Designing an Implementable Clinical Prediction Model for Near-Term Mortality and Long-Term Survival in Patients on Maintenance Hemodialysis
AMERICAN JOURNAL OF KIDNEY DISEASES(2024)
摘要
Rationale & ObjectiveLife expectancy of patients treated with maintenance hemodialysis (MHD) is heterogeneous. Knowledge of life-expectancy may focus care decisions on near-term vs. long-term goals. Current tools are limited and focus on near-term mortality. Here, we develop and assess potential utility for predicting near-term mortality and long-term survival on MHD.Study DesignPredictive modelling study.Setting & Participants42,351 patients contributing 997,381 patient months over 11 years, abstracted from the EHR system of mid-size, non-profit dialysis providers.New Predictors & Established PredictorsDemographics, laboratory results, vital signs, and service utilization data available within dialysis EHR.OutcomesFor each patient month, we ascertained death within the next 6-months (i.e., near-term mortality) and survival over more than 5-years during receipt of MHD or following kidney transplantation (i.e., long-term survival).Analytical ApproachWe used LASSO logistic regression and gradient-boosting machines to predict each outcome. We compared these to time-to-event models spanning both time horizons. We explored the performance of decision rules at different cut-points.ResultsAll models achieved AUROC ≥ 0.80 and optimal calibration metrics in the test set. Long-term survival models had significantly better performance than near-term mortality models. Time-to-event models performed similarly to binary models. Applying different cutpoints spanning from the 1st to 90th percentile of the predictions, a positive predictive value (PPV) of 54% could be achieved for near-term mortality, but with poor sensitivity of 6%. A PPV of 71% could be achieved for long-term survival with a sensitivity of 67%.LimitationsThe retrospective models would need to be prospectively validated before they could be appropriately used as clinical decision aids.ConclusionsA model built with readily available clinical variables to support easy implementation, can predict clinically important life expectancy thresholds and shows promise as a clinical decision support tool for patients on MHD. Predicting long-term survival has better decision rule performance than predicting near-term mortality.
更多查看译文
关键词
Clinical Prediction,Dialysis,Mortality,Risk Prediction,Machine Learning
AI 理解论文
溯源树
样例
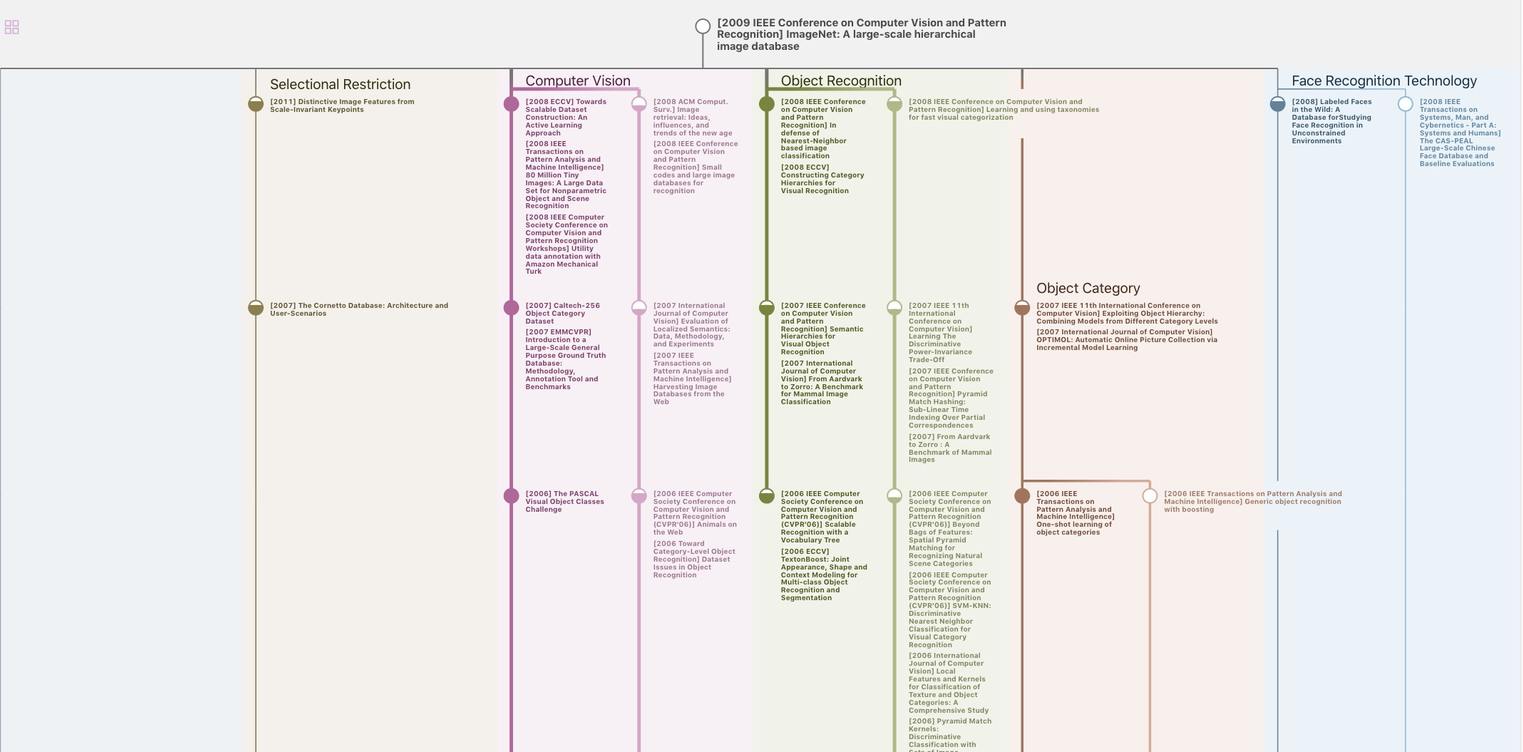
生成溯源树,研究论文发展脉络
Chat Paper
正在生成论文摘要