MetaCluster: A Universal Interpretable Classification Framework for Cybersecurity.
IEEE Trans. Inf. Forensics Secur.(2024)
摘要
Rising cyber threats have created an immediate demand for Deep Learning (DL) in cybersecurity. Nevertheless, the opaque nature of DL models poses challenges in deploying, collaborating, and assessing their effectiveness in less reliable cybersecurity environments. Despite eXplainable Artificial Intelligence (XAI) playing a role in enhancing cybersecurity analytics, the limited task scope, the propensity for data overfitting, and the stochastic explanations hinder its broader application. To fill the gap, this paper introduces a generic interpretable classification framework, named MetaCluster. MetaCluster generates semantic prototypes for features, patterns, and domains at varying granular levels by following three fundamental steps: embedding representations, acquiring prototypes, and aggregating semantics. These mechanisms guarantee that MetaCluster achieves critical information extraction and reliable classification at minimal cost. The experiments encompass cybersecurity classification tasks and assess the interpretability of the framework. These tasks encompass malware family classification, threat behavior analysis, and malicious traffic identification. In particular, when compared to other DL models, MetaCluster exhibits a significant reduction in parameter consumption by 79.52% to 91.78%, and boosts operational speed up to 71.37%, while its F1 scores remain stable or slightly increase. Additionally, MetaCluster possesses the ability to assess and visually represent the significance of image, text, and statistical features. This capability leads to a reduction of Mean Squared Error (MSE) between expected and actual predictions by 0.0101 to 0.1020.
更多查看译文
关键词
eXplainable Artificial Intelligence (XAI),Cybersecurity,Interpretable Classification,Model Lightweight,General Framework
AI 理解论文
溯源树
样例
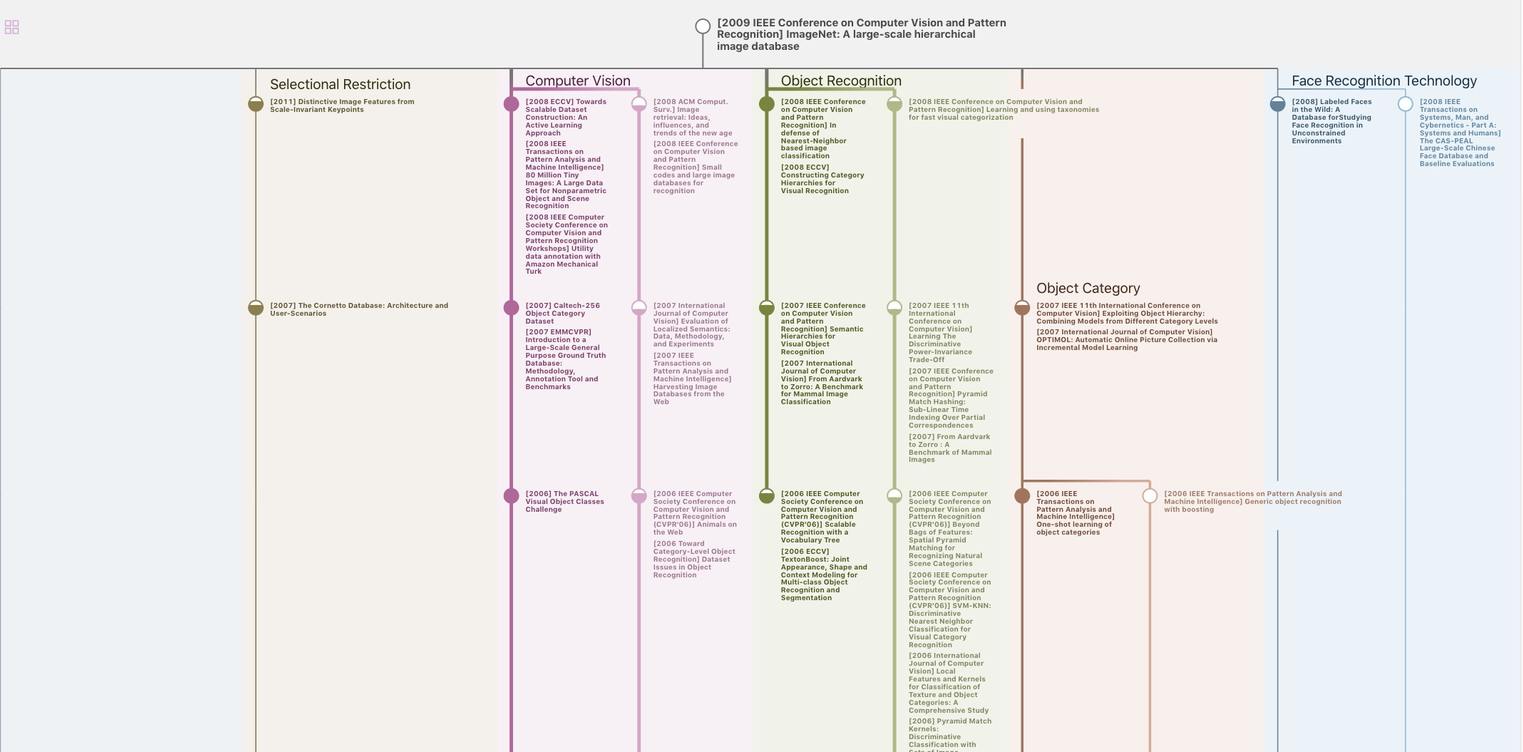
生成溯源树,研究论文发展脉络
Chat Paper
正在生成论文摘要