Machine Learning Aided Investigation on the Structure-Performance Correlation of MOF for Membrane-Based He/H2 Separation
Green Chemical Engineering(2024)
摘要
The separation of He/H2 using membrane technology has gained significant interest in the field of He extraction from natural gas. One of the greatest challenges associated with this process is the extremely close kinetic diameters of the two gas molecules, resulting in low membrane selectivity. In this study, we investigated the structure-performance relationship of metal-organic framework (MOF) membranes for He/H2 separation through molecular simulations and machine learning approaches. By conducting molecular simulations, we identified the potential MOF membranes with high separation performance from the Computation-Ready Experimental (CoRE) MOF database, and the diffusion-dominated mechanism was further elucidated. Moreover, random forest (RF)-based machine learning models were established to identify the crucial factors influencing the He/H2 separation performance of MOF membranes. The pore limiting diameter (PLD) and void fraction (φ), are revealed as the most important physical features for determining the membrane selectivity and He permeability, respectively. Additionally, density functional theory (DFT) calculations were carried out to validate the molecular simulation results and suggested that the electronegative atoms on the pore surfaces can enhance the diffusion-based separation of He/H2, which is critical for improving the membrane selectivities of He/H2. This study offers useful insights for designing and developing novel MOF membranes for the separation of He/H2 at the molecular level.
更多查看译文
关键词
He/H2,Membrane separation,Molecular simulation,Machine learning,DFT calculation
AI 理解论文
溯源树
样例
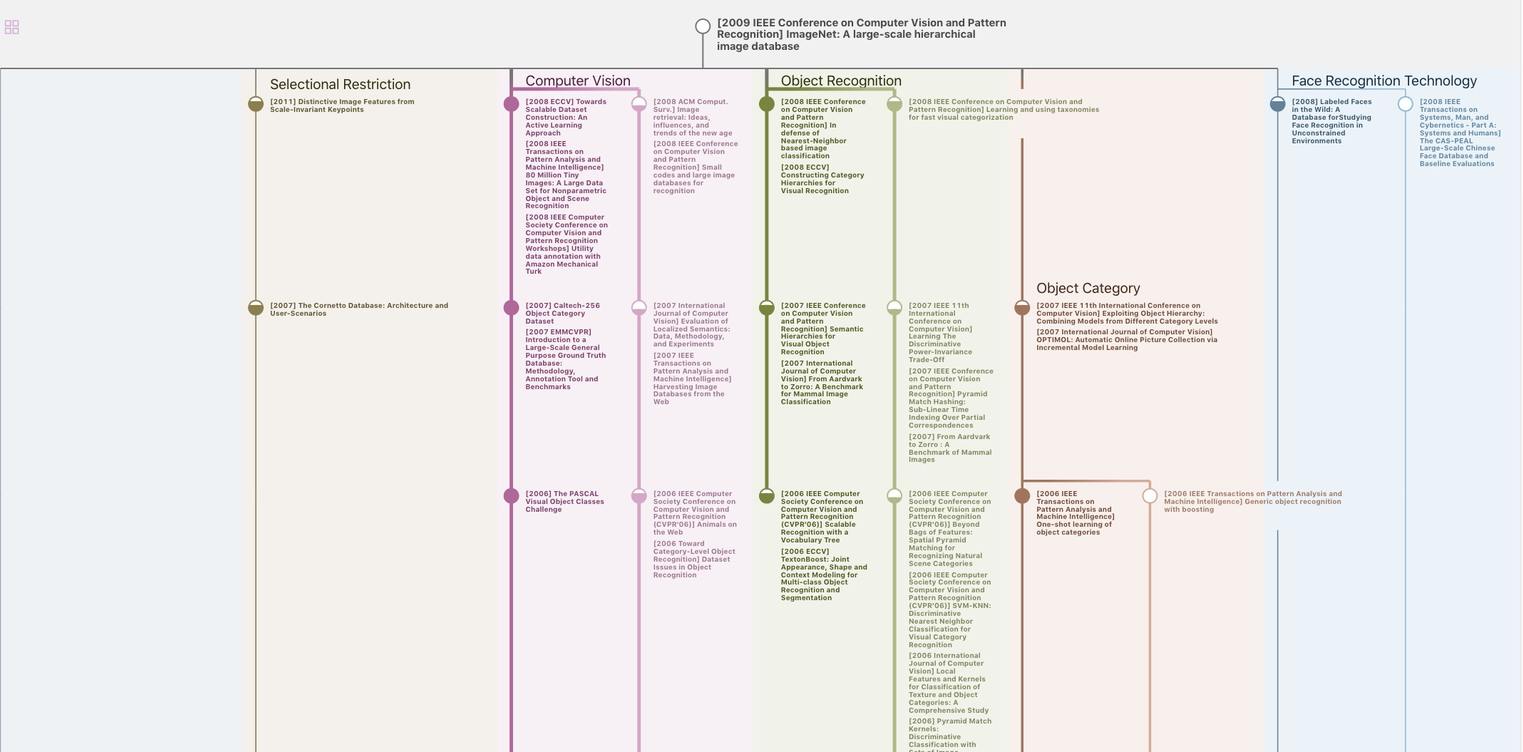
生成溯源树,研究论文发展脉络
Chat Paper
正在生成论文摘要