Conscious Perception of Fear in Faces: Insights from High-Density EEG and Perceptual Awareness Scale with Threshold Stimuli
Cortex(2024)
摘要
Contrary to the extensive research on processing subliminal and/or unattended emotional facial expressions, only a minority of studies have investigated the neural correlates of consciousness (NCCs) of emotions conveyed by faces. In the present high-density electroencephalography (EEG) study, we first employed a staircase procedure to identify each participant's perceptual threshold of the emotion expressed by the face and then compared the EEG signals elicited in trials where the participants were aware with the activity elicited in trials where participants were unaware of the emotions expressed by these, otherwise identical, faces. Drawing on existing knowledge of the neural mechanisms of face processing and NCCs, we hypothesized that activity in frontal electrodes would be modulated in relation to participants' awareness of facial emotional content. More specifically, we hypothesized that the NCC of fear seen on someone else's face could be detected as a modulation of a later and more anterior (i.e., at frontal sites) event-related potential (ERP) than the face-sensitive N170. By adopting a data-driven approach and cluster-based statistics to the analysis of EEG signals, the results were clear-cut in showing that visual awareness of fear was associated with the modulation of a frontal ERP component in a 150 -300 msec interval. These insights are dissected and contextualized in relation to prevailing theories of visual consciousness and their proposed NCC benchmarks. (c) 2024 The Author(s). Published by Elsevier Ltd. This is an open access article under the CC BY -NC -ND license (http://creativecommons.org/licenses/by-nc-nd/4.0/).
更多查看译文
关键词
Faces,Facial expressions,Fear,Neural correlates of consciousness,Event-related potentials,Electroencephalography
AI 理解论文
溯源树
样例
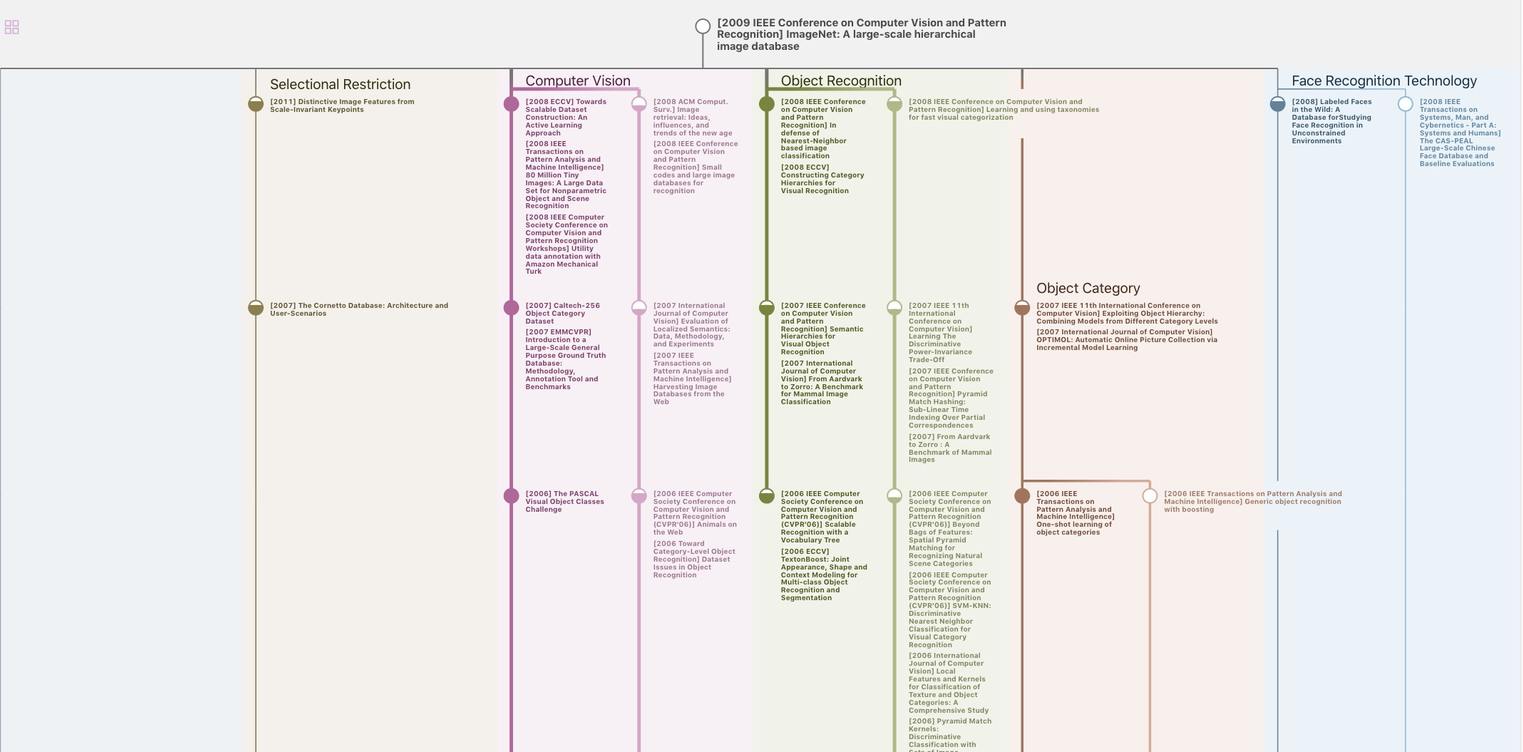
生成溯源树,研究论文发展脉络
Chat Paper
正在生成论文摘要