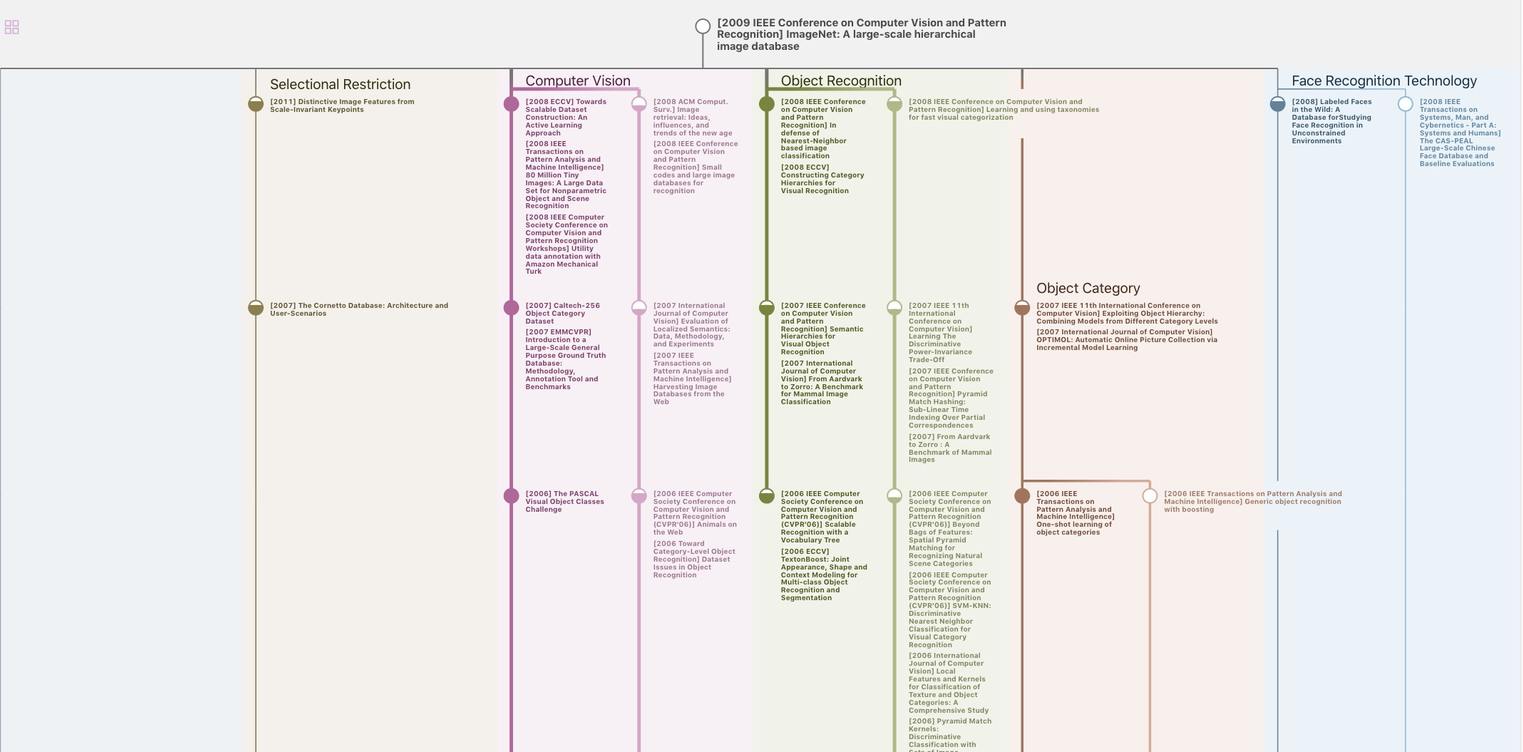
生成溯源树,研究论文发展脉络
Chat Paper
正在生成论文摘要